Single-cell Map of Diverse Immune Phenotypes Driven by the Tumor Microenvironment
Posted on: 17 May 2018 , updated on: 5 July 2018
Preprint posted on 2 April 2018
Article now published in Cell at http://dx.doi.org/10.1016/j.cell.2018.05.060
Enormous details and variance of the immune cells present in breast tumors are normalized across patients
Selected by Tim FessendenCategories: cancer biology, genomics, immunology
Context:
Immunologists have long struggled against, or defended, their reliance on cell surface markers to study subsets of immune cells. Hiding behind cell surface markers, we fear, is great biological complexity that goes unseen. Similarly, gene expression studies on bulk populations often mask biologically relevant variation among individual cells. Thus a growing camp of immunologists have turned to single cell RNA sequencing (scRNA seq) to refine canonical immune cell subsets and measure gene expression changes particular to them (Tirosh et al). Yet with the ability to query individual cells comes the new challenge of clustering them into biologically meaningful populations, despite the huge variation within or between them. With a new kind of measurement must come new methods to normalize and compare the results they provide. This is especially the case for integrating data obtained from patients into clinically meaningful categories such as molecular subtypes or metastatic site from cancer patients.
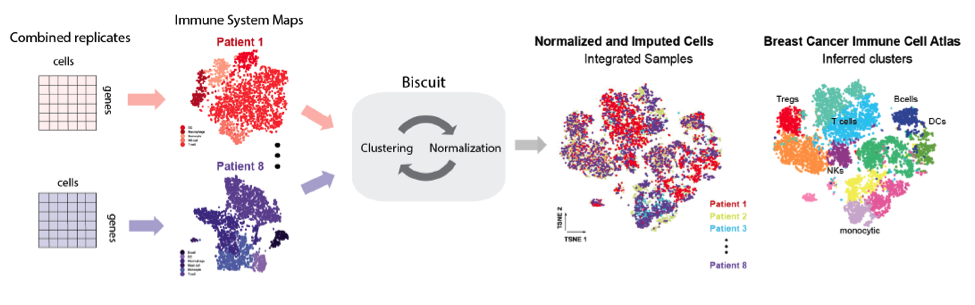
Data:
The authors obtained tissue from eight breast cancer patients representing different subtypes. For three of these patients the authors also obtained adjacent normal tissue, and from one patient they obtained a lymph node metastasis. They enriched for immune cells and sequenced via the InDrop platform, sequencing over 47,000 immune cells in aggregate. Following sequencing, their first pass analysis showed that immune cells exhibited significant patient-specific batch effects. A major advance put forth by this preprint addresses this challenge using BISCUIT, described in a prior paper, to remove such effects and normalize scRNA seq data across all eight patients, enabling comparisons among them (Prabhakaran et al).
Their most detailed and provocative observations concern tumor-resident T cells, which were not clustered into pools of distinct phenotypes such as naïve or activated. Individual cells rather were smeared along a continuum in which gene modules for these states were coexpressed and vary widely. This continuum was best captured along axes of activation, terminal differentiation and signatures of hypoxia. To capture the total variation among cells the authors described the “phenotypic volume,” i.e. the summed diversity of cell states, which was vastly expanded within tumors vs normal tissue. This observation of continuous cell states agrees with previous work published by the Pe’er group, showing continuous gene expression changes in response to competing cytokine inputs (Antebi and Reich-Zeliger, et al).
After posting these results, the authors later added data from T cell receptor (TCR) sequencing by using both InDrop and 10X platorms, to query TCR clones in three additional patients. Somewhat unsurprisingly, clonotypes clustered with restricted suites of cell states, rather than exhibiting the full panoply of T cell phenotypes. They argue, convincingly, that T cell clones likely respond uniquely to microenvironments of their antigen, yielding spatially distributed T cell phenotypes according to antigen distribution and microenvironmental cues.
Turning to cells of the myeloid lineage, the authors find a similar phenomenon in macrophages. Contrary to models espousing mutually exclusive M1 and M2 subsets, macrophages express M1 and M2-type gene modules simultaneously. Indeed, M1 and M2 genes were positively correlated in macrophages.
Implications:
In light of their observations, the authors argue for refined models of T cell activation and differentiation in malignant tissues that account for their apparent plasticity as they encounter aberrant environments. The notion that the local environment strongly determines T cell phenotypes predicts trouble for T cell therapies that use genetic modifications to hardwired cell activation, as these may not surmount microenvironmental effects within a target tissue. How accurately the signature for hypoxia actually reports on hypoxic environments is not assessed by this study. Yet the broader points – of continuous variation in T cell phenotype, trending with signatures for hypoxia – already presents a set of provocative and testable predictions.
With the new level of detail offered by scRNA seq, biologists promise (and are promised) to advance our understanding of immunology and, in this case, immunotherapy for cancer. However, these more detailed measurements threaten to outpace our ability to meaningfully analyze cell populations, and to apply these analyses across patients. Thus we might temper our enthusiasm for this vast increase in granularity.
Questions:
Does the correlation between hypoxia and differentiation gene expression signatures reflect a direct causative relationship?
Would normalizing hypoxic microenvironments necessarily change T cell functions?
How can we make comparisons between patients, or conclusions on patient cohorts, when our yardstick is high-dimensional datasets that encompass enormous variation?
References
Prabhakaran, S., Azizi, E., Carr, A., and Pe’er, D. (2016). Dirichlet Process Mixture Model for Correcting Technical Variation in Single-Cell Gene Expression Data. In Proceedings of The 33rd International Conference on Machine Learning, B. Maria Florina, and Q.W. Kilian, eds. (Proceedings of Machine Learning Research: PMLR), pp.1070–1079.
Tirosh, I., Izar, B., Prakadan, S.M., Wadsworth, M.H., 2nd, Treacy, D., Trombetta, J.J., Rotem, A., Rodman, C., Lian, C., Murphy, G., et al. (2016). Dissecting the multicellular ecosystem of metastatic melanoma by single-cell RNA-seq. Science 352, 189-196.
Yaron E. Antebi, Shlomit Reich-Zeliger, Yuval Hart , Avi Mayo , Inbal Eizenberg , Jacob Rimer , Prabhakar Putheti, Dana Pe’er, Nir Friedman. (2013). Mapping Differentiation under Mixed Culture Conditions Reveals a Tunable Continuum of T Cell Fates. PLoS Biol. 11(7): e1001616.
Read preprintSign up to customise the site to your preferences and to receive alerts
Register hereAlso in the cancer biology category:
Mitochondria-derived nuclear ATP surge protects against confinement-induced proliferation defects
Teodora Piskova

Spatial transcriptomics elucidates medulla niche supporting germinal center response in myasthenia gravis thymoma
Jessica Chevallier

Invasion of glioma cells through confined space requires membrane tension regulation and mechano-electrical coupling via Plexin-B2
Jade Chan

Also in the genomics category:
A fine kinetic balance of interactions directs transcription factor hubs to genes
Deevitha Balasubramanian
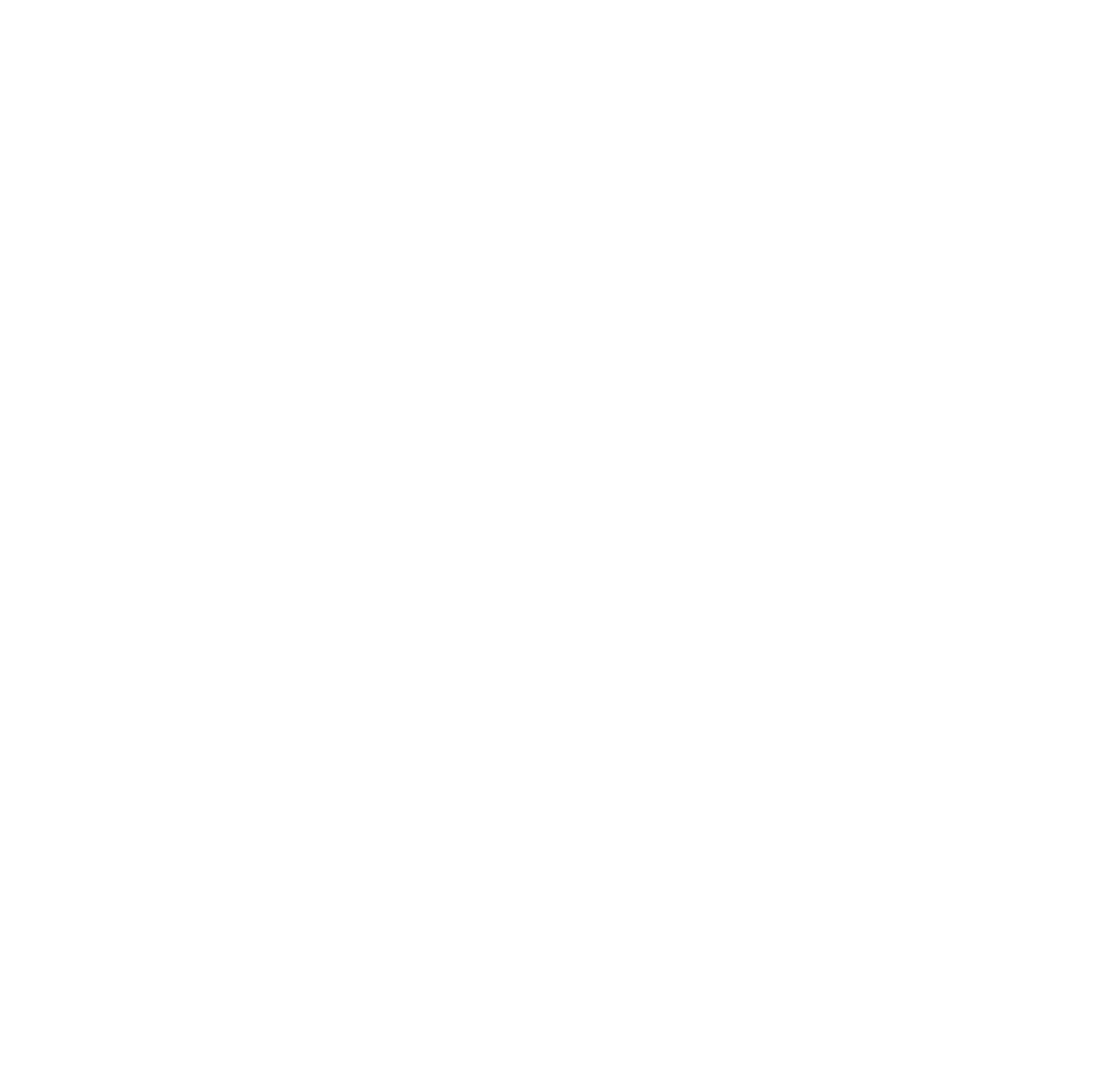
Enhancer-driven cell type comparison reveals similarities between the mammalian and bird pallium
Rodrigo Senovilla-Ganzo

Modular control of time and space during vertebrate axis segmentation
AND
Natural genetic variation quantitatively regulates heart rate and dimension
Girish Kale, Jennifer Ann Black

Also in the immunology category:
Control of Inflammatory Response by Tissue Microenvironment
Roberto Amadio

G6b-B antibody-based cis-acting platelet receptor inhibitors (CAPRIs) as a new family of anti-thrombotic therapeutics
Simon Cleary
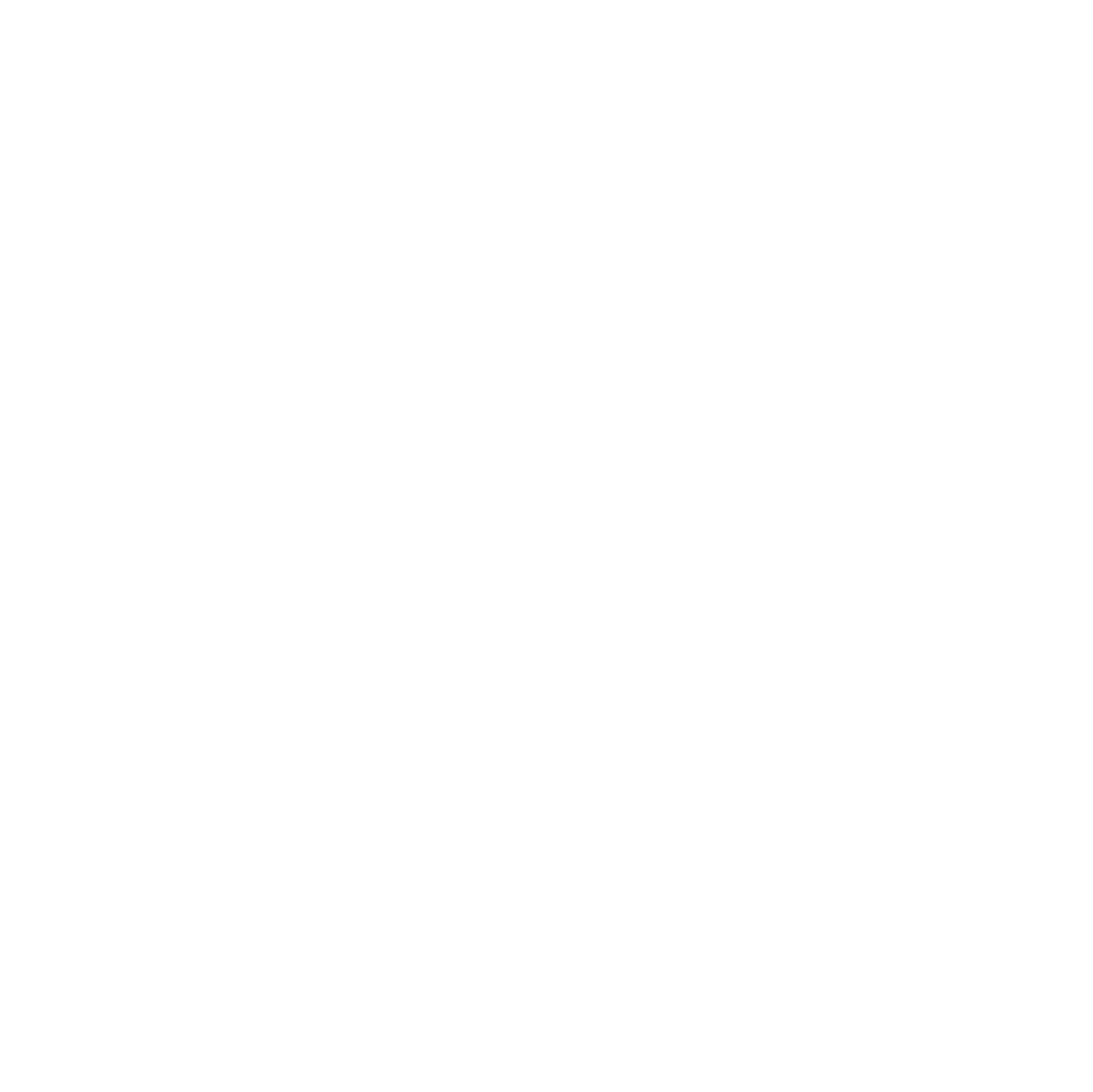
Feedback loop regulation between viperin and viral hemorrhagic septicemia virus through competing protein degradation pathways
UofA IMB565 et al.

preListscancer biology category:
in theBSCB-Biochemical Society 2024 Cell Migration meeting
This preList features preprints that were discussed and presented during the BSCB-Biochemical Society 2024 Cell Migration meeting in Birmingham, UK in April 2024. Kindly put together by Sara Morais da Silva, Reviews Editor at Journal of Cell Science.
List by | Reinier Prosee |
CSHL 87th Symposium: Stem Cells
Preprints mentioned by speakers at the #CSHLsymp23
List by | Alex Eve |
Journal of Cell Science meeting ‘Imaging Cell Dynamics’
This preList highlights the preprints discussed at the JCS meeting 'Imaging Cell Dynamics'. The meeting was held from 14 - 17 May 2023 in Lisbon, Portugal and was organised by Erika Holzbaur, Jennifer Lippincott-Schwartz, Rob Parton and Michael Way.
List by | Helen Zenner |
CellBio 2022 – An ASCB/EMBO Meeting
This preLists features preprints that were discussed and presented during the CellBio 2022 meeting in Washington, DC in December 2022.
List by | Nadja Hümpfer et al. |
Fibroblasts
The advances in fibroblast biology preList explores the recent discoveries and preprints of the fibroblast world. Get ready to immerse yourself with this list created for fibroblasts aficionados and lovers, and beyond. Here, my goal is to include preprints of fibroblast biology, heterogeneity, fate, extracellular matrix, behavior, topography, single-cell atlases, spatial transcriptomics, and their matrix!
List by | Osvaldo Contreras |
Single Cell Biology 2020
A list of preprints mentioned at the Wellcome Genome Campus Single Cell Biology 2020 meeting.
List by | Alex Eve |
ASCB EMBO Annual Meeting 2019
A collection of preprints presented at the 2019 ASCB EMBO Meeting in Washington, DC (December 7-11)
List by | Madhuja Samaddar et al. |
Lung Disease and Regeneration
This preprint list compiles highlights from the field of lung biology.
List by | Rob Hynds |
Anticancer agents: Discovery and clinical use
Preprints that describe the discovery of anticancer agents and their clinical use. Includes both small molecules and macromolecules like biologics.
List by | Zhang-He Goh |
Biophysical Society Annual Meeting 2019
Few of the preprints that were discussed in the recent BPS annual meeting at Baltimore, USA
List by | Joseph Jose Thottacherry |
Also in the genomics category:
BSCB-Biochemical Society 2024 Cell Migration meeting
This preList features preprints that were discussed and presented during the BSCB-Biochemical Society 2024 Cell Migration meeting in Birmingham, UK in April 2024. Kindly put together by Sara Morais da Silva, Reviews Editor at Journal of Cell Science.
List by | Reinier Prosee |
9th International Symposium on the Biology of Vertebrate Sex Determination
This preList contains preprints discussed during the 9th International Symposium on the Biology of Vertebrate Sex Determination. This conference was held in Kona, Hawaii from April 17th to 21st 2023.
List by | Martin Estermann |
Semmelweis Symposium 2022: 40th anniversary of international medical education at Semmelweis University
This preList contains preprints discussed during the 'Semmelweis Symposium 2022' (7-9 November), organised around the 40th anniversary of international medical education at Semmelweis University covering a wide range of topics.
List by | Nándor Lipták |
20th “Genetics Workshops in Hungary”, Szeged (25th, September)
In this annual conference, Hungarian geneticists, biochemists and biotechnologists presented their works. Link: http://group.szbk.u-szeged.hu/minikonf/archive/prg2021.pdf
List by | Nándor Lipták |
EMBL Conference: From functional genomics to systems biology
Preprints presented at the virtual EMBL conference "from functional genomics and systems biology", 16-19 November 2020
List by | Jesus Victorino |
TAGC 2020
Preprints recently presented at the virtual Allied Genetics Conference, April 22-26, 2020. #TAGC20
List by | Maiko Kitaoka et al. |
Zebrafish immunology
A compilation of cutting-edge research that uses the zebrafish as a model system to elucidate novel immunological mechanisms in health and disease.
List by | Shikha Nayar |
Also in the immunology category:
Journal of Cell Science meeting ‘Imaging Cell Dynamics’
This preList highlights the preprints discussed at the JCS meeting 'Imaging Cell Dynamics'. The meeting was held from 14 - 17 May 2023 in Lisbon, Portugal and was organised by Erika Holzbaur, Jennifer Lippincott-Schwartz, Rob Parton and Michael Way.
List by | Helen Zenner |
Fibroblasts
The advances in fibroblast biology preList explores the recent discoveries and preprints of the fibroblast world. Get ready to immerse yourself with this list created for fibroblasts aficionados and lovers, and beyond. Here, my goal is to include preprints of fibroblast biology, heterogeneity, fate, extracellular matrix, behavior, topography, single-cell atlases, spatial transcriptomics, and their matrix!
List by | Osvaldo Contreras |
Single Cell Biology 2020
A list of preprints mentioned at the Wellcome Genome Campus Single Cell Biology 2020 meeting.
List by | Alex Eve |
Autophagy
Preprints on autophagy and lysosomal degradation and its role in neurodegeneration and disease. Includes molecular mechanisms, upstream signalling and regulation as well as studies on pharmaceutical interventions to upregulate the process.
List by | Sandra Malmgren Hill |
Antimicrobials: Discovery, clinical use, and development of resistance
Preprints that describe the discovery of new antimicrobials and any improvements made regarding their clinical use. Includes preprints that detail the factors affecting antimicrobial selection and the development of antimicrobial resistance.
List by | Zhang-He Goh |