The geometry of abstraction in hippocampus and prefrontal cortex
Posted on: 27 February 2020 , updated on: 15 January 2021
Preprint posted on 4 October 2019
Article now published in Cell at http://dx.doi.org/10.1016/j.cell.2020.09.031
Neural representations of task-relevant variables are encoded in an abstract format that promotes generalization and cognitive flexibility.
Selected by Cody WaltersCategories: neuroscience
Background
The ability to rapidly adapt to novel environments (e.g., starting a new job or navigating a city you have never visited before) requires the use of information about the underlying structure of past experiences that generalize to the current situation. In complex (i.e., high-dimensional) environments, it is not always clear which features are behaviorally relevant and which features can safely be ignored. This can make it challenging to associate the outcome of an action (e.g., reward or punishment) with a feature or set of features that predict that outcome owing to the many potential causal variables. This phenomenon is known as the curse of dimensionality and is related to the credit assignment problem (i.e., how to assign the value of an outcome to the feature(s) that caused that outcome). If, however, there are a small subset of variables that underlie superficially disparate tasks, then we can leverage that shared structure (e.g., a schema for, say, going to the airport) to reduce the dimensionality of the feature space, thus achieving generalization (i.e., we can develop a sufficiently general algorithm for commuting to the airport, getting through security, and boarding the plane that works in almost every country and context despite there being countless non-essential variables that differ within each individual trip). It remains an open question how the brain might represent task variables in such an abstract format.
Key Findings
Bernardi et al. trained two male rhesus monkeys in a reversal learning task. The monkeys were taught to hold down a button and view one of four visual cues (i.e., fractal images), with each cue being associated with an operant response (i.e., continue holding the button or release the button). For two of the four fractal images, the correct response resulted in liquid reward. For the other two fractal images, the correct response did not result in reward, but instead allowed the animal to progress to the next trial.
The task involved two contingency blocks (or contexts). The correct response associated with one of the fractal images in context 1 (e.g., release the button) was reversed in context 2 (e.g., do not release the button). Likewise, one of the fractal images that was rewarded in context 1 was no longer rewarded in context 2, and one of the fractal images that was unrewarded in context 1 was now rewarded in context 2.
While the monkeys performed this task, Bernardi et al. used individually drivable electrode arrays with 16 contact sites to record from anterior cingulate cortex (ACC) and dorsolateral prefrontal cortex (DLPFC) and 24 contact sites to record from the hippocampus (HPC). Using these data, they trained a linear classifier on a subset of conditions (e.g., two rewarded fractal images, one from each context) then tested the decoding accuracy using a new subset of conditions (e.g., two unrewarded fractal images, one from each context). If, for example, context is being represented, the line (or plane in three-dimensions, or hyperplane in higher dimensions) that separates the two training points (one from each context) will be similar to the line that separates the two test points (i.e., the high-dimensional neural representations for conditions in the same context are clustered).
Two metrics were used to quantify population activity: the cross-condition generalization performance (CCGP) and the parallelism score (PS). The extent to which the classifier, trained on one subset of conditions, generalized to a new subset of conditions was captured by the CCGP, and the difference in the angle of the separating hyperplane for the training set and the test set was quantified as the parallelism score (with the CCGP and PS being, for the most part, positively correlated).
The authors looked at two time points: a 900ms epoch prior to image onset (to investigate the extent to which information about task-relevant variables are represented during the inter-stimulus interval) and a 900ms epoch 100ms after image onset. In both the pre- and post-image onset epochs, they found that most variables of interest could be decoded above chance, but few variables were abstractly represented (as measured by the CCGP and PS). Indeed, the three variables that were the most abstractly represented were context (in all three brain areas), the value (i.e., rewarded or unrewarded) on the previous trial (in all three brain areas), and the action from the previous trial (in ACC and DLPFC but not in HPC). Interestingly, in the post-image onset epoch (relative to the pre-image onset epoch) they found that context representation decreased significantly in ACC and DLPFC while action representation (for the current trial) increased in HPC.
These results suggest that 1) certain task variables such as context, value, and action are represented in an abstract format (as measured by the CCGP and PS) and 2) that the geometry of this high-dimensional neural firing-rate space is not static but rather dynamically shifts when the animal has access to information (i.e., the visual stimulus) that informs an upcoming action.
Future directions/questions for the authors
Q1) In the example given in the manuscript of training the classifier on one subset of conditions (one condition from each context) then testing it on a new subset of conditions (also one condition from each context), how do you dissociate context from time? That is, given that the visual cues within a context occur in the same block of time, how do you ensure that context and not time is being represented?
Q2) Using a linear classifier implicitly assumes that the brain is conducting some form of linear decoding. While this is likely true in many cases and analytically serves as a valuable starting point, do you think there are scenarios where a non-linear classifier might successfully model aspects of cognition and behavior that a linear classifier would fail to capture?
doi: https://doi.org/10.1242/prelights.17378
Read preprintSign up to customise the site to your preferences and to receive alerts
Register hereAlso in the neuroscience category:
Evolution of taste processing shifts dietary preference
T. W. Schwanitz

A zebrafish circuit for behavioral credit assignment
Muhammed Sinan Malik
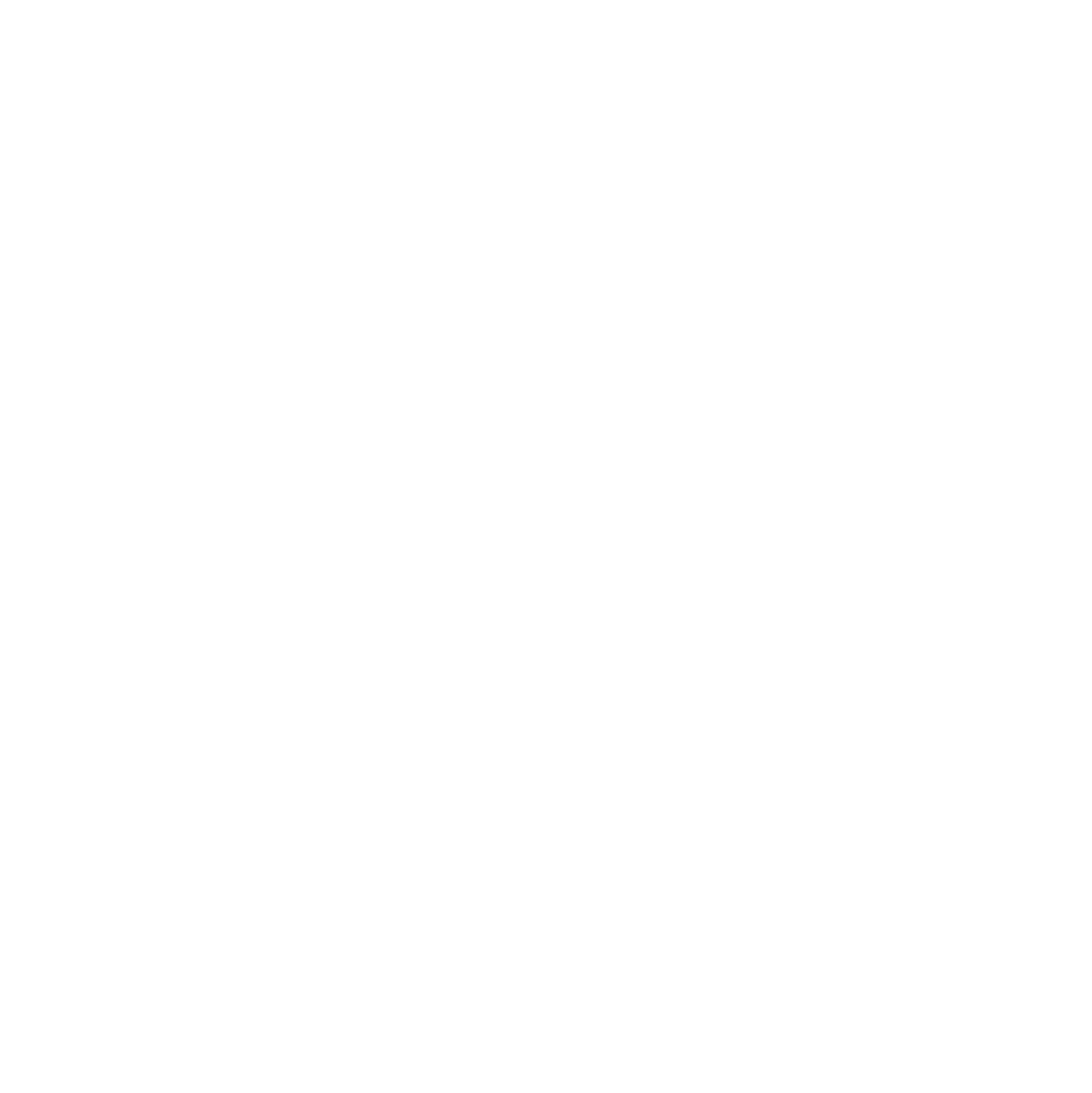
Fis1 is required for the development of the dendritic mitochondrial network in pyramidal cortical neurons
Felipe Del Valle Batalla

preLists in the neuroscience category:
Biologists @ 100 conference preList
This preList aims to capture all preprints being discussed at the Biologists @100 conference in Liverpool, UK, either as part of the poster sessions or the (flash/short/full-length) talks.
List by | Jonathan Townson, Jonathan Townson |
2024 Hypothalamus GRC
This 2024 Hypothalamus GRC (Gordon Research Conference) preList offers an overview of cutting-edge research focused on the hypothalamus, a critical brain region involved in regulating homeostasis, behavior, and neuroendocrine functions. The studies included cover a range of topics, including neural circuits, molecular mechanisms, and the role of the hypothalamus in health and disease. This collection highlights some of the latest advances in understanding hypothalamic function, with potential implications for treating disorders such as obesity, stress, and metabolic diseases.
List by | Nathalie Krauth |
‘In preprints’ from Development 2022-2023
A list of the preprints featured in Development's 'In preprints' articles between 2022-2023
List by | Alex Eve, Katherine Brown |
CSHL 87th Symposium: Stem Cells
Preprints mentioned by speakers at the #CSHLsymp23
List by | Alex Eve |
Journal of Cell Science meeting ‘Imaging Cell Dynamics’
This preList highlights the preprints discussed at the JCS meeting 'Imaging Cell Dynamics'. The meeting was held from 14 - 17 May 2023 in Lisbon, Portugal and was organised by Erika Holzbaur, Jennifer Lippincott-Schwartz, Rob Parton and Michael Way.
List by | Helen Zenner |
FENS 2020
A collection of preprints presented during the virtual meeting of the Federation of European Neuroscience Societies (FENS) in 2020
List by | Ana Dorrego-Rivas |
ASCB EMBO Annual Meeting 2019
A collection of preprints presented at the 2019 ASCB EMBO Meeting in Washington, DC (December 7-11)
List by | Madhuja Samaddar et al. |
SDB 78th Annual Meeting 2019
A curation of the preprints presented at the SDB meeting in Boston, July 26-30 2019. The preList will be updated throughout the duration of the meeting.
List by | Alex Eve |
Autophagy
Preprints on autophagy and lysosomal degradation and its role in neurodegeneration and disease. Includes molecular mechanisms, upstream signalling and regulation as well as studies on pharmaceutical interventions to upregulate the process.
List by | Sandra Malmgren Hill |
Young Embryologist Network Conference 2019
Preprints presented at the Young Embryologist Network 2019 conference, 13 May, The Francis Crick Institute, London
List by | Alex Eve |