Drug mechanism-of-action discovery through the integration of pharmacological and CRISPR screens
Posted on: 20 March 2020 , updated on: 23 March 2020
Preprint posted on 14 January 2020
Article now published in Molecular Systems Biology at http://dx.doi.org/10.15252/msb.20199405
Genome-wide CRISPR screens and drug screens come together to reveal cancer drug mechanism-of-action.
Selected by Georgina SavaCategories: bioinformatics, cancer biology
BACKGROUND
Drug development has a notoriously high failure rate, with less than 10% of candidate compounds entering Phase I clinical trials1. Understanding drug mechanism-of-action can provide information about specificity and potency, and off-target activity which may contribute to toxicity. It can also help identify biomarkers of activity and response, allowing the drug to be trialled in those patients who are most likely to derive benefit. This knowledge can increase the likelihood of a drug eventually achieving clinical success.
In vitro kinase assays are commonly used to assess the specificity and potency of protein kinase inhibitors, however, their in vitro nature means protein-drug interactions are not assessed in their native cellular context. Furthermore, a whole host of non-kinase proteins are present in cells, which are not profiled for off-target interactions in these assays. Other approaches to investigate drug mechanism-of-action include transcriptional profiling following drug treatment and kinobead assays. Despite the utility of these approaches, gaining a full picture of drug mechanism-of-action in cells remains challenging.
Pharmacological screens, in which the activities of hundreds of anti-cancer compounds are profiled on large panels of cancer cell lines, have been used to identify molecular features associated with drug sensitivity. More recently, the advent of CRISPR-based gene editing has allowed these cell lines to be screened genome-wide for gene loss-of-function effects on a genome-wide scale, to explore cancer vulnerabilities and identify new drug targets.
Here, Gonçalves et al. have integrated pharmacological data, for 397 anti-cancer compounds screened in 484 cancer cell lines2-4, with two genome-wide CRISPR-Cas9 knockout screens from the same cell lines5,6. Combining these two powerful datasets provides insights into drug potency and selectivity, underlying cellular networks influencing drug sensitivity, and provides a framework for using these types of data to guide drug development.
KEY FINDINGS
The authors began by using linear regression to systematically search for associations between drug sensitivity and gene fitness effects (a measure of cell line dependency on a specific gene), testing almost 8 million potential drug-gene associations! 865 significant drug-gene pairs were identified. One might expect that a cell line that is especially sensitive to an anti-cancer drug will also be particularly sensitive to knockout of the drug target. There were 358 drugs for which the known target was also knocked-out in the CRISPR screens. Of these, 26% were, in fact, significantly associated with their target, for example cell line response to the BCL2 inhibitor venetoclax was strongly correlated with the effect of BCL2 knockout.
To further investigate the remaining drugs, significant drug-gene pairs were superimposed onto a protein-protein interaction (PPI) network – perhaps these drugs are associated with genes in the same PPI network as their target? Indeed, a further 76 drugs had a significant association, not with their target, but with a protein closely related in the network. This still leaves 167 drugs (46.6%) with no significant gene association. There are many possible explanations for this, including the fact that the effects of gene knockouts can be intrinsically different to those of protein inhibition. Nevertheless, it may be worthwhile investigating these compounds for low potency, alternative molecular mechanisms, or polypharmacology.

Next, the strongest drug-gene associations were examined. Remarkably, this method was able to distinguish between isoform-selective PI3K inhibitors. For example, the PI3K-alpha selective inhibitor alpelisib was associated with PIK3CA, but no other PI3K isoform genes. Seven MCL1 inhibitors were among the strongest drug-gene pair associations. MCL1 represses the mitochondrial apoptotic pathway by regulating proteins which control mitochondrial fragmentation and cytochrome c release. Interestingly, these were all strongly associated with both MCL1 and with another gene, MARCH5, a key regulator of mitochondrial fission. Cell lines that are dependent on both MCL1 and MARCH5 are especially sensitive to MCL1 inhibition. This highlights a functional link between MARCH5 and MCL1 inhibitor sensitivity, which with further investigation, may help guide clinical development of MCL1 inhibitors in certain cancer types.
Lastly, the authors hypothesise that molecular features associated with both drug response and target gene fitness represent robust pharmacogenomic biomarkers. They were able to identify well established biomarkers of drug response, for example Nutlin-3a sensitivity and p53 status, and olaparib sensitivity and EWSR-FLI1 fusion status.
WHY I CHOSE THIS PREPRINT
I am currently investigating response and resistance mechanisms to a new targeted therapy for cancer, so I am always interested in reading about novel ways to investigate this. Recently a huge amount of pharmacological and genome-wide CRISPR screen data has been made publicly available, providing an invaluable resource for the scientific community, and there is likely much more to come! I have been eager to see how these screens might start to be used to inform drug discovery.
FUTURE DIRECTIONS
Recently, the CRISPR-Cas9 gene-editing system has been adapted so that genes can be specifically activated (CRISPRa) or repressed (CRISPRi)7. Integration of future genome-wide CRISPRa or CRISPRi screens with this pharmacological data may help identify drug-gene associations for the 167 drugs for which no associations were found, particularly as CRISPRi may resemble incomplete target inhibition by a compound more closely than gene knockout.
One aim of this work is to identify off-target effects of cancer drugs. It may be useful to perform similar screens on panels of non-cancer cell lines, which may uncover cell-type specific drug-gene associations which contribute to some of the side-effects of cancer drugs.
A really exciting element of this work is the suggestion that in the future it may be possible to screen drug candidate compounds in this way to help prioritise those for further development. Perhaps something like this may even become a routine step in the drug discovery pipeline.
QUESTIONS FOR AUTHORS
- Were any drug-gene associations found for drugs for which a target is currently unknown? Could this approach be used to help identify targets for those?
- Do you plan to further investigate/confirm the role of MARCH5 in MCL1 inhibitor response, and how might you go about this?
REFERENCES
1 Dowden, H. & Munro, J. Trends in clinical success rates and therapeutic focus. Nat Rev Drug Discov 18, 495-496, doi:10.1038/d41573-019-00074-z (2019).
2 Garnett, M. J. et al. Systematic identification of genomic markers of drug sensitivity in cancer cells. Nature 483, 570-575, doi:10.1038/nature11005 (2012).
3 Iorio, F. et al. A Landscape of Pharmacogenomic Interactions in Cancer. Cell 166, 740-754, doi:10.1016/j.cell.2016.06.017 (2016).
4 Yang, W. et al. Genomics of Drug Sensitivity in Cancer (GDSC): a resource for therapeutic biomarker discovery in cancer cells. Nucleic Acids Research 41, D955-D961, doi:10.1093/nar/gks1111 (2012).
5 Meyers, R. M. et al. Computational correction of copy number effect improves specificity of CRISPR-Cas9 essentiality screens in cancer cells. Nat Genet 49, 1779-1784, doi:10.1038/ng.3984 (2017).
6 Behan, F. M. et al. Prioritization of cancer therapeutic targets using CRISPR-Cas9 screens. Nature 568, 511-516, doi:10.1038/s41586-019-1103-9 (2019).
7 Fellmann, C., Gowen, B. G., Lin, P.-C., Doudna, J. A. & Corn, J. E. Cornerstones of CRISPR-Cas in drug discovery and therapy. Nature reviews. Drug discovery 16, 89-100, doi:10.1038/nrd.2016.238 (2017).
doi: https://doi.org/10.1242/prelights.17722
Read preprintSign up to customise the site to your preferences and to receive alerts
Register hereAlso in the bioinformatics category:
Enhancer-driven cell type comparison reveals similarities between the mammalian and bird pallium
Rodrigo Senovilla-Ganzo

Expressive modeling and fast simulation for dynamic compartments
Benjamin Dominik Maier

Transcriptional profiling of human brain cortex identifies novel lncRNA-mediated networks dysregulated in amyotrophic lateral sclerosis
Julio Molina Pineda
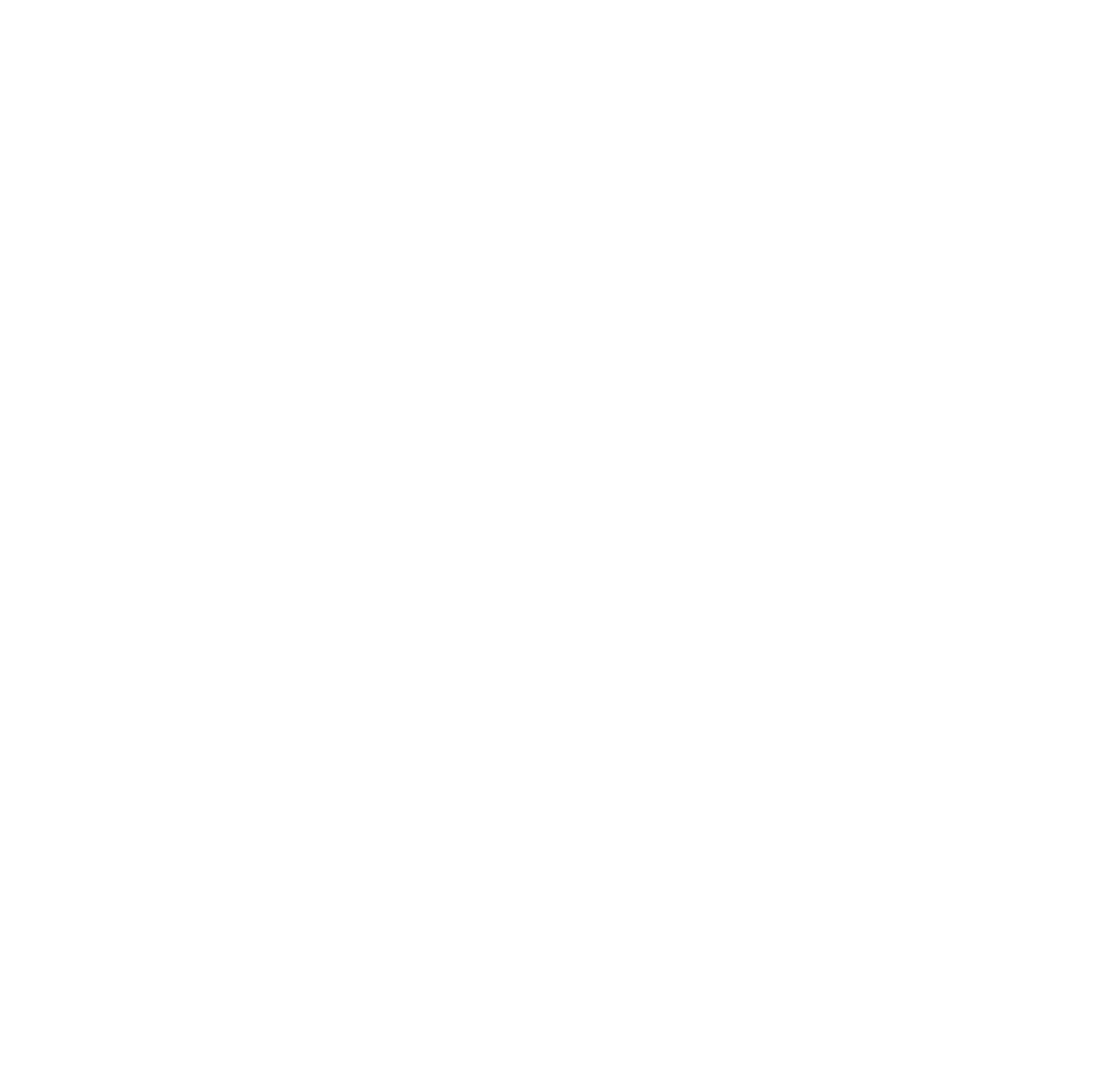
Also in the cancer biology category:
Mitochondria-derived nuclear ATP surge protects against confinement-induced proliferation defects
Teodora Piskova

Spatial transcriptomics elucidates medulla niche supporting germinal center response in myasthenia gravis thymoma
Jessica Chevallier

Invasion of glioma cells through confined space requires membrane tension regulation and mechano-electrical coupling via Plexin-B2
Jade Chan

preListsbioinformatics category:
in the‘In preprints’ from Development 2022-2023
A list of the preprints featured in Development's 'In preprints' articles between 2022-2023
List by | Alex Eve, Katherine Brown |
9th International Symposium on the Biology of Vertebrate Sex Determination
This preList contains preprints discussed during the 9th International Symposium on the Biology of Vertebrate Sex Determination. This conference was held in Kona, Hawaii from April 17th to 21st 2023.
List by | Martin Estermann |
Alumni picks – preLights 5th Birthday
This preList contains preprints that were picked and highlighted by preLights Alumni - an initiative that was set up to mark preLights 5th birthday. More entries will follow throughout February and March 2023.
List by | Sergio Menchero et al. |
Fibroblasts
The advances in fibroblast biology preList explores the recent discoveries and preprints of the fibroblast world. Get ready to immerse yourself with this list created for fibroblasts aficionados and lovers, and beyond. Here, my goal is to include preprints of fibroblast biology, heterogeneity, fate, extracellular matrix, behavior, topography, single-cell atlases, spatial transcriptomics, and their matrix!
List by | Osvaldo Contreras |
Single Cell Biology 2020
A list of preprints mentioned at the Wellcome Genome Campus Single Cell Biology 2020 meeting.
List by | Alex Eve |
Antimicrobials: Discovery, clinical use, and development of resistance
Preprints that describe the discovery of new antimicrobials and any improvements made regarding their clinical use. Includes preprints that detail the factors affecting antimicrobial selection and the development of antimicrobial resistance.
List by | Zhang-He Goh |
Also in the cancer biology category:
BSCB-Biochemical Society 2024 Cell Migration meeting
This preList features preprints that were discussed and presented during the BSCB-Biochemical Society 2024 Cell Migration meeting in Birmingham, UK in April 2024. Kindly put together by Sara Morais da Silva, Reviews Editor at Journal of Cell Science.
List by | Reinier Prosee |
CSHL 87th Symposium: Stem Cells
Preprints mentioned by speakers at the #CSHLsymp23
List by | Alex Eve |
Journal of Cell Science meeting ‘Imaging Cell Dynamics’
This preList highlights the preprints discussed at the JCS meeting 'Imaging Cell Dynamics'. The meeting was held from 14 - 17 May 2023 in Lisbon, Portugal and was organised by Erika Holzbaur, Jennifer Lippincott-Schwartz, Rob Parton and Michael Way.
List by | Helen Zenner |
CellBio 2022 – An ASCB/EMBO Meeting
This preLists features preprints that were discussed and presented during the CellBio 2022 meeting in Washington, DC in December 2022.
List by | Nadja Hümpfer et al. |
Fibroblasts
The advances in fibroblast biology preList explores the recent discoveries and preprints of the fibroblast world. Get ready to immerse yourself with this list created for fibroblasts aficionados and lovers, and beyond. Here, my goal is to include preprints of fibroblast biology, heterogeneity, fate, extracellular matrix, behavior, topography, single-cell atlases, spatial transcriptomics, and their matrix!
List by | Osvaldo Contreras |
Single Cell Biology 2020
A list of preprints mentioned at the Wellcome Genome Campus Single Cell Biology 2020 meeting.
List by | Alex Eve |
ASCB EMBO Annual Meeting 2019
A collection of preprints presented at the 2019 ASCB EMBO Meeting in Washington, DC (December 7-11)
List by | Madhuja Samaddar et al. |
Lung Disease and Regeneration
This preprint list compiles highlights from the field of lung biology.
List by | Rob Hynds |
Anticancer agents: Discovery and clinical use
Preprints that describe the discovery of anticancer agents and their clinical use. Includes both small molecules and macromolecules like biologics.
List by | Zhang-He Goh |
Biophysical Society Annual Meeting 2019
Few of the preprints that were discussed in the recent BPS annual meeting at Baltimore, USA
List by | Joseph Jose Thottacherry |