Retrospective identification of rare cell populations underlying drug resistance connects molecular variability with cell fate
Posted on: 27 April 2020
Preprint posted on 19 March 2020
Turning back time: development of a methodology to identify rare cell states!
Selected by Pavithran RavindranCategories: systems biology
Another preLight was recently published about the study and offers a great summary of this preprint! Click here to read it.
Background
Many biological processes involve a strong bottleneck in which one or a few cells are able to survive and eventually overtake the population. This is especially true in the case of cancer in which drug resistance and metastasis commonly represent cells that are able to survive this strong selection to produce a new tumor1,2. In recent years, it has become increasingly clear that more than simple genetic differences between the cells that survive such bottlenecks there may be non-genetic differences that mark a primed state that help such cells overcome such processes3. In past work, the authors identified such a cell priming state in which melanoma cells simultaneously expressing certain genes (AXL, NGFR, EGFR, etc.) to a high level are able to survive vemurafenib treatment4,5. However, until now there are no good ways to identify such primed states. In this preprint, the authors developed a method to do exactly this in what they have called Rewind.
Key Findings
In this preprint, the authors set out to develop a method to identify primed cells that lead to their ability to pass through a bottleneck (i.e. drug resistance). To do this, they developed a method that coupled RNA FISH and barcoded cells in a system they call Rewind. Essentially their methodology consists of (1) infecting a population of cells with a library of barcodes encoded within the 3’ UTR of a GFP to mark cells, (2) letting them grow up to divide 2-3 times, (3) splitting the population in half, fixing one and using the rest to undergo selection (in this case, drug resistance), (4) sequencing the resulting clones that survived drug treatment for barcodes, and then (5) using RNA FISH to mark and separate out the twins of the cells that survived in the fixed population (Fig 1). From here one could do any number of fixed cell assays such as RNAseq to understand what makes these cells different from the rest of the population.
As a first proof of principle the authors tackled the question of vemurafenib resistance in melanoma. For this, they took a melanoma cell line and performed the Rewind methodology and then performed RNAseq on the rare cells they identified in the original population. With this data they found many of the same markers they found in their original study but also novel ECM/cell migration markers. They verified one of these new genes (ITGA3) by sorting cells high and low for this gene and then treating with vemurafenib finding that the ITGA3-high cells produced 124 drug resistant colonies whereas ITGA3 low cells made 11 resistant colonies. Overall, they were able to show that their Rewind technology allows them to mark and pull out primed cells and understand the molecular differences that lead to this phenotype.
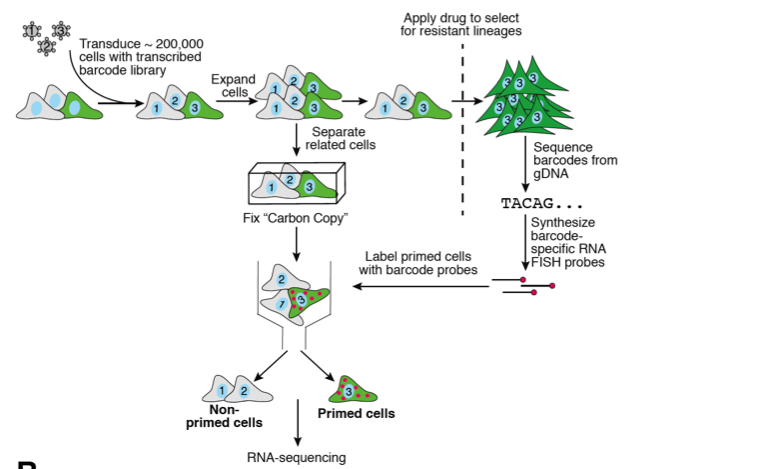
From here the authors wanted to know if the quantity of barcodes in the final resistant population could be used as a proxy for level of resistance and if these portray different subpopulations of rare cells that could eventually lead to different severities of resistant colonies. To test this, they had a similar set up as before but in this case they quantified the number of barcodes in the resistant population and found 2 barcodes with one having ~1500 counts while another had ~400 counts and then produced RNA FISH probes for the highly resistant primed cells and the less resistant primed cells. After validating that these probes did indeed mark colonies with more resistant cells versus fewer cells, they did RNA FISH for 9 of the priming markers initially marked. Using UMAP analysis, they found that non-primed cells very clearly clustered away from primed cells and that within primed cells there were subpopulations for the highly resistant cells and the less resistant ones. From this analysis they found that a gene known as FN1 parked both sub-populations of resistant cells while genes likes AXL and MMP1 seem to more selectively mark the highly resistant versus less resistant cells, respectively. As a final showing of their system, the authors wanted to follow-up on a recent preprint they published describing how more vemurafenib resistant clones grew upon inhibition of a histone methylase known as DOT1L6. One hypothesis would be that this inhibition caused the normal primed cells to grow faster or for more cells to get into the same primed state. Another hypothesis is that this inhibition reveals a completely separate set of cells that are now primed to survive vemurafenib treatment. Indeed, after applying Rewind for this situation, the authors found that this latter situation was true and new, separate sets of cells were primed to become resistant clones but only after DOT1L inhibition. After molecularly profiling these new cells they found that genes such as DEPTOR seemed to mark these DOT1L pre-resistant cells. Overall they concluded that Rewind could not only mark primed cells for a given cell fate but also subpopulations for different variants on such a phenotype.
Why I chose this preprint
I chose this preprint due to the simplicity yet novelty in the Rewind technology that may enable new avenues of research into how rare states may play an important role in defining the ability to take on specific cell fates. The work was extremely well communicated with both easily digestible figures and text. Overall, this preprint was a true joy to read.
Questions for the authors
- How easily does your protocol transfer to new contexts? What are the biggest limitations to setting up this system in a new context?
- In this manuscript you identify that DEPTOR is a marker for DOT1L inhibition dependent pre-resistant cells. Do you believe that there is a causal link between DEPTOR expression and this molecular state or it is simply correlational and there is another cause for this phenotype?
References
- Celià-Terrassa, T. & Kang, Y. Metastatic niche functions and therapeutic opportunities. Nat. Cell Biol. 20, 868–877 (2018).
- Lawson, D. A., Kessenbrock, K., Davis, R. T., Pervolarakis, N. & Werb, Z. Tumour heterogeneity and metastasis at single-cell resolution. Nat. Cell Biol. 20, 1349–1360 (2018).
- Rebecca, V. W. & Herlyn, M. Nongenetic Mechanisms of Drug Resistance in Melanoma. Annu. Rev. Cancer Biol. 4, 315–330 (2020).
- Shaffer, S. M. et al. Rare cell variability and drug-induced reprogramming as a mode of cancer drug resistance. Nat. Publ. Gr. 546, 431–435 (2017).
- Shaffer, S. M. et al. Memory sequencing reveals heritable single cell gene expression programs associated with distinct cellular behaviors. Biorxiv (2018).
- Torre, E. A. et al. Genetic screening for single-cell variability modulators driving therapy resistance Eduardo. BioRXiv (2019).
doi: https://doi.org/10.1242/prelights.19579
Read preprintSign up to customise the site to your preferences and to receive alerts
Register hereAlso in the systems biology category:
Modular control of time and space during vertebrate axis segmentation
AND
Natural genetic variation quantitatively regulates heart rate and dimension
Girish Kale, Jennifer Ann Black

Expressive modeling and fast simulation for dynamic compartments
Benjamin Dominik Maier

Clusters of lineage-specific genes are anchored by ZNF274 in repressive perinucleolar compartments
Silvia Carvalho

preListssystems biology category:
in the‘In preprints’ from Development 2022-2023
A list of the preprints featured in Development's 'In preprints' articles between 2022-2023
List by | Alex Eve, Katherine Brown |
EMBL Synthetic Morphogenesis: From Gene Circuits to Tissue Architecture (2021)
A list of preprints mentioned at the #EESmorphoG virtual meeting in 2021.
List by | Alex Eve |
Single Cell Biology 2020
A list of preprints mentioned at the Wellcome Genome Campus Single Cell Biology 2020 meeting.
List by | Alex Eve |
ASCB EMBO Annual Meeting 2019
A collection of preprints presented at the 2019 ASCB EMBO Meeting in Washington, DC (December 7-11)
List by | Madhuja Samaddar et al. |
EMBL Seeing is Believing – Imaging the Molecular Processes of Life
Preprints discussed at the 2019 edition of Seeing is Believing, at EMBL Heidelberg from the 9th-12th October 2019
List by | Dey Lab |
Pattern formation during development
The aim of this preList is to integrate results about the mechanisms that govern patterning during development, from genes implicated in the processes to theoritical models of pattern formation in nature.
List by | Alexa Sadier |