Using machine learning to guide targeted and locally-tailored empiric antibiotic prescribing in a children's hospital in Cambodia
Posted on: 22 July 2018 , updated on: 29 September 2019
Preprint posted on 13 July 2018
What the AI doctor ordered: Applying Machine Learning methods to combat antimicrobial resistance
Selected by Zhang-He GohCategories: epidemiology
Background of preprint
Considered by WHO to be “one of the most serious threats to global health, food security and development” [1], the rising global rates of antimicrobial resistance in the past decade has led to increasing efforts to preserve the diminishing arsenal of antimicrobials by healthcare professionals worldwide. To this end, antimicrobial stewardship programmes have been set up in many countries to reduce the unnecessary use of precious antimicrobials in patients. The use of antimicrobials in patients has two categories of negative consequences. The first category pertains to the patient directly in the form of adverse effects arising from the use of antimicrobials. The second category affects the broader community, as resistance rates in healthcare institutions generally rise over time following repeated use of the same antibiotic regimens, a phenomenon known as the ecological effect. Therefore, there is a pressing need for inexpensive and easily implemented methods to optimise antimicrobial use. But accurately predicting which patients would benefit from a course of antibiotics presents a challenge. Therefore, this 2018 study by Oonsivilai et al explores the potential of utilising Machine Learning strategies to reduce antibiotic use by helping physicians decide when to prescribe or withhold antibiotics.
Key findings of preprint
Oonsivilai et al used retrospective secondary data from a children’s hospital in Cambodia in training and testing three main algorithms used in Machine Learning against a logistic regression as follows.
- Decision Trees (DTs) with recursive partitioning, random forest, and adaptive boosts
- Support Vector Machines (SVMs) with linear, polynomial, and radial kernels
- k-nearest neighbours
These algorithms were compared on their ability to correctly predict the causative organisms’ susceptibility to two different antibiotic regimens: (i) ampicillin and gentamicin, or (ii) ceftriaxone. Among the 243 cases that this study looked at, 80% of the data was used for training the algorithms and 20% was used for testing. From their analysis, the authors found the random forest algorithm to be the most optimal, with a sensitivity of 80% and a specificity of 20%.
The second finding of this preprint involves the identification of four important predictors of this outcome:
- Days from hospital admission to blood sample
- Patient’s age
- Classification of the infection: Whether it was hospital- or community-acquired
- Patient’s age-adjusted weight
All these predictors ranked highly in the random forests model in predicting whether pathogens were susceptible to ceftriaxone. These results suggest that using Machine Learning strategies could potentially be used as an adjunct to existing clinical guidelines in promoting the judicious use of antibiotics in healthcare institutions.
What I like about this preprint
Oonsivilai et al adopted a different algorithm from previous machine learning approaches in the literature. Most studies use SVMs, which are mysterious “black-boxes” that do not reveal the logic behind their predictions [2]. In contrast, four clinically significant predictors were identified in this preprint. First, it is widely accepted among healthcare professionals that obtaining a culture is one of the most important procedures upon admission of patients suspected of suffering from infections. Patients’ ages and weights, too, are known to be clinically important factors that affect prognosis. Finally, published clinical guidelines also rely on the accurate classification of the infection as hospital- or community-acquired in the recommendation of an antibiotic regimen.
A second aspect of this preprint that interested me was the quantification of utility in antibiotic resistance. Oonsivilai et al considered the significance of utility in both over- and under-treatment, including the actual financial costs involved in such decision making. Rather than consider just the sensitivity and specificity of their model, the researchers also assigned a different cost to false positives and false negatives identified by the model. This is vital because wrongly classifying a non-susceptible infection as susceptible (false positive) is likely to have more serious consequences than wrongly classifying a susceptible infection as non-susceptible (false negative)—the former may lead to sepsis, a severe and life-threatening condition. Therefore, this additional feature both makes for a more complete analysis and provides support for the authors’ discussion as well.
Future directions
This preprint generates many hypotheses which could be further tested in future studies. As Oonsivilai et al point out, antibiotic susceptibility rates differ across local settings. Still, this preprint lays out the key principles that are useful in informing different research questions and hypotheses posed by future larger studies. By virtue of their larger size and greater statistical power, bigger datasets would allow researchers to look at even more features and explore more predictive relationships while decreasing the chance of overfitting.
Questions for the authors
- Why was susceptibility to ampicillin/gentamicin and ceftriaxone chosen as the outcome? Was susceptibility to other antibiotic regimens considered?
- Given that the setting for this preprint is in a children’s hospital in Cambodia, would you expect a similar level of complexity for an analysis of the dataset from a hospital which also cares for adult patients?
- Were there any inclusion and exclusion criteria that used in this study in forming the dataset, or were all patients extracted from the database used for this study regardless of disease conditions?
References
[1] Destoumieux-Garzón D, Mavingui P, Boetsch G, Boissier J, Darriet F, Duboz P, Fritsch C, Giraudoux P, Le Roux F and Morand S (2018) The one health concept: 10 years old and a long road ahead. Frontiers in veterinary science 5: 14.
[2] Arango-Argoty G, Garner E, Pruden A, Heath LS, Vikesland P and Zhang L (2018) DeepARG: a deep learning approach for predicting antibiotic resistance genes from metagenomic data. Microbiome 6(1): 23.
Read preprintSign up to customise the site to your preferences and to receive alerts
Register hereAlso in the epidemiology category:
Multimodal interactions in Stomoxys navigation reveals synergy between olfaction and vision
Maitri Manjunath

Mapping current and future thermal limits to suitability for malaria transmission by the invasive mosquito Anopheles stephensi
Sophia Friesen
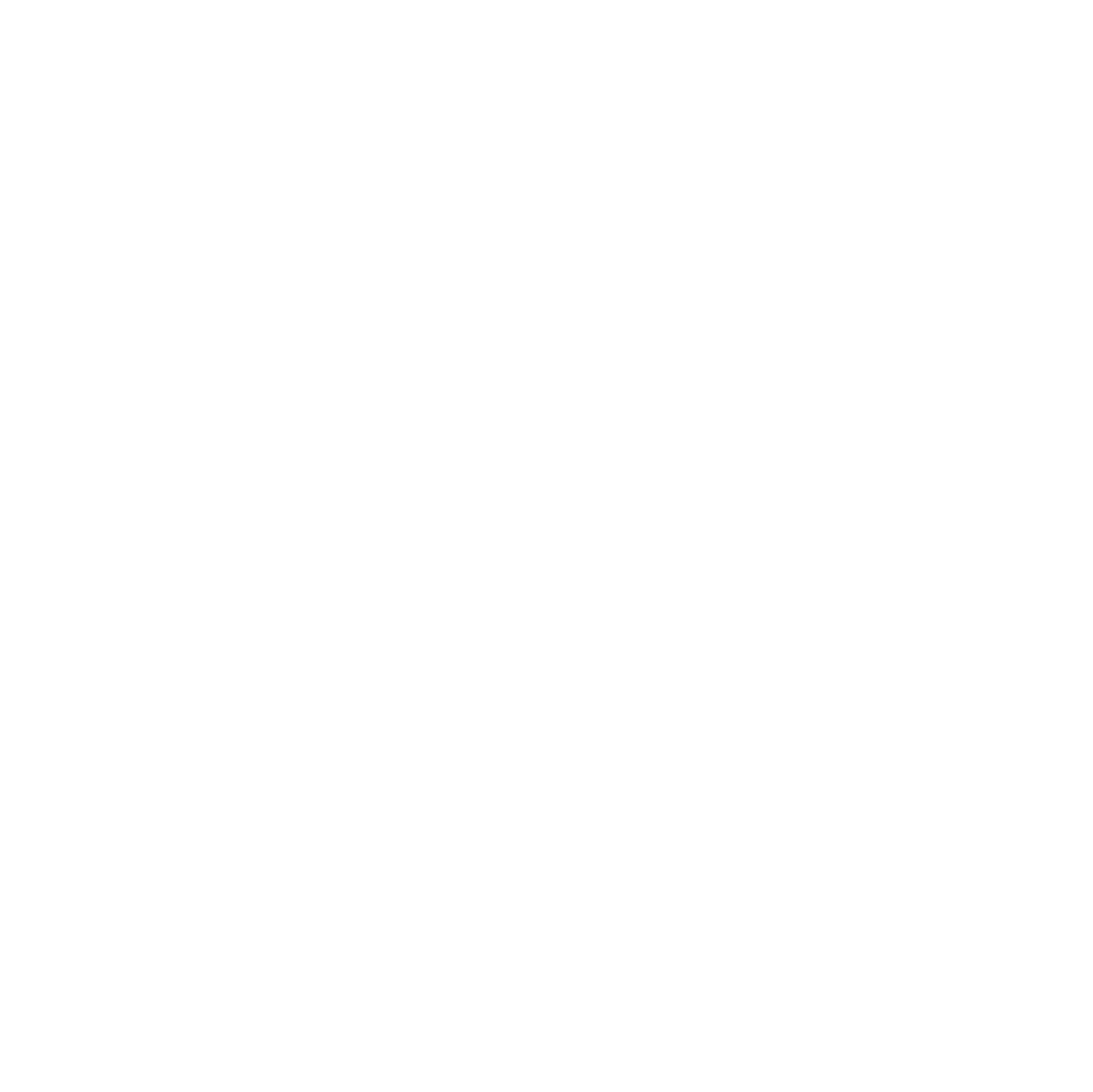
Isolation disrupts social interactions and destabilizes brain development in bumblebees
Niveda Udaykumar

preListsepidemiology category:
in theBioMalPar XVI: Biology and Pathology of the Malaria Parasite
[under construction] Preprints presented at the (fully virtual) EMBL BioMalPar XVI, 17-18 May 2020 #emblmalaria
List by | Dey Lab, Samantha Seah |
1
COVID-19 / SARS-CoV-2 preprints
List of important preprints dealing with the ongoing coronavirus outbreak. See http://covidpreprints.com for additional resources and timeline, and https://connect.biorxiv.org/relate/content/181 for full list of bioRxiv and medRxiv preprints on this topic
List by | Dey Lab, Zhang-He Goh |
1
Antimicrobials: Discovery, clinical use, and development of resistance
Preprints that describe the discovery of new antimicrobials and any improvements made regarding their clinical use. Includes preprints that detail the factors affecting antimicrobial selection and the development of antimicrobial resistance.
List by | Zhang-He Goh |