Gene regulatory network inference and analysis of multidrug-resistant Pseudomonas aeruginosa
Posted on: 19 July 2019 , updated on: 22 July 2019
Preprint posted on 28 July 2019
Article now published in Memórias do Instituto Oswaldo Cruz at http://dx.doi.org/10.1590/0074-02760190105
By taking a holistic, network-based approach, the authors of this study were able to model and study a gene regulatory network for ~1000 genes in a pathogenic, multi-drug resistant strain of Pseudomonas aeruginosa.
Selected by Euan McDonnellCategories: bioinformatics, microbiology, pathology, systems biology
Background
Pseudomonas aeruginosa is a human pathogen that is a major cause of hospital- acquired infections (HAIs), primarily in the skin, lower respiratory tract, urinary tract and eyes, infections that can all lead to more serious manifestations, including bacteremia and endocarditis (1). Of particular concern is the prevalence of multi-drug resistance (MDR) strains, lending to the inclusion of P. aeruginosa as one of the ESKAPE pathogens, a set of MDR bacterial pathogens that exert great economic and social burdens. One such strain is the CCBH4851 which has caused multiple outbreaks in Brazil in recent years, where 35% of strains tested were resistant to carbapenems (a common treatment for P. aeruginosa infection) (2). A plethora of encoded resistance mechanisms confer resistance in P. aeruginosa, including efflux pumps, β-lactamases and biofilm formation, which can all be acquired either endogenously due to mutation or via horizontal gene transfer (2) .
Together, these resistance determinants, alongside less specific regulatory architectures that control their activity, provide elements of redundancy and synergy; however, the mechanisms which underpin them remain poorly understood. This is primarily due to the limitations of individual gene studies and reductionist approaches, which fail to capture the full scale of the networks regulating these processes and other cellular behaviors. In recent years, more holistic analysis methods have been applied to better grasp these networks, and one such set of methodologies is Graph theory (3). It is a set of mathematical doctrines which focuses on the construction of a network model, or a graphical representation of the regulatory network. Such network models aim to simulate the relationships and interactions between various genes, in order to infer underlying regulatory circuits and interactions that can be used to further understand the bacterium or to propose possible drug targets. When applying graph theory, genes take the form of nodes, connected by edges which represent the interaction between genes. Network topology, connectivity and clustering of nodes are all metrics that can then be quantified and used to further describe or infer biological processes and regulatory mechanisms (4). As such, the authors of this paper used existing databases and adapted annotations of other P. aeruginosa strains to CCBH4851 to generate a network model, through which the authors were able to gain insights into the bacterium’s pathology and resistance mechanisms (5).
Key findings
-
They identified a new sub-network not previously described that regulates the polysaccharide synthesis locus. This locus has roles relevant to pathology and resistance, including drug efflux and biofilm formation.
-
They were able to improve on previous network models that focus on the more benign, well-characterised PAO1 strain and were able to identify novel network properties (6). For example, they determined that ~50% of genes had an activatory effect on other genes, which they suggest is important for processes that, once started, need to go to completion. Many of the processes they regulate fit this category, including pathogenic traits, adhesion, virulence, resistance factors and biofilm formation.
-
Many genes undergo auto-regulation, where gene’s can influence their own expression via either up-regulation or down-regulation. However they identified that the majority of genes that exhibit auto-regulation have a negative effect, meaning that they repress their own expression, which provides robustness for the system to external fluctuations. Most of these genes were associated with factors regulating biosynthesis and they suggest that negative autoregulation enables P. aeruginosa to prevent wastage of resources.
-
Finally, they identified various “hub” genes, or genes that interact with many other genes, including lasR, fur and np20. These are ideal targets for drugs as their disruption will have a greater effect on cellular behaviour than less connected genes.
Why I chose this paper
Given the looming threat of prevalent anti-microbial resistance combined with the low rate of novel antibiotic discovery, we are in dire need of new methodologies to identify novel drug targets. This paper represents one such method that is able to i) identify and understand key regulatory mechanisms that are relevant to pathogenesis and ii) propose a list of candidate hub genes that are possible targets for drug development. Moreover, they were able to perform this analysis entirely computationally, only utilizing previously published data meaning that such studies are low-cost and relatively easy to scale. Such holistic analyses have proved effective in understanding the behavior of viral infections and cancers and providing guidance for drug development. As such, I think that these studies will become much more abundant in future years, given the abundance of publicly-available data and the wealth of insight that such analyses provide (3,4,7).
Future perspectives
-
I think further investigation into the “druggability” of some of these hub genes would be beneficial, alongside application of the understanding acquired to better prevent and control infections by P. aeruginosa, particularly in cystic fibrosis patients.
-
I think it would be beneficial to apply this analysis to other bacterial pathogens of the ESKAPE classification, given that these too are huge burdens on the human population.
-
Deletion and mutation of various hub genes and fitness studies could be performed to further determine ideal candidates.
-
Given that hub genes are most likely to be evolutionary ancient and thus more likely to share homology with human orthologs, phylogenetic analysis and sequence comparison could be performed to further filter candidates that are inappropriate due to similarity with human genes.
Bibliography
-
Lyczak JB, Cannon CL, Pier GB. Establishment of Pseudomonas aeruginosa infection: lessons from a versatile opportunist. Microbes Infect [Internet]. 2000 Jul 1 [cited 2019 Jul 9];2(9):1051–60. Available from: https://www.sciencedirect.com/science/article/pii/S1286457900012594
-
Maraolo AE, Cascella M, Corcione S, Cuomo A, Nappa S, Borgia G, et al. Management of multidrug- resistant Pseudomonas aeruginosa in the intensive care unit: state of the art. Expert Rev Anti Infect Ther [Internet]. 2017 Sep 2 [cited 2019 Jul 9];15(9):861–71. Available from: https://www.tandfonline.com/doi/full/10.1080/14787210.2017.1367666
-
Pavlopoulos GA, Secrier M, Moschopoulos CN, Soldatos TG, Kossida S, Aerts J, et al. Using graph theory to analyze biological networks. BioData Min [Internet]. 2011 Dec 28 [cited 2019 Jul 9];4(1):10. Available from: https://biodatamining.biomedcentral.com/articles/10.1186/1756-0381-4-10
-
Arrell DK, Terzic A. Network Systems Biology for Drug Discovery. Clin Pharmacol Ther [Internet]. 2010 Jul 2 [cited 2019 Jul 9];88(1):120–5. Available from: http://doi.wiley.com/10.1038/clpt.2010.91
-
Filho FM, Nascimento APB do, Santos MT dos, Carvalho-Assef APD, Silva FAB da. Gene regulatory network inference and analysis of multidrug-resistant Pseudomonas aeruginosa. bioRxiv [Internet]. 2019 Jun 28 [cited 2019 Jul 9];610493. Available from: https://www.biorxiv.org/content/10.1101/610493v2.full
-
Stover CK, Pham XQ, Erwin AL, Mizoguchi SD, Warrener P, Hickey MJ, et al. Complete genome sequence of Pseudomonas aeruginosa PAO1, an opportunistic pathogen. Nature [Internet]. 2000 Aug [cited 2019 Jul 9];406(6799):959–64. Available from: http://www.nature.com/articles/35023079
-
Mason O, Verwoerd M. Graph theory and networks in Biology. IET Syst Biol [Internet]. 2007 Mar 1 [cited 2019 Jul 9];1(2):89–119. Available from: https://digital- library.theiet.org/content/journals/10.1049/iet-syb_20060038
doi: https://doi.org/10.1242/prelights.12282
Read preprintSign up to customise the site to your preferences and to receive alerts
Register hereAlso in the bioinformatics category:
Enhancer-driven cell type comparison reveals similarities between the mammalian and bird pallium
Rodrigo Senovilla-Ganzo

Expressive modeling and fast simulation for dynamic compartments
Benjamin Dominik Maier

Transcriptional profiling of human brain cortex identifies novel lncRNA-mediated networks dysregulated in amyotrophic lateral sclerosis
Julio Molina Pineda
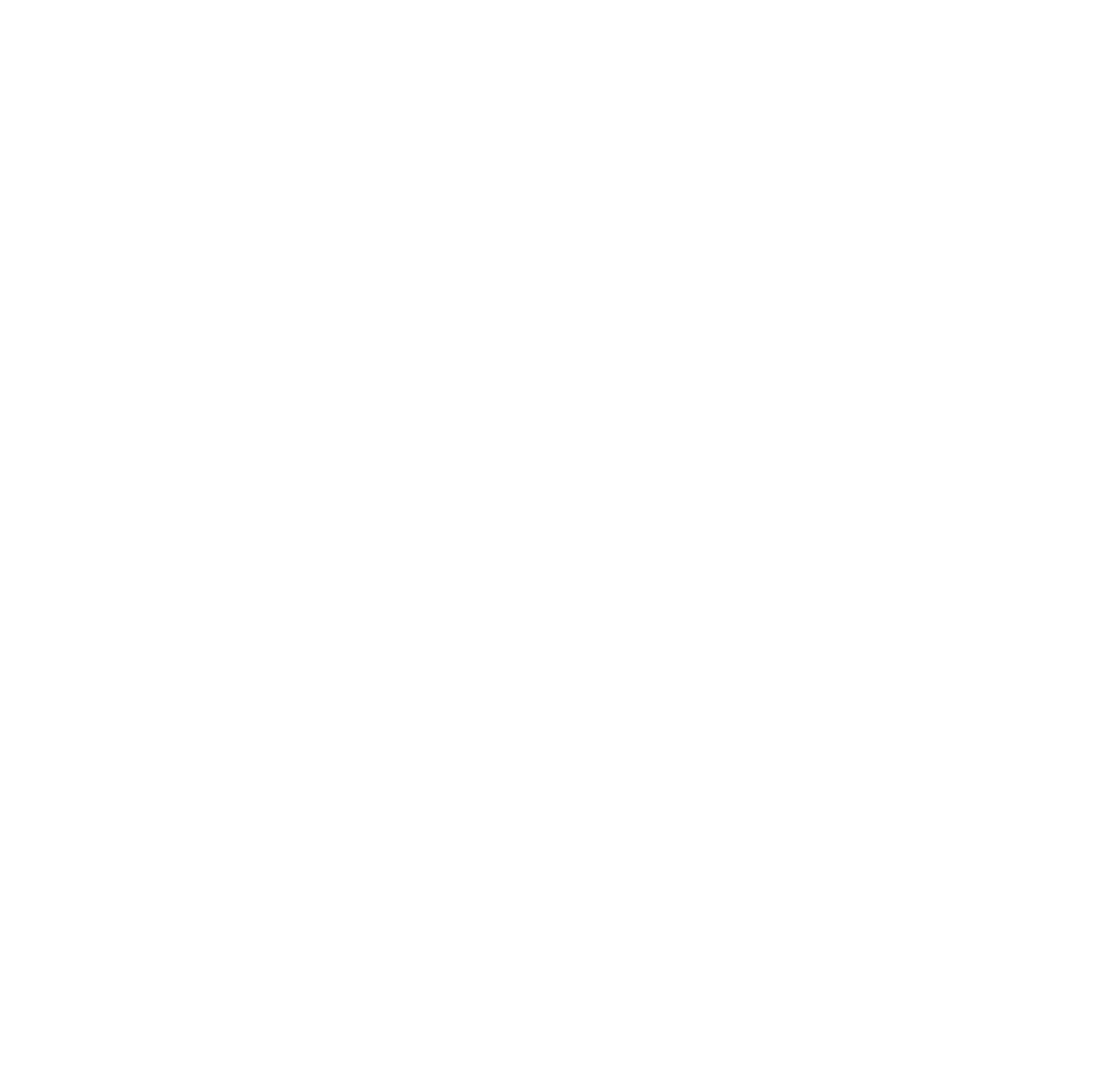
Also in the microbiology category:
Characterization of natural product inhibitors of quorum sensing in Pseudomonas aeruginosa reveals competitive inhibition of RhlR by ortho-vanillin
UofA IMB565 et al.
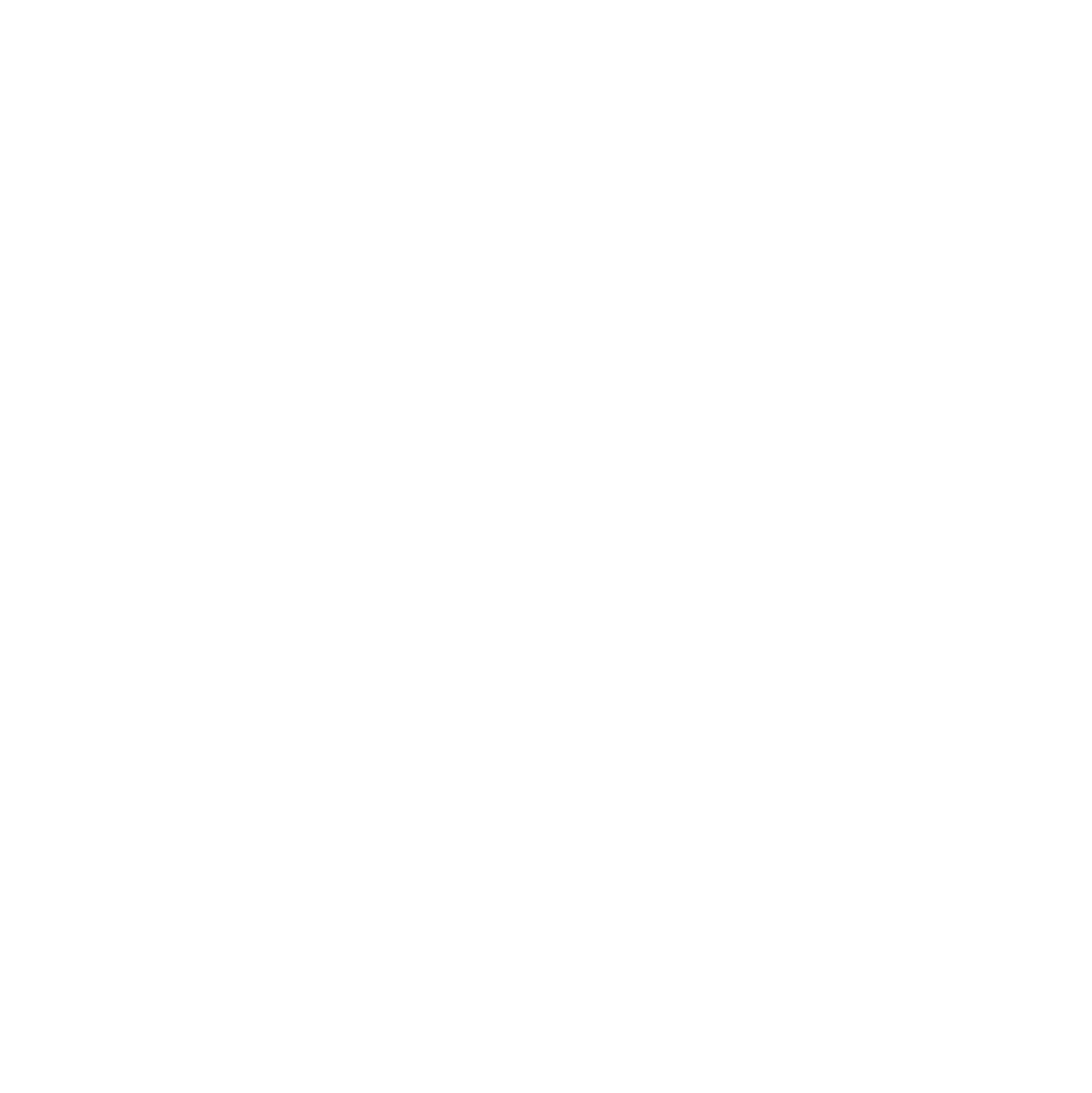
Feedback loop regulation between viperin and viral hemorrhagic septicemia virus through competing protein degradation pathways
UofA IMB565 et al.

Lytic bacteriophages interact with respiratory epithelial cells and induce the secretion of antiviral and proinflammatory cytokines
UofA IMB565 et al.
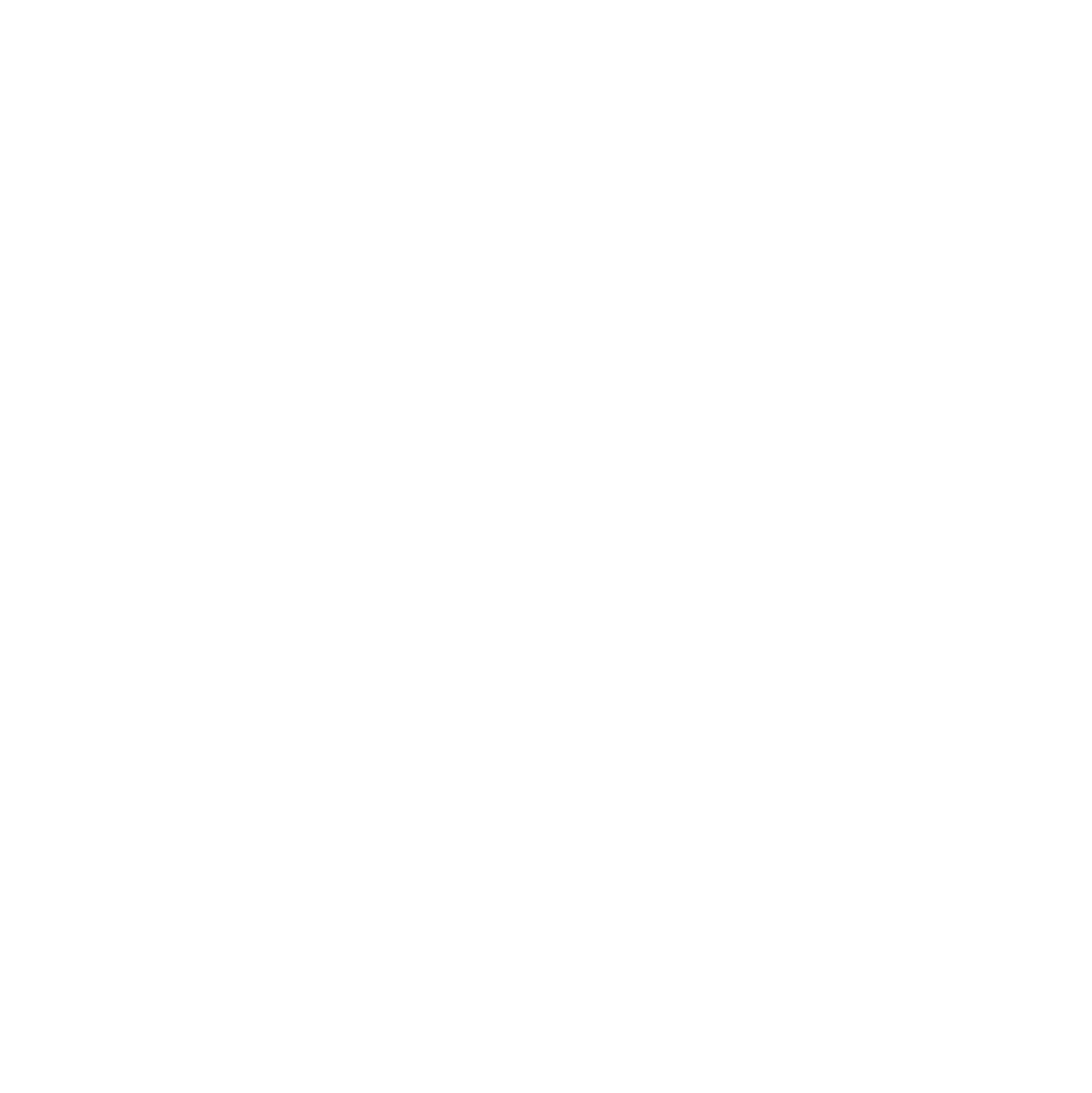
Also in the pathology category:
LINC complex alterations are a hallmark of sporadic and familial ALS/FTD
Megane Rayer et al.

Hypoxia blunts angiogenic signaling and upregulates the antioxidant system in elephant seal endothelial cells
Sarah Young-Veenstra

H2O2 sulfenylates CHE linking local infection to establishment of systemic acquired resistance
Marc Somssich
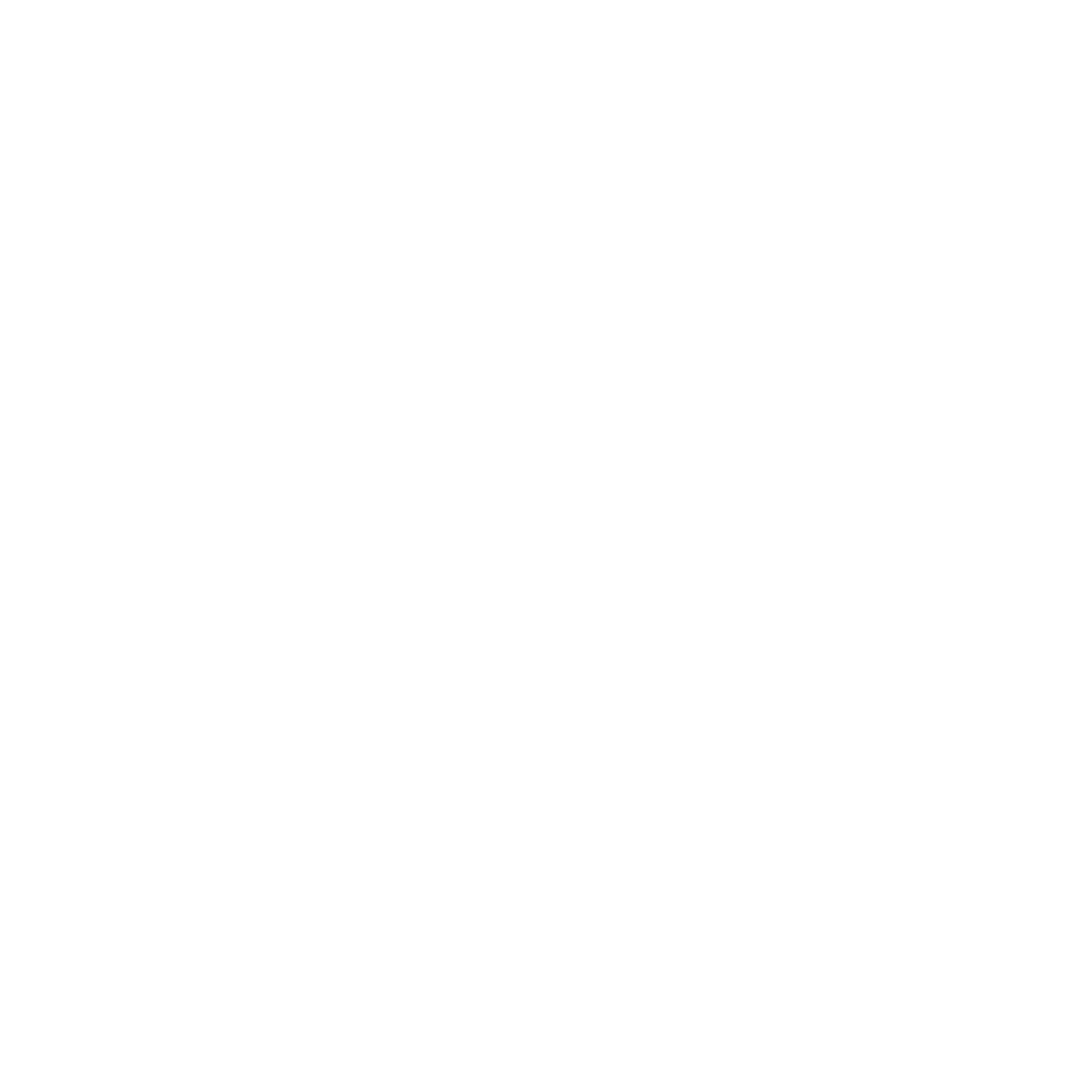
Also in the systems biology category:
Modular control of time and space during vertebrate axis segmentation
AND
Natural genetic variation quantitatively regulates heart rate and dimension
Girish Kale, Jennifer Ann Black

Expressive modeling and fast simulation for dynamic compartments
Benjamin Dominik Maier

Clusters of lineage-specific genes are anchored by ZNF274 in repressive perinucleolar compartments
Silvia Carvalho

preListsbioinformatics category:
in the‘In preprints’ from Development 2022-2023
A list of the preprints featured in Development's 'In preprints' articles between 2022-2023
List by | Alex Eve, Katherine Brown |
9th International Symposium on the Biology of Vertebrate Sex Determination
This preList contains preprints discussed during the 9th International Symposium on the Biology of Vertebrate Sex Determination. This conference was held in Kona, Hawaii from April 17th to 21st 2023.
List by | Martin Estermann |
Alumni picks – preLights 5th Birthday
This preList contains preprints that were picked and highlighted by preLights Alumni - an initiative that was set up to mark preLights 5th birthday. More entries will follow throughout February and March 2023.
List by | Sergio Menchero et al. |
Fibroblasts
The advances in fibroblast biology preList explores the recent discoveries and preprints of the fibroblast world. Get ready to immerse yourself with this list created for fibroblasts aficionados and lovers, and beyond. Here, my goal is to include preprints of fibroblast biology, heterogeneity, fate, extracellular matrix, behavior, topography, single-cell atlases, spatial transcriptomics, and their matrix!
List by | Osvaldo Contreras |
Single Cell Biology 2020
A list of preprints mentioned at the Wellcome Genome Campus Single Cell Biology 2020 meeting.
List by | Alex Eve |
Antimicrobials: Discovery, clinical use, and development of resistance
Preprints that describe the discovery of new antimicrobials and any improvements made regarding their clinical use. Includes preprints that detail the factors affecting antimicrobial selection and the development of antimicrobial resistance.
List by | Zhang-He Goh |
Also in the microbiology category:
BioMalPar XVI: Biology and Pathology of the Malaria Parasite
[under construction] Preprints presented at the (fully virtual) EMBL BioMalPar XVI, 17-18 May 2020 #emblmalaria
List by | Dey Lab, Samantha Seah |
1
ECFG15 – Fungal biology
Preprints presented at 15th European Conference on Fungal Genetics 17-20 February 2020 Rome
List by | Hiral Shah |
EMBL Seeing is Believing – Imaging the Molecular Processes of Life
Preprints discussed at the 2019 edition of Seeing is Believing, at EMBL Heidelberg from the 9th-12th October 2019
List by | Dey Lab |
Also in the pathology category:
Fibroblasts
The advances in fibroblast biology preList explores the recent discoveries and preprints of the fibroblast world. Get ready to immerse yourself with this list created for fibroblasts aficionados and lovers, and beyond. Here, my goal is to include preprints of fibroblast biology, heterogeneity, fate, extracellular matrix, behavior, topography, single-cell atlases, spatial transcriptomics, and their matrix!
List by | Osvaldo Contreras |
ECFG15 – Fungal biology
Preprints presented at 15th European Conference on Fungal Genetics 17-20 February 2020 Rome
List by | Hiral Shah |
COVID-19 / SARS-CoV-2 preprints
List of important preprints dealing with the ongoing coronavirus outbreak. See http://covidpreprints.com for additional resources and timeline, and https://connect.biorxiv.org/relate/content/181 for full list of bioRxiv and medRxiv preprints on this topic
List by | Dey Lab, Zhang-He Goh |
1
Cellular metabolism
A curated list of preprints related to cellular metabolism at Biorxiv by Pablo Ranea Robles from the Prelights community. Special interest on lipid metabolism, peroxisomes and mitochondria.
List by | Pablo Ranea Robles |
Also in the systems biology category:
‘In preprints’ from Development 2022-2023
A list of the preprints featured in Development's 'In preprints' articles between 2022-2023
List by | Alex Eve, Katherine Brown |
EMBL Synthetic Morphogenesis: From Gene Circuits to Tissue Architecture (2021)
A list of preprints mentioned at the #EESmorphoG virtual meeting in 2021.
List by | Alex Eve |
Single Cell Biology 2020
A list of preprints mentioned at the Wellcome Genome Campus Single Cell Biology 2020 meeting.
List by | Alex Eve |
ASCB EMBO Annual Meeting 2019
A collection of preprints presented at the 2019 ASCB EMBO Meeting in Washington, DC (December 7-11)
List by | Madhuja Samaddar et al. |
EMBL Seeing is Believing – Imaging the Molecular Processes of Life
Preprints discussed at the 2019 edition of Seeing is Believing, at EMBL Heidelberg from the 9th-12th October 2019
List by | Dey Lab |
Pattern formation during development
The aim of this preList is to integrate results about the mechanisms that govern patterning during development, from genes implicated in the processes to theoritical models of pattern formation in nature.
List by | Alexa Sadier |