Opposing influence of top-down and bottom-up input on different types of excitatory layer 2/3 neurons in mouse visual cortex
Posted on: 7 April 2020
Preprint posted on 23 March 2020
Article now published in Neuron at http://dx.doi.org/10.1016/j.neuron.2020.09.024
Intracellular mismatch! Jordan and Keller record membrane voltage of V1 pyramidal neurons during visuomotor mismatch responses in mice, showing layer specific prediction error computations.
Selected by Mahesh KarnaniCategories: neuroscience, physiology
Context
Predictive coding is a classic topic in neuroscience because prediction is central to the function of nervous systems. From accurately maintaining a 2-m distance with coughing people on the street to planning a week of remote work, generating predictions is a key brain process. In the past decade, Georg Keller’s lab has lead the effort in studying predictive processing in the mouse primary visual cortex (V1)1. Their work has typically consisted of Ca2+ imaging of neural activity across multiple neural cell types during a visuomotor mismatch paradigm2. This paradigm, also used in the preprint discussed here, entails a head-fixed mouse running in a visual virtual reality corridor where the speed of the visual flow is coupled to movement of a floating styrofoam ball, which the mouse moves as it runs. The visual flow is then suddenly stopped for 1 second, causing a mismatch between visual flow and mouse movement, which triggers robust mismatch responses in the V1 neurons.
The general framework of predictive coding with a forward model is well explained in [ref 1] and the below video. The hypothesis calls for the existence of three key nodes: representation neurons (observation of external world), prediction neurons (internal forward model) and prediction error neurons (comparison of observation and internal prediction). In the visuomotor mismatch paradigm, the internal forward model should predict expected visual flow speed based on locomotion speed, constituting an efference copy. If the hypothesis holds, the prediction error neurons should compute a difference of the input they get from representation and prediction neurons. The preprint demonstrates that this is indeed the case in L2/3 of V1.
Key findings
The preprint shows that L2/3 pyramidal neurons have heterogeneous subthreshold membrane potential responses to visuomotor mismatch. Many of them have depolarizing mismatch responses (dMM), and some have hyperpolarizing mismatch responses (hMM). While these had been indicated by previous data with Ca2+ imaging3, this is the first demonstration of these subtypes with whole cell recordings. The method is key here because it reveals subthreshold membrane potential (Vm) responses arising from synaptic input. It also allowed the authors to report electrophysiological properties of these cells for the first time.
The authors then record from the same neurons with uncoupled visual flow and locomotion to find out their separate influences on the cells. These recordings demonstrate that the sensory and locomotion computation in most of these neurons are consistent with the predictive coding hypothesis: in dMM neurons Vm correlation with locomotion speed is positive (prediction input) while correlation with visual flow speed is negative (observation input), while the opposite is true of hMM neurons. This arrangement accounts for the mismatch responses of both cell types as a comparison of their sensory and locomotion responses, as expected for prediction error neurons. The dMM neurons had elevated resting membrane potential and spike threshold compared to hMM neurons, suggesting specialized electrophysiological ‘tuning’ to their functional roles.
The authors go on to record from L5/6 pyramidal neurons, showing that they have predominantly hyperpolarizing subthreshold mismatch responses. In other words, L5/6 neurons do not report prediction errors to downstream neurons. The L5/6 neurons have positive Vm correlations with locomotion and visual flow speed. So, instead of computing a prediction error response, they hyperpolarize either as a simple result of diminished visual input or through actively inhibitory input. This potential inhibition might arise from L5/6 interneurons that are excited by L2/3 dMM prediction error signals, as such connections can exist based on connectivity data4.
The authors end with a profound yet complex plot summarizing the differences between mismatch responses in L2/3 and L5/6 (their figure 6A,B, reprinted below). This key plot shows that mismatch responses in L2/3 are true prediction error responses because they arise from opposing signs of membrane potential correlation with locomotion speed vs visual flow speed. On the contrary, L5/6 hyperpolarizing mismatch responses are not prediction error responses because they arise from positive correlations of membrane potential with both locomotion and visual flow speeds (Figure 1).
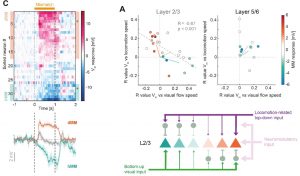
Why I chose this preprint
This study is important because it contains the first whole-cell recordings of mismatch responses from the Keller lab. In contrast to the previous Ca2+ imaging datasets, this new work shows subthreshold membrane potential responses in L2/3 neurons, as well as in L5/6 which has not been possible to reach with Ca2+ imaging. The findings show a good fit to what would be expected from prediction error computation in L2/3 pyramidal neurons. However, in L5/6 the neurons do not have the expected responses. Instead L5/6 pyramidal neurons fit the profile of representation neurons, where they would simply encode mouse speed through the world. Thus only L2/3 computes prediction errors. The work thereby demonstrates layer specific sensorimotor integration.
What next?
This work shows robust evidence for prediction error computation in V1 L2/3 and raises many interesting questions. Obviously the mechanisms generating the responses are of interest, and they are schematized by the authors in their Figure 6D. A previous study from the Keller lab concluded that somatostatin (SOM) and vasoactive intestinal peptide (VIP) interneurons are involved3. The delta oscillation seen in whole-cell traces during visual flow surely suggests involvement of a slowish excitatory/inhibitory oscillatory circuit somewhere in the mix. This could arise from burst firing of mutually inhibitory VIP and SOM interneuron populations. Top-down projections from the cingulate cortex are known to disinhibit V1 through primarily targeting VIP interneurons5. Previous work from the Keller lab indicates that this projection is also important for generating mismatch responses and has topographical organization on a gross level6. While it has not been quantified whether this projection is organized into finely clustered axonal fields in L1 of V1 (though Figure3A in Leinweber et al. 20176 suggests this may be the case), inputs from thalamus and secondary visual areas certainly are. Thalamic and secondary visual axons form an interleaved patchwork of ~60um diameter patches in V1, and these correspond to muscarinic receptor distributions and differences in interneuron circuitry7,8. Various other strands of evidence suggest existence of local interneuron teams at a similar spatial scale9. Might there be a topographical distribution of hyperpolarizing and depolarizing mismatch neurons associated with these patches?
How does V1 learn to generate these experience dependent responses?3 Are the responses ‘calibrated’ to match the visual flow conditions? For example, if a mouse were to move from the tall grass to arid plains, its visual flow might require retuning. For many eye movements, the cerebellum is thought to perform the role of a ‘teacher’, which is not required for the movement itself, but enables its tuning. Might loops between the cerebellum and prefrontal cortex, through thalamus and pontine nuclei10–12, also be involved in modifying V1 prediction error responses? It has been demonstrated that V1 SOM interneurons do not undergo marked plasticity while parvalbumin (PV) and neurogliaform neuropeptide Y (NPY) interneurons do1,3,13. PV and NPY interneurons inhibit SOM interneurons, pyramidal cells and VIP interneurons, which positions them as potential mediators of prediction error modifications.
How do V1 mismatch responses differ from those in other sensory cortices with similar circuitry, like somatosensory or auditory cortex? For example, is the hMM response in L5/6 somehow related to sensory suppression that occurs during saccades or self-generated sounds? The potential logic being that after reporting an observation, sensory representation should be suppressed during mismatch in order to prevent it from participating in further processing loops or modification.
Finally, how do the various mismatch neurons interact with each other? As the authors discuss, the predictive coding framework suggests the prediction error neurons and representative neurons would communicate to update predictions.1 As PV and NPY interneurons have strong, experience dependent mismatch responses3, perhaps dMMs drive them during mismatch causing hMM responses in L5/6. Would this inhibition be an update of the representation?
References:
- Keller, G. B. & Mrsic-Flogel, T. D. Predictive Processing: A Canonical Cortical Computation. Neuron 100, 424–435 (2018).
- Keller, G. B., Bonhoeffer, T. & Hübener, M. Sensorimotor mismatch signals in primary visual cortex of the behaving mouse. Neuron 74, 809–815 (2012).
- Attinger, A., Wang, B. & Keller, G. B. Visuomotor Coupling Shapes the Functional Development of Mouse Visual Cortex. Cell 169, 1291-1302.e14 (2017).
- Jiang, X. et al. Principles of connectivity among morphologically defined cell types in adult neocortex. Science 350, aac9462–aac9462 (2015).
- Zhang, S. et al. Selective attention. Long-range and local circuits for top-down modulation of visual cortex processing. Science 345, 660–5 (2014).
- Leinweber, M., Ward, D. R., Sobczak, J. M., Attinger, A. & Keller, G. B. A Sensorimotor Circuit in Mouse Cortex for Visual Flow Predictions. Neuron 95, 1420-1432.e5 (2017).
- D’Souza, R. D., Bista, P., Meier, A. M., Ji, W. & Burkhalter, A. Spatial Clustering of Inhibition in Mouse Primary Visual Cortex. Neuron 104, 588-600.e5 (2019).
- Ji, W. et al. Modularity in the Organization of Mouse Primary Visual Cortex. Neuron 87, 632–643 (2015).
- Karnani, M. M. & Jackson, J. Interneuron Cooperativity in Cortical Circuits. Neuroscientist 24, 329–341 (2018).
- Gao, Z. et al. A cortico-cerebellar loop for motor planning. Nature 563, 113–116 (2018).
- Chabrol, F., Blot, A. & Mrsic-Flogel, T. D. Cerebellar contribution to preparatory activity in motor neocortex. bioRxiv 335703 (2018) doi:10.1101/335703.
- Proville, R. D. et al. Cerebellum involvement in cortical sensorimotor circuits for the control of voluntary movements. Nat. Neurosci. 17, 1233–9 (2014).
- Xue, M., Atallah, B. V. & Scanziani, M. Equalizing excitation–inhibition ratios across visual cortical neurons. Nature 511, 596–600 (2014).
doi: https://doi.org/10.1242/prelights.18184
Read preprintSign up to customise the site to your preferences and to receive alerts
Register hereAlso in the neuroscience category:
Sexually dimorphic role of diet and stress on behavior, energy metabolism, and the ventromedial hypothalamus
Jimeng Li
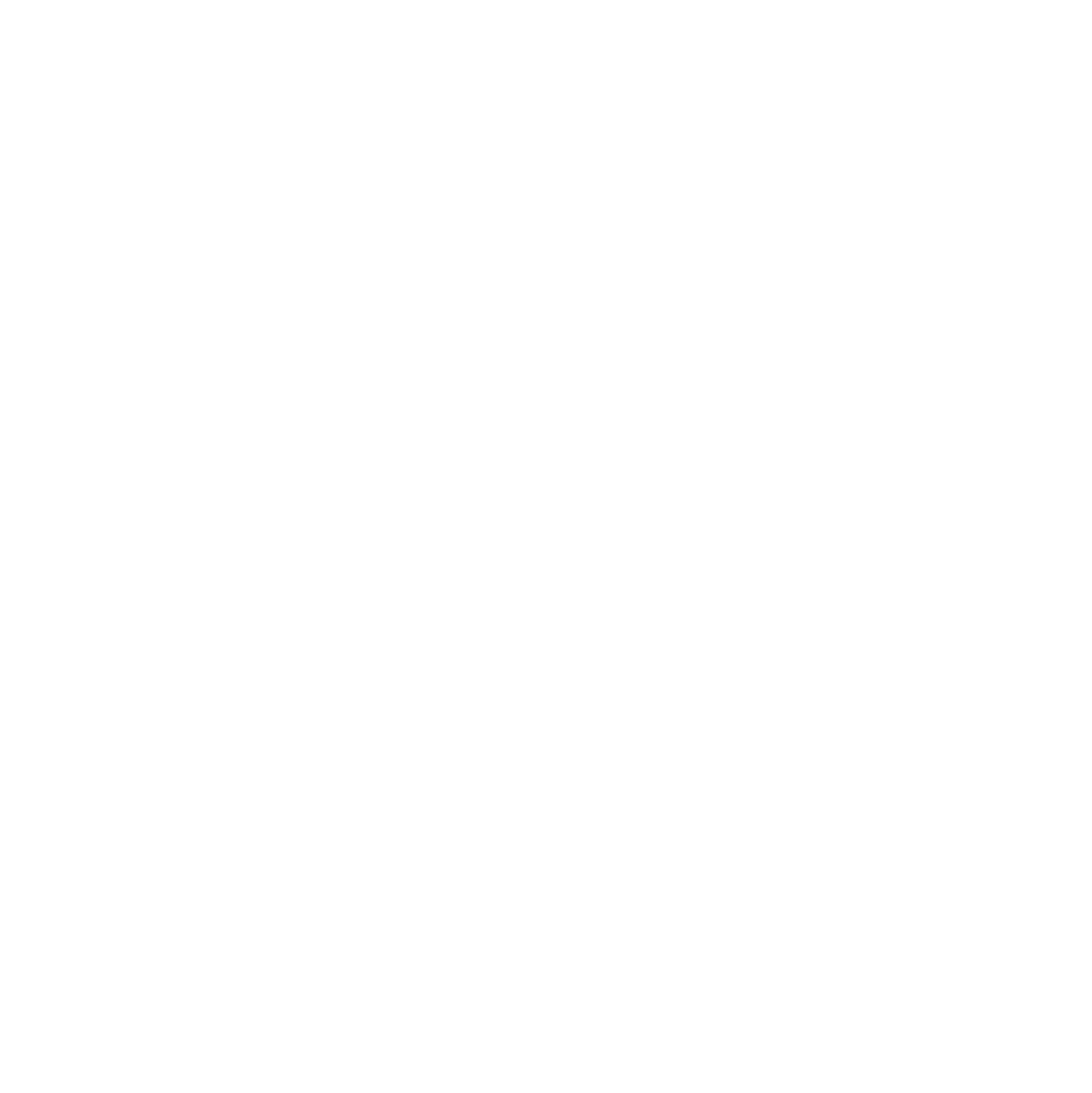
Enhancer-driven cell type comparison reveals similarities between the mammalian and bird pallium
Rodrigo Senovilla-Ganzo

Autism gene variants disrupt enteric neuron migration and cause gastrointestinal dysmotility
Rachel Mckeown
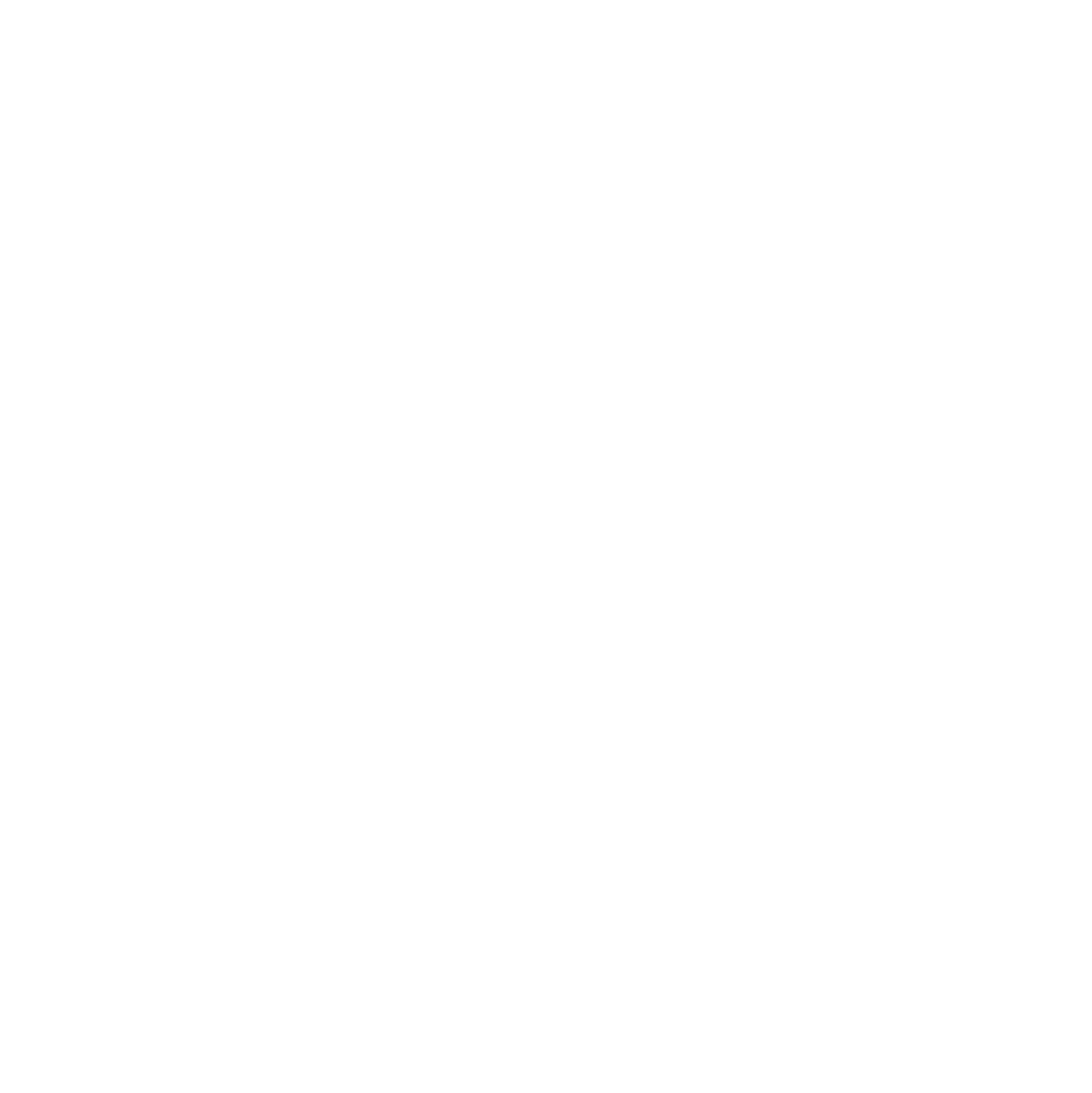
Also in the physiology category:
Gestational exposure to high heat-humidity conditions impairs mouse embryonic development
Girish Kale, preLights peer support

Modular control of time and space during vertebrate axis segmentation
AND
Natural genetic variation quantitatively regulates heart rate and dimension
Girish Kale, Jennifer Ann Black

Blue appendages and temperature acclimation increase survival during acute heat stress in the upside-down jellyfish, Cassiopea xamachana
Maitri Manjunath

preListsneuroscience category:
in the‘In preprints’ from Development 2022-2023
A list of the preprints featured in Development's 'In preprints' articles between 2022-2023
List by | Alex Eve, Katherine Brown |
CSHL 87th Symposium: Stem Cells
Preprints mentioned by speakers at the #CSHLsymp23
List by | Alex Eve |
Journal of Cell Science meeting ‘Imaging Cell Dynamics’
This preList highlights the preprints discussed at the JCS meeting 'Imaging Cell Dynamics'. The meeting was held from 14 - 17 May 2023 in Lisbon, Portugal and was organised by Erika Holzbaur, Jennifer Lippincott-Schwartz, Rob Parton and Michael Way.
List by | Helen Zenner |
FENS 2020
A collection of preprints presented during the virtual meeting of the Federation of European Neuroscience Societies (FENS) in 2020
List by | Ana Dorrego-Rivas |
ASCB EMBO Annual Meeting 2019
A collection of preprints presented at the 2019 ASCB EMBO Meeting in Washington, DC (December 7-11)
List by | Madhuja Samaddar et al. |
SDB 78th Annual Meeting 2019
A curation of the preprints presented at the SDB meeting in Boston, July 26-30 2019. The preList will be updated throughout the duration of the meeting.
List by | Alex Eve |
Autophagy
Preprints on autophagy and lysosomal degradation and its role in neurodegeneration and disease. Includes molecular mechanisms, upstream signalling and regulation as well as studies on pharmaceutical interventions to upregulate the process.
List by | Sandra Malmgren Hill |
Young Embryologist Network Conference 2019
Preprints presented at the Young Embryologist Network 2019 conference, 13 May, The Francis Crick Institute, London
List by | Alex Eve |
Also in the physiology category:
Fibroblasts
The advances in fibroblast biology preList explores the recent discoveries and preprints of the fibroblast world. Get ready to immerse yourself with this list created for fibroblasts aficionados and lovers, and beyond. Here, my goal is to include preprints of fibroblast biology, heterogeneity, fate, extracellular matrix, behavior, topography, single-cell atlases, spatial transcriptomics, and their matrix!
List by | Osvaldo Contreras |
FENS 2020
A collection of preprints presented during the virtual meeting of the Federation of European Neuroscience Societies (FENS) in 2020
List by | Ana Dorrego-Rivas |
TAGC 2020
Preprints recently presented at the virtual Allied Genetics Conference, April 22-26, 2020. #TAGC20
List by | Maiko Kitaoka et al. |
Autophagy
Preprints on autophagy and lysosomal degradation and its role in neurodegeneration and disease. Includes molecular mechanisms, upstream signalling and regulation as well as studies on pharmaceutical interventions to upregulate the process.
List by | Sandra Malmgren Hill |
Cellular metabolism
A curated list of preprints related to cellular metabolism at Biorxiv by Pablo Ranea Robles from the Prelights community. Special interest on lipid metabolism, peroxisomes and mitochondria.
List by | Pablo Ranea Robles |