The landscape of antigen-specific T cells in human cancers
Posted on: 19 November 2018
Preprint posted on 1 November 2018
Identifying the specific targets of T cells that can destroy cancer cells is a priority in the field of immunotherapy. Here, the authors use a clustering approach in pan-cancer RNAseq datasets to discover new recurrent targets.
Selected by Rob HyndsCategories: bioinformatics, cancer biology, immunology
Background
Antigen-specific tumour-infiltrating lymphocytes (TILs) can mediate tumour destruction but identifying the specificity of these is a challenge due to the variety of possible sources (including single nucleotide variants (SNVs), insertion or deletions (indels), re-expression of developmental antigens, intron retention etc.), the diversity of the antigen binding region and the promiscuous binding of antigenic peptides. This study attempts to develop and test an improved strategy to study TCR repertoires associated with cancer by leveraging publicly available datasets.
Highlights
- A new pipeline to identify clusters of recurrent TCR complementarity-determining regions (CDRs; also known as hypervariable regions) from TGCA RNAseq that are not present in healthy controls.
- Gene expression analysis shows CDR3 (which is the most variable of the three CDRs) clusters are from samples with an enriched T cell activation signature.
- Clustering CDR3s reveals a CD8+ T cell subset with a Trm signature. The differentiation pathway of these cells is mapped using trajectory analysis.
- Clustered CDR3s are used to identify neoantigens and cancer-associated antigens.
- It is possible to detect cancer-associated CDR3s in early breast cancer samples so this protocol may be relevant for early detection of cancers.
Detecting cancer-associated CDR3s
Using an algorithm (TRUST), the authors identified 1.5 x 106 CDR3 sequences in over 9,000 (pan-cancer) TGCA RNAseq samples. Around 170,000 of these were complete productive sequences and after excluding sequences that are also found in a healthy population, the remaining 82,000 sequences were dubbed ‘cancer-associated’. These CDR3 sequences were clustered (using ‘iSMART’), generating 4501 clusters containing 15,254 sequences. Given the probability of sequences that are not found in the healthy population co-occuring in multiple individuals is low, they assume that these share antigen specificity.
Relationship of clusters with gene expression
The authors go on to correlate gene expression and the number of clustered CDR3s in samples and find that genes involved in immune response (inc. T cell activation) are positively correlated, while a number of immune inhibitory genes are negatively correlated. They conclude that samples with clustered CDR3s are enriched for activated TILs. Next, single cell RNAseq data is used to look at the phenotype of T cell clonotypes with clustered CDR3s and a CD8+ subpopulation that has high T cell cytotoxicity and exhaustion scores (resembling a recently described Trm cell signature) is found. Trajectory analysis sheds some light on Trm differentiation: early in pseudotime cells express precursor markers and low levels of exhaustion markers whereas later, two populations emerge. The metabolic activity of one is low, while the other is high. The highly metabolic population comes from low metabolic activity differentiated cells and not directly from progenitors. Analysis of individual clonotypes shows this is dependent on receptor sequence.
Figure 2C from the preprint shows the pseudotime trajectory plot that depicts the inferred Trm differentiation pathway.
Neoantigen identification
The authors sought associations between recurrent SNVs (>3) and CDR3 clusters to see whether genomic aberrations generate abnormal proteins that are recognised by the immune system. Six such associations were significant, four of which are predicted to bind to HLA molecules. Two patients with these mutations also had a matched HLA genotype. As well as SNVs, the authors looked at indels, identifying 10 significant pairs despite a five-fold lower number of indels compared to SNVs. Four were confirmed in RNAseq data (which is important as mutant transcripts can be degraded by nonsense-mediated decay); interestingly two hits are Wnt pathway genes and all of these samples are from stomach cancer patients with high microsatellite instability and indels in short-tandem repeat regions. The validity of this approach is supported by the fact that these patients have a good clinical response to checkpoint inhibition.
Cancer-associated antigen identification
Differential gene expression analysis for CDR3 clusters identified two clusters with distinct CDR3 patterns that targeted the gene HSFX1. These were derived from colon and endometrial cancer samples. Since HSFX1 is lowly expressed by normal tissues but is expressed in 13% CRC and 75% of endometrial cancers and high expression of HSFX1 is a positive prognostic indicator in endometrial cancer, it is a promising cancer associated antigen candidate. Strong binding of HSFX1 peptides was predicted to three common HLA alleles (including HLA-A*02:01) so the authors synthesised 9-mer peptides and injected them into humanised mice with HLA-A*02:01. IFNg ELISPOT on splenocytes from these mice demonstrated a response, suggesting the immunoreactivity of these peptides in vivo. The authors conclude that these likely escape central tolerance and could act as cancer-associated antigens in these cancer types.
Early detection relevance
The authors ask whether it’s possible to identify CDR3 clusters in PBMCs (rather than TILs) as this could allow early detection of cancer using peripheral blood samples. There were more cancer-associated CDR3s in 16 early stage breast cancer PBMC samples than healthy controls. Interestingly, these are fewer in number than in an advanced melanoma cohort but those that are found are detected at higher abundance. The authors suggest this is due to Trm differentiation reducing clonotype frequencies in more advanced tumours.
Questions for the authors
Q1) Could the use of healthy controls to filter out ‘non-cancer’ CDR3 sequences mean that co-morbidities contribute the ‘cancer-associated’ list? For example, are cancer patients more likely to have viral-reactive TCRs?
Q2) Few neoantigen-TCR pairs are detected, do the authors think that sequencing or analysis factors are more important in this? Have they tried the pipeline in TCR-enriched datasets?
Q3) Do the authors have a hypothesis for how receptor sequence influences Trm differentiation?
doi: https://doi.org/10.1242/prelights.5692
Read preprintSign up to customise the site to your preferences and to receive alerts
Register hereAlso in the bioinformatics category:
Enhancer-driven cell type comparison reveals similarities between the mammalian and bird pallium
Rodrigo Senovilla-Ganzo

Expressive modeling and fast simulation for dynamic compartments
Benjamin Dominik Maier

Transcriptional profiling of human brain cortex identifies novel lncRNA-mediated networks dysregulated in amyotrophic lateral sclerosis
Julio Molina Pineda
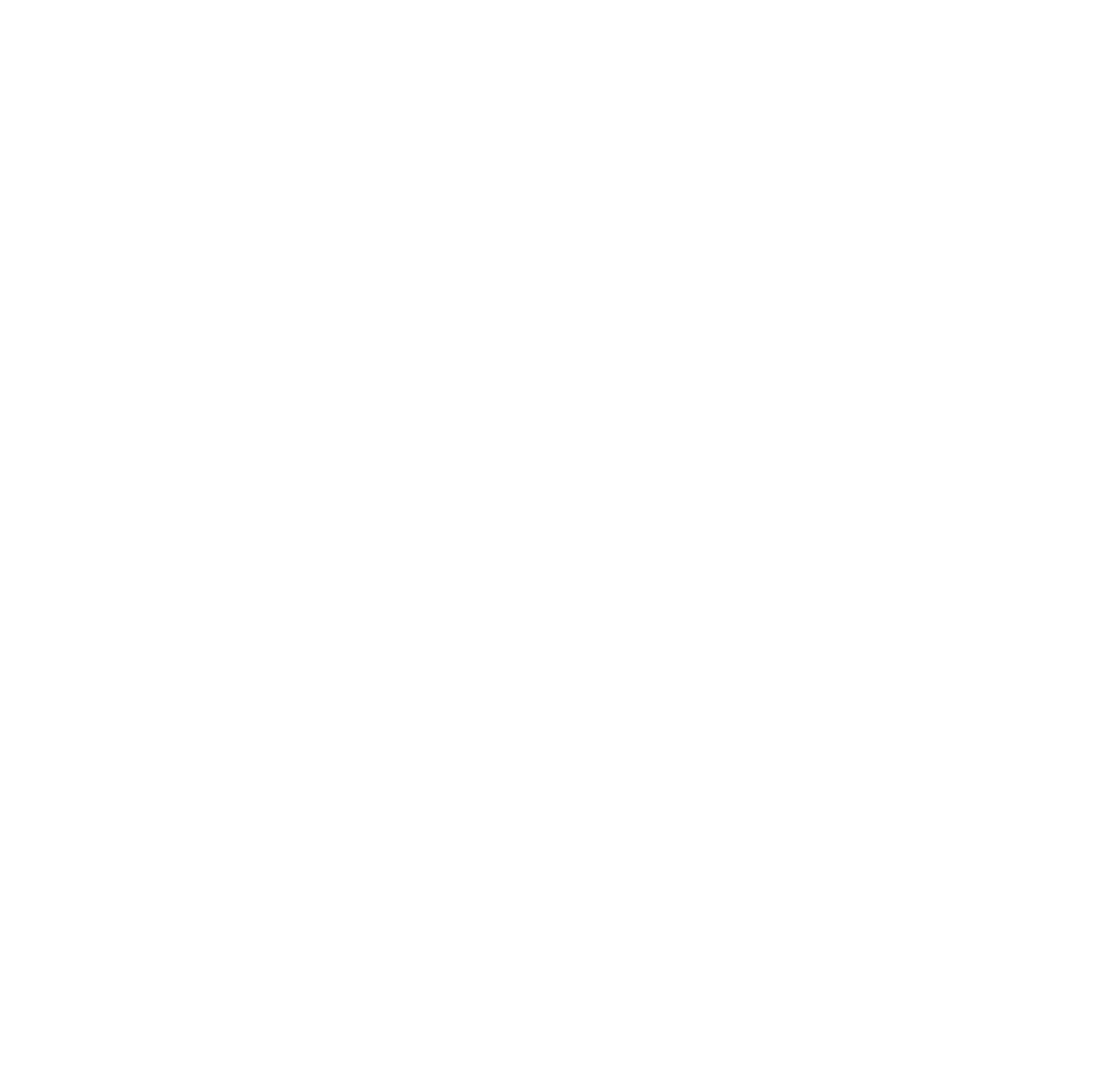
Also in the cancer biology category:
Mitochondria-derived nuclear ATP surge protects against confinement-induced proliferation defects
Teodora Piskova

Spatial transcriptomics elucidates medulla niche supporting germinal center response in myasthenia gravis thymoma
Jessica Chevallier

Invasion of glioma cells through confined space requires membrane tension regulation and mechano-electrical coupling via Plexin-B2
Jade Chan

Also in the immunology category:
Control of Inflammatory Response by Tissue Microenvironment
Roberto Amadio

G6b-B antibody-based cis-acting platelet receptor inhibitors (CAPRIs) as a new family of anti-thrombotic therapeutics
Simon Cleary
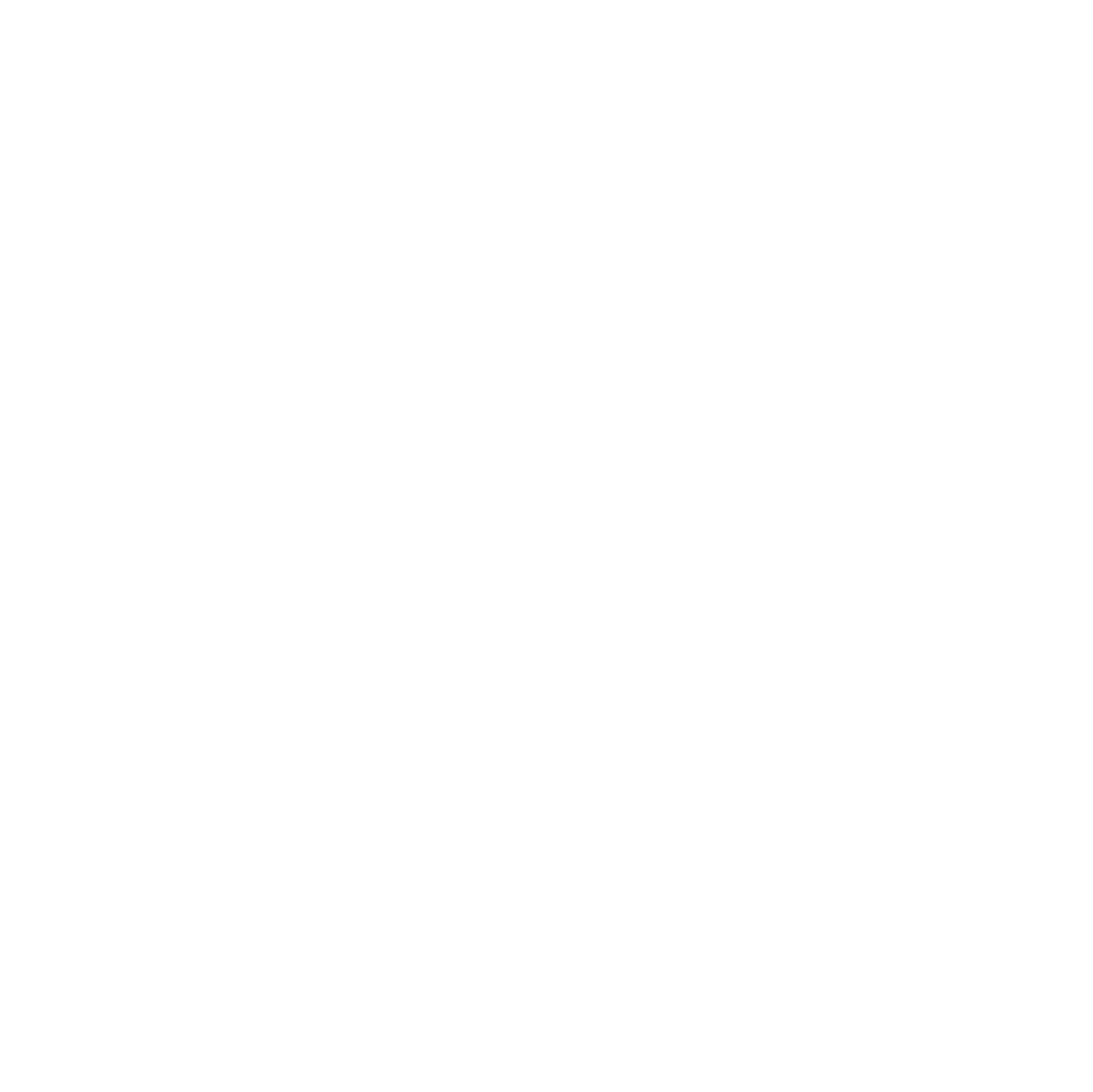
Feedback loop regulation between viperin and viral hemorrhagic septicemia virus through competing protein degradation pathways
UofA IMB565 et al.

preListsbioinformatics category:
in the‘In preprints’ from Development 2022-2023
A list of the preprints featured in Development's 'In preprints' articles between 2022-2023
List by | Alex Eve, Katherine Brown |
9th International Symposium on the Biology of Vertebrate Sex Determination
This preList contains preprints discussed during the 9th International Symposium on the Biology of Vertebrate Sex Determination. This conference was held in Kona, Hawaii from April 17th to 21st 2023.
List by | Martin Estermann |
Alumni picks – preLights 5th Birthday
This preList contains preprints that were picked and highlighted by preLights Alumni - an initiative that was set up to mark preLights 5th birthday. More entries will follow throughout February and March 2023.
List by | Sergio Menchero et al. |
Fibroblasts
The advances in fibroblast biology preList explores the recent discoveries and preprints of the fibroblast world. Get ready to immerse yourself with this list created for fibroblasts aficionados and lovers, and beyond. Here, my goal is to include preprints of fibroblast biology, heterogeneity, fate, extracellular matrix, behavior, topography, single-cell atlases, spatial transcriptomics, and their matrix!
List by | Osvaldo Contreras |
Single Cell Biology 2020
A list of preprints mentioned at the Wellcome Genome Campus Single Cell Biology 2020 meeting.
List by | Alex Eve |
Antimicrobials: Discovery, clinical use, and development of resistance
Preprints that describe the discovery of new antimicrobials and any improvements made regarding their clinical use. Includes preprints that detail the factors affecting antimicrobial selection and the development of antimicrobial resistance.
List by | Zhang-He Goh |
Also in the cancer biology category:
BSCB-Biochemical Society 2024 Cell Migration meeting
This preList features preprints that were discussed and presented during the BSCB-Biochemical Society 2024 Cell Migration meeting in Birmingham, UK in April 2024. Kindly put together by Sara Morais da Silva, Reviews Editor at Journal of Cell Science.
List by | Reinier Prosee |
CSHL 87th Symposium: Stem Cells
Preprints mentioned by speakers at the #CSHLsymp23
List by | Alex Eve |
Journal of Cell Science meeting ‘Imaging Cell Dynamics’
This preList highlights the preprints discussed at the JCS meeting 'Imaging Cell Dynamics'. The meeting was held from 14 - 17 May 2023 in Lisbon, Portugal and was organised by Erika Holzbaur, Jennifer Lippincott-Schwartz, Rob Parton and Michael Way.
List by | Helen Zenner |
CellBio 2022 – An ASCB/EMBO Meeting
This preLists features preprints that were discussed and presented during the CellBio 2022 meeting in Washington, DC in December 2022.
List by | Nadja Hümpfer et al. |
Fibroblasts
The advances in fibroblast biology preList explores the recent discoveries and preprints of the fibroblast world. Get ready to immerse yourself with this list created for fibroblasts aficionados and lovers, and beyond. Here, my goal is to include preprints of fibroblast biology, heterogeneity, fate, extracellular matrix, behavior, topography, single-cell atlases, spatial transcriptomics, and their matrix!
List by | Osvaldo Contreras |
Single Cell Biology 2020
A list of preprints mentioned at the Wellcome Genome Campus Single Cell Biology 2020 meeting.
List by | Alex Eve |
ASCB EMBO Annual Meeting 2019
A collection of preprints presented at the 2019 ASCB EMBO Meeting in Washington, DC (December 7-11)
List by | Madhuja Samaddar et al. |
Lung Disease and Regeneration
This preprint list compiles highlights from the field of lung biology.
List by | Rob Hynds |
Anticancer agents: Discovery and clinical use
Preprints that describe the discovery of anticancer agents and their clinical use. Includes both small molecules and macromolecules like biologics.
List by | Zhang-He Goh |
Biophysical Society Annual Meeting 2019
Few of the preprints that were discussed in the recent BPS annual meeting at Baltimore, USA
List by | Joseph Jose Thottacherry |
Also in the immunology category:
Journal of Cell Science meeting ‘Imaging Cell Dynamics’
This preList highlights the preprints discussed at the JCS meeting 'Imaging Cell Dynamics'. The meeting was held from 14 - 17 May 2023 in Lisbon, Portugal and was organised by Erika Holzbaur, Jennifer Lippincott-Schwartz, Rob Parton and Michael Way.
List by | Helen Zenner |
Fibroblasts
The advances in fibroblast biology preList explores the recent discoveries and preprints of the fibroblast world. Get ready to immerse yourself with this list created for fibroblasts aficionados and lovers, and beyond. Here, my goal is to include preprints of fibroblast biology, heterogeneity, fate, extracellular matrix, behavior, topography, single-cell atlases, spatial transcriptomics, and their matrix!
List by | Osvaldo Contreras |
Single Cell Biology 2020
A list of preprints mentioned at the Wellcome Genome Campus Single Cell Biology 2020 meeting.
List by | Alex Eve |
Autophagy
Preprints on autophagy and lysosomal degradation and its role in neurodegeneration and disease. Includes molecular mechanisms, upstream signalling and regulation as well as studies on pharmaceutical interventions to upregulate the process.
List by | Sandra Malmgren Hill |
Antimicrobials: Discovery, clinical use, and development of resistance
Preprints that describe the discovery of new antimicrobials and any improvements made regarding their clinical use. Includes preprints that detail the factors affecting antimicrobial selection and the development of antimicrobial resistance.
List by | Zhang-He Goh |
Zebrafish immunology
A compilation of cutting-edge research that uses the zebrafish as a model system to elucidate novel immunological mechanisms in health and disease.
List by | Shikha Nayar |