Applications of Machine Learning in Decision Analysis for Dose Management for Dofetilide
Posted on: 10 February 2019 , updated on: 29 September 2019
Preprint posted on 27 January 2019
Article now published in PLOS ONE at http://dx.doi.org/10.1371/journal.pone.0227324
Optimal Scheduling of Bevacizumab and Pemetrexed/Cisplatin Dosing in Non-Small Cell Lung Cancer
Posted on: , updated on: 29 September 2019
Preprint posted on 5 February 2019
Where dosing dreams are born: Developments in drug toxicity modelling and reinforcement learning in optimising drug dosing regimens
Selected by Zhang-He GohCategories: pharmacology and toxicology
Background of preprints
Dofetilide is a small molecule antiarrhythmic commonly used to treat atrial fibrillation. However, it can give rise to QT-prolongation that can result in fatal arrhythmias, one of its most serious side effects. Coupled with its narrow therapeutic window, a 3-day monitoring period is mandatory for patients in which dofetilide has been initiated. Moreover, most dosing adjustments are made on a non-standard, case-by-case basis. In their preprint, Levy et al aim to resolve this problem by (a) characterising prescribing patterns and (b) applying reinforcement learning to the development of algorithms aimed at successful initiation of dofetilide.
On the other side of the spectrum, bevacizumab is a anti-vascular endothelial growth factor (VEGF) humanised monoclonal antibody. Bevacizumab is known for its many side effects, mostly caused by its extensive effects on the circulatory system. However, clinical trial results tentatively suggest that a combination of bevacizumab and pemetrexed/cisplatin (BEV-PEM/CIS) is an effective first line and maintenance therapy for non-small cell lung cancer (NSCLC). In their preprint, Schneider et al validate and refine the model used to predict the optimal scheduling gap between bevacizumab and PEM/CIS administration by building on their previous work [1].
Key findings of preprints
(A) Levy et al
Levy et al first performed univariate regression on baseline characteristics for successful initiation across the course of dofetilide initiation, showing that the following ten factors were predictors of successful initiation, with the strongest predictors bolded below. Between dose amount and dose adjustment, Levy et al chose the latter to be the target for machine learning approaches, marked with an asterisk (*).
- Dose number
- Dose amount (starting dose < 500 mcg)
- Dose adjustment *
- Ejection fraction
- History of heart failure
- Sinus rhythm
- QRS interval
- Corrected QT interval (QTc)
- Presence of a pacemaker
- Coronary artery disease
The authors then performed supervised, unsupervised, and reinforcement learning on their training set, which consisted 80% of their data set. They achieved good accuracy with tabular and linear reinforcement-learning models, though the latter was highly labile and had unstable weight estimates.
The key findings of their preprint can be summarised into three main points:
- Making dose adjustments at later time points was associated with less probability of successfully initiating dofetilinide.
- None of the supervised learning algorithms were able to improve prediction about providers’ dose decisions based on available clinical information. This difference between supervised and reinforcement learning was attributed to the reliance on human behaviour as the gold standard in the former while leaving the computer to learn by itself in the latter.
- Applying machine learning to healthcare is challenging due to the observational nature of most healthcare studies.
(B) Schneider et al
To expand their work based on results previously obtained in Imbs et al, Schneider et al undertook the following four steps:
- Validated their previously developed semi-mechanistic model, showing that it explained the data better than comparable models. A log-normal constant-error model was used to quantify measurement error as it best fit the data.
- Refined the parameter estimates of the model and used it to predict the optimal scheduling gap between bevacizumab and PEM/CIS administration. One-compartment models with first-order intraperitoneal absorption and elimination were used to describe the pharmacokinetics (PK) of bevacizumab, pemetrexed, and cisplatin; this was based on literature descriptions and PK parameter estimates. A Gompertz function was used to describe unperturbed tumour growth and then the log-kill effects of pemetrexed, cisplatin, and bevacizumab were considered in parallel; the log-kill effect of bevacizumab was deemed insignificant and was thus removed from the model.
- Used stochastic simulations to explore (a) the marginal loss in therapeutic efficacy when BEV-PEM/CIS was administered at a suboptimal gap, (b) the effect of bevacizumab dose scaling on population optimal gap, and (c) the interindividual variability (IIV) of optimal gap. This revealed that the optimal time delay between scheduling bevacizumab and PEM/CIS in mice is 2.0 days, and that the scaling of the dose of bevacizumab had no effect on the optimal gap.
- Scaled the model to estimate optimal BEV-PEM/CIS scheduling in humans using literature human PK/PD models and parameter estimates. IV administration, two-compartment absorption, and first-order elimination models and parameters were used.
Why I chose these preprints
Both preprints by Levy et al and Schneider et al looked at drug regimens with very narrow therapeutic windows; the former considers the dosing of dofetilide therapy, which has an inherent risk of arrhythmias arising from QTc prolongation; the latter considers the dosing of BEV-PEM/CIS dual therapy, whose toxicity largely arises from pemetrexed and cisplatin.
I selected these two preprints in this first post of 2019 as they revisit the important role that modelling plays in healthcare research today. Regardless of whether the drug under investigation is a small molecule (dofetilide) or a large antibody (bevacizumab), the ability to characterise the pharmacology of these drug regimens is becoming especially important for individualised therapy. In recent years, our understanding of pharmacology has advanced sufficiently for healthcare researchers to dextrously walk the fine line between effectiveness and toxicity, allowing us to maximise the use of drugs with narrow therapeutic windows. This research is best applied to older (and usually cheaper) drugs like dofetilide, bevacizumab, pemetrexed, and cisplatin, whose first-line nature likely arises from their traditional use by healthcare professionals.
Future directions
(A) Levy et al
In their preprint, Levy et al identified three limitations in their study, which may be overcome with improvements in auxiliary technologies and big data collection (Table 1).
Table 1. Future directions of the Levy et al preprint.
(B) Schneider et al
Schneider et al foresee that their work can be used to refine cancer treatment modalities by reconfiguring their model to extend predictions to other regimens that include antiangiogenics, including concomitant therapeutics with bevacizumab and other antiangiogenics. More importantly, the model developed by Schneider et al will also be further explored and substantiated by clinical evidence in two ways. First, more data measurements will be collected in mice to overcome problems associated with “sparseness in sampling” that Schneider et al highlighted in their preprint. Second, the model will also be substantiated by data collected in humans undergoing BEV-PEM/CIS therapy.
Other than substantiating the model presented in this preprint with more in vivo and in-human evidence, the insights gained from this preprint can also be expanded in the following ways:
- To inform and justify dosing schedules in future clinical trials involving combinations of BEV-PEM/CIS.
- To explore how the pharmacokinetics of large proteinaceous drugs administered via different routes (intravenously, intramuscularly, etc.) can potentially affect the optimal dosing gap.
- To apply the model developed in this preprint to the potential prediction of Type A adverse drug reactions (ADRs)—adverse effects that directly arise from the drug’s pharmacologic effect. In this case, these Type A ADRs involve vascular side effects that result from bevacizumab’s anti-VEGF and antiangiogenic actions [2].
Questions for authors
(A) Levy et al
- In your analysis, you found that QRS interval was a predictor for successful dofetilide initiation. How was this measured? Would an implication be that having a longer or shorter QRS interval that deviates from the average QRS interval may potentially compromise dofetilide initiation?
- Given that many other drugs, such as macrolide antibiotics and tricyclic antidepressants, also affect QTc prolongation, to what extent are these drug-drug interactions (DDI) likely to affect the incorporation of this model into automated decision-making for dofetilide initiation?
(B) Schneider et al
- The concomitant administration of bevacizumab with chemotherapy in the clinical setting may be due to logistical and practical reasons, such as saving patients a trip to the clinic where the chemotherapy is administered. Based on the current model described in your preprint, would a different route of administration or the formulation of a slower, sustained release preparation of bevacizumab help to mitigate this problem by delaying systemic absorption of bevacizumab?
References
[1] Imbs D-C, El Cheikh R, Boyer A, Ciccolini J, Mascaux C, Lacarelle B, Barlesi F, Barbolosi D, Benzekry S, Revisiting Bevacizumab + Cytotoxics Scheduling Using Mathematical Modeling: Proof of Concept Study in Experimental Non-Small Cell Lung Carcinoma, CPT: Pharmacometrics & Systems Pharmacology 7(1) (2018) 42-50.
[2] Abraham J, Biological mechanisms of bevacizumab-associated adverse events AU – Higa, Gerald M, Expert Review of Anticancer Therapy 9(7) (2009) 999-1007.
Sign up to customise the site to your preferences and to receive alerts
Register hereAlso in the pharmacology and toxicology category:
G6b-B antibody-based cis-acting platelet receptor inhibitors (CAPRIs) as a new family of anti-thrombotic therapeutics
Simon Cleary
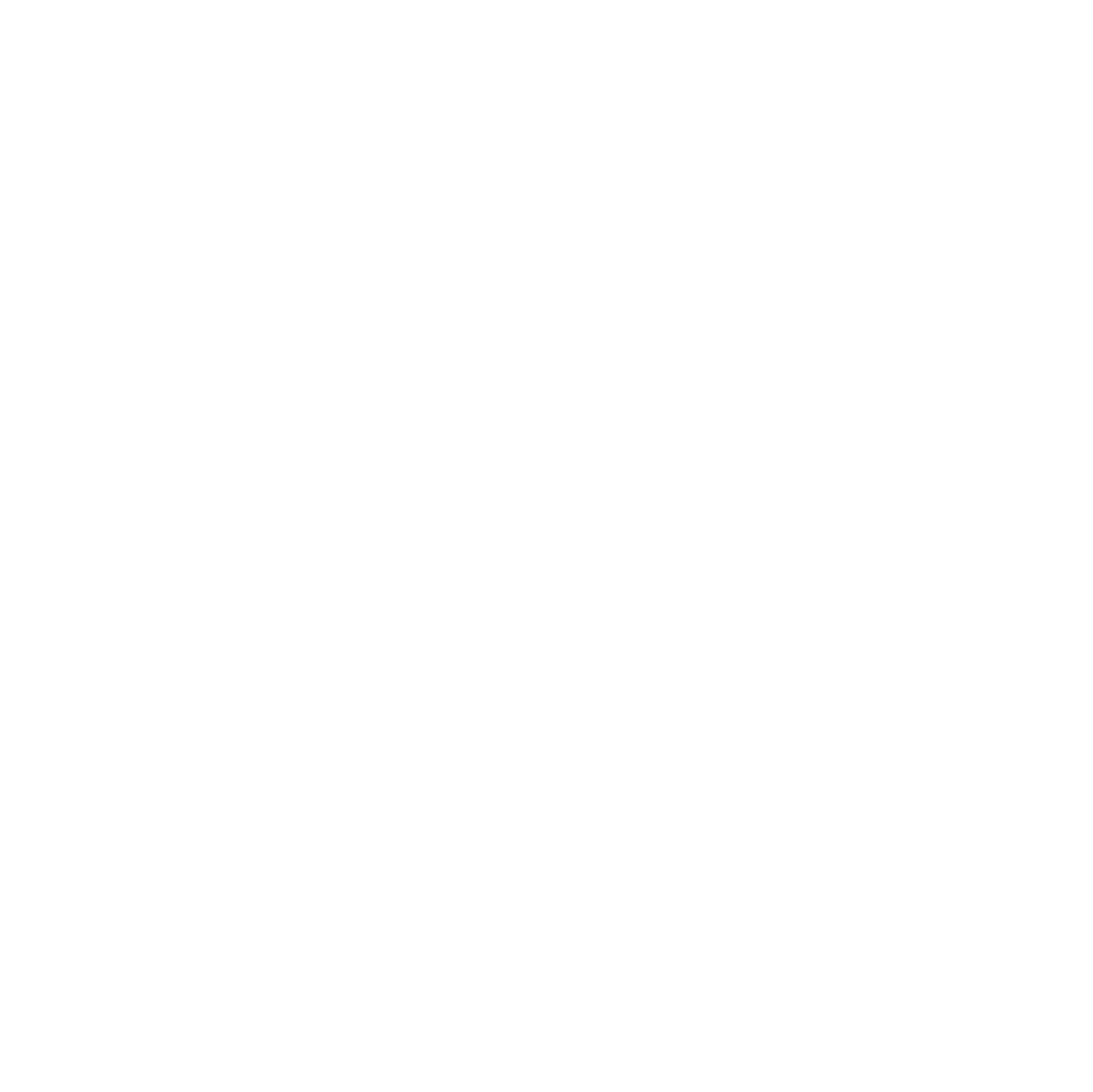
Pervasive sublethal effects of agrochemicals as contributing factors to insect decline
Roberto Amadio

Mixed Alkyl/Aryl Phosphonates Identify Metabolic Serine Hydrolases as Antimalarial Targets
Zhang-He Goh
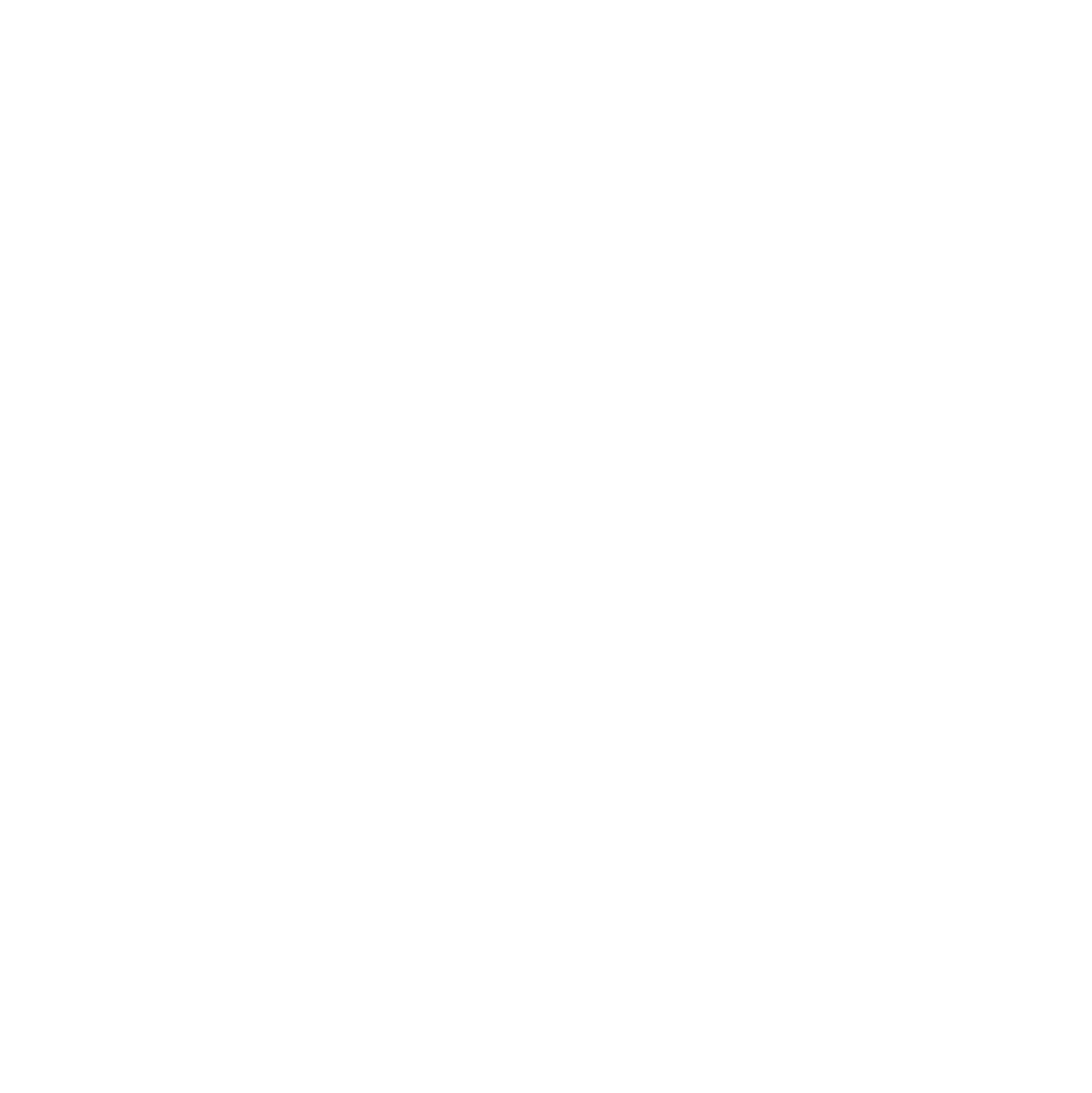
preListspharmacology and toxicology category:
in theFENS 2020
A collection of preprints presented during the virtual meeting of the Federation of European Neuroscience Societies (FENS) in 2020
List by | Ana Dorrego-Rivas |
COVID-19 / SARS-CoV-2 preprints
List of important preprints dealing with the ongoing coronavirus outbreak. See http://covidpreprints.com for additional resources and timeline, and https://connect.biorxiv.org/relate/content/181 for full list of bioRxiv and medRxiv preprints on this topic
List by | Dey Lab, Zhang-He Goh |
1
Drug use in special populations
Any drugs that are being used in special populations: Patients with liver and kidney failure, in paediatrics, in geriatrics, and in pregnant or lactating patients. Includes the discovery of factors that could potentially affect drug use in these special populations.
List by | Zhang-He Goh |
Toxicology of toxicants, existing therapeutics, and investigational drugs
Preprints that describe the toxicology of environmental pollutants and existing and upcoming drugs. Includes both toxicokinetics and toxicodynamics, as well as technological improvements that will help in the characterisation of this field.
List by | Zhang-He Goh |
Antimicrobials: Discovery, clinical use, and development of resistance
Preprints that describe the discovery of new antimicrobials and any improvements made regarding their clinical use. Includes preprints that detail the factors affecting antimicrobial selection and the development of antimicrobial resistance.
List by | Zhang-He Goh |
Anticancer agents: Discovery and clinical use
Preprints that describe the discovery of anticancer agents and their clinical use. Includes both small molecules and macromolecules like biologics.
List by | Zhang-He Goh |
Advances in Drug Delivery
Advances in formulation technology or targeted delivery methods that describe or develop the distribution of small molecules or large macromolecules to specific parts of the body.
List by | Zhang-He Goh |