Charting a tissue from single-cell transcriptomes
Posted on: 24 January 2019
Preprint posted on 30 October 2018
Nitzan et al.'s exciting new approach promises to resuscitate the spatial context of cells from scRNAseq without marker genes
Selected by Irepan Salvador-MartinezCategories: bioinformatics, genomics, systems biology
Introduction
The field of developmental genetics has been largely built on the description of spatiotemporal patterns of gene expression during development. In the mid-80’s, in situ hybridization (ISH) using digoxygenin-labelled DNA probes was developed, which allowed the localisation of RNA products in whole mount Drosophila embryos. Papers describing spatial gene expression patterns came one after another in the following years. The big disadvantage of the ISH technique is that only 1 or few RNAs can be assessed at the same time. To overcome this limitation, large-scale ISH assays and/or great community efforts have generated databases with thousands of in situ images for a few model organisms (e.g., FlyExpress in Drosophila). However, a precise inference of gene co-expression is not possible given the staining variability between ISH experiments or biological variability of gene expression between organisms.
In parallel, DNA sequencing technology has improved rapidly in recent years, both in terms of cost and the amount of biological sample required for analysis, so now it is possible to sequence the genomic DNA or the transcriptome of a single cell. This had led to an explosion in single-cell sequencing technology, currently allowing for sequencing of >100,000 cells. scRNAseq has allowed the identification of previously unknown rare cell types and has provided a clearer picture of the gene expression differences underlying cell type differentiation. The drawback of scRNAseq is that the spatial context of the cells is lost, due to the required cell dissociation. This means that by using scRNAseq you might get to know the gene expression profiles of every single cell in a tissue/embryo, but lack information about where the cells came from in the original tissue.
The work from Nitzan et al. tries to fill this gap of scRNAseq techniques by resuscitating the spatial context of the cells. The idea is straightforward: if we know the gene expression of every single cell in a tissue, can we reconstruct its spatial organisation? Last year, Karaiskos et al., demonstrated that it was possible to use scRNAseq data of the Drosophila blastoderm to reconstruct the spatial distribution of the cells at single cell resolution[1]. To do this, they used as a reference the previously known spatial distribution of only 84 genes (binarised as ON/OFF), that were mapped onto a 2D “virtual embryo” of ~3000 bins (each bin can be thought of as a “virtual cell”). Then, gene expression data of single cells (coming from scRNAseq) was also binarised and a correlation coefficient for each cell/bin pair was calculated. Finally, every sequenced cell was assigned to one (or multiple) bins in the virtual embryo. Karaiskos et al. could not only reproduce known gene expression patterns (as revealed previously by ISH), but they could also predict the unknown expression pattern of some transcription factors and long noncoding RNAs.
The preprint
The main novelty of Nitzan et al.’s approach is that they propose a de novo reconstruction of gene expression spatial patterns, i.e., without the need of a reference map of gene expression based on marker genes. Instead, their mapping (which they name novoSpaRc) is based solely on the sequencing data and geometric features of the tissue/embryo to be reconstructed (see Figure 1). To do this, they use a well known mathematical framework called “optimal transport problem” which was originally motivated by a seemingly simple problem, how can I rearrange a pile of dirt from a monticle to another configuration by expending the minimal amount of energy?
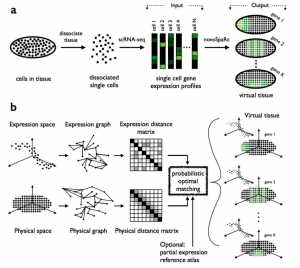
In this specific application of the optimal transport problem, the aim is to find a correspondence between two groups: the gene expression profiles coming from scRNAseq (“cells”) and the cellular locations of the tissue to be reconstructed (“positions”). They start by calculating two different parwise distance matrices: 1) a “physical distance matrix” based on the Euclidean distance between “positions” and 2) a “expression distance matrix” based on a correlation-based distance between “cells”. Then, the optimal transport problem framework is used to find a probabilistic embedding of the “cells” to “positions” that minimises the discrepancy between the distances in expression and physical space (Figure 1).
Nitzan et al. used various datasets to test their approach, including Karaiskos et al. data. They demonstrated that a de novo reconstruction of the embryo (without using marker genes) successfully separated major gene expression spatial domains (i.e., endoderm and mesoderm) and largely reconstructed gene expression patterns across the dorso-ventral and anterio-posterior axes. Amazingly, by using information of only 4 marker genes (instead of 84 genes used by Karaiskos et al.) they could reconstruct almost perfectly original fluorescence ISH data (Figure 2).
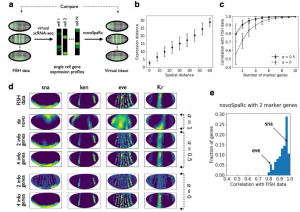
Future work
This approach is based on the strong assumption that gene expression between nearby cells is more similar than gene expression between cells which are separated by larger distances. Nitzan et al., used mostly pre-gastrulation gene expression data to test their approach, which is an ideal dataset due to the low spatial gene expression differentiation at these stages and because the gene expression patterns are essentially 2D. It still remains unclear if this approach would be applicable to more complex gene expression datasets (e.g., at later developmental stages or 3D data). Nevertheless, this work provides an exciting proof of principle and will carve the way for studies in the near future.
Questions for the authors:
- Could this framework be used to reconstruct more complex gene expression patterns (i.e., where the assumption that genetic expression and spatial distance are correlated is not met)?
- Could this be extended to 3D spatial coordinates?
References
[1] Karaiskos, N. et al. The Drosophila embryo at single-cell transcriptome resolution. Science 358, 194-199 (2017).
doi: https://doi.org/10.1242/prelights.7753
Read preprintSign up to customise the site to your preferences and to receive alerts
Register hereAlso in the bioinformatics category:
Enhancer-driven cell type comparison reveals similarities between the mammalian and bird pallium
Rodrigo Senovilla-Ganzo

Expressive modeling and fast simulation for dynamic compartments
Benjamin Dominik Maier

Transcriptional profiling of human brain cortex identifies novel lncRNA-mediated networks dysregulated in amyotrophic lateral sclerosis
Julio Molina Pineda
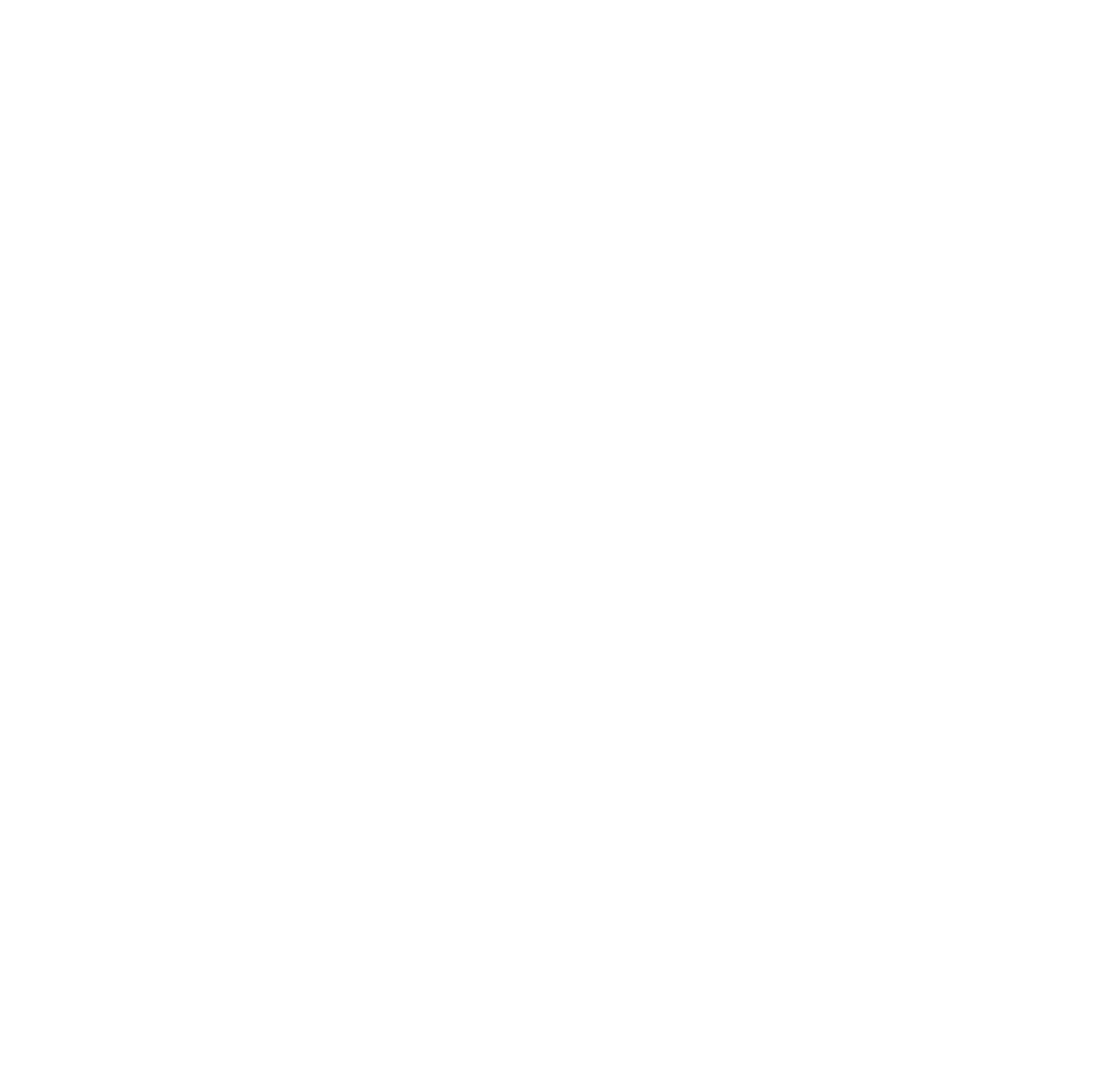
Also in the genomics category:
A fine kinetic balance of interactions directs transcription factor hubs to genes
Deevitha Balasubramanian
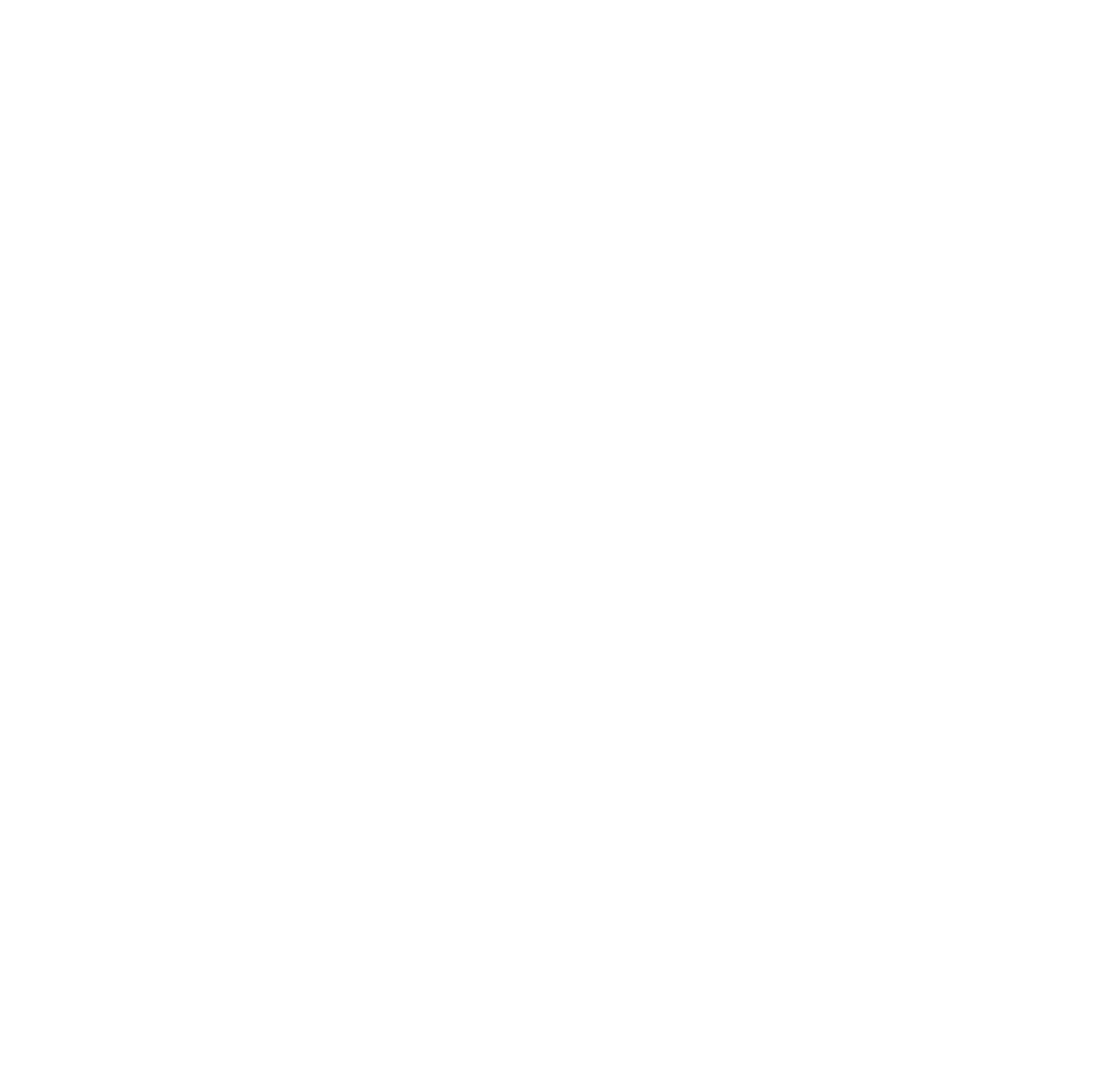
Enhancer-driven cell type comparison reveals similarities between the mammalian and bird pallium
Rodrigo Senovilla-Ganzo

Modular control of time and space during vertebrate axis segmentation
AND
Natural genetic variation quantitatively regulates heart rate and dimension
Girish Kale, Jennifer Ann Black

Also in the systems biology category:
Expressive modeling and fast simulation for dynamic compartments
Benjamin Dominik Maier

Clusters of lineage-specific genes are anchored by ZNF274 in repressive perinucleolar compartments
Silvia Carvalho

Holimap: an accurate and efficient method for solving stochastic gene network dynamics
Benjamin Dominik Maier

preListsbioinformatics category:
in the‘In preprints’ from Development 2022-2023
A list of the preprints featured in Development's 'In preprints' articles between 2022-2023
List by | Alex Eve, Katherine Brown |
9th International Symposium on the Biology of Vertebrate Sex Determination
This preList contains preprints discussed during the 9th International Symposium on the Biology of Vertebrate Sex Determination. This conference was held in Kona, Hawaii from April 17th to 21st 2023.
List by | Martin Estermann |
Alumni picks – preLights 5th Birthday
This preList contains preprints that were picked and highlighted by preLights Alumni - an initiative that was set up to mark preLights 5th birthday. More entries will follow throughout February and March 2023.
List by | Sergio Menchero et al. |
Fibroblasts
The advances in fibroblast biology preList explores the recent discoveries and preprints of the fibroblast world. Get ready to immerse yourself with this list created for fibroblasts aficionados and lovers, and beyond. Here, my goal is to include preprints of fibroblast biology, heterogeneity, fate, extracellular matrix, behavior, topography, single-cell atlases, spatial transcriptomics, and their matrix!
List by | Osvaldo Contreras |
Single Cell Biology 2020
A list of preprints mentioned at the Wellcome Genome Campus Single Cell Biology 2020 meeting.
List by | Alex Eve |
Antimicrobials: Discovery, clinical use, and development of resistance
Preprints that describe the discovery of new antimicrobials and any improvements made regarding their clinical use. Includes preprints that detail the factors affecting antimicrobial selection and the development of antimicrobial resistance.
List by | Zhang-He Goh |
Also in the genomics category:
BSCB-Biochemical Society 2024 Cell Migration meeting
This preList features preprints that were discussed and presented during the BSCB-Biochemical Society 2024 Cell Migration meeting in Birmingham, UK in April 2024. Kindly put together by Sara Morais da Silva, Reviews Editor at Journal of Cell Science.
List by | Reinier Prosee |
9th International Symposium on the Biology of Vertebrate Sex Determination
This preList contains preprints discussed during the 9th International Symposium on the Biology of Vertebrate Sex Determination. This conference was held in Kona, Hawaii from April 17th to 21st 2023.
List by | Martin Estermann |
Semmelweis Symposium 2022: 40th anniversary of international medical education at Semmelweis University
This preList contains preprints discussed during the 'Semmelweis Symposium 2022' (7-9 November), organised around the 40th anniversary of international medical education at Semmelweis University covering a wide range of topics.
List by | Nándor Lipták |
20th “Genetics Workshops in Hungary”, Szeged (25th, September)
In this annual conference, Hungarian geneticists, biochemists and biotechnologists presented their works. Link: http://group.szbk.u-szeged.hu/minikonf/archive/prg2021.pdf
List by | Nándor Lipták |
EMBL Conference: From functional genomics to systems biology
Preprints presented at the virtual EMBL conference "from functional genomics and systems biology", 16-19 November 2020
List by | Jesus Victorino |
TAGC 2020
Preprints recently presented at the virtual Allied Genetics Conference, April 22-26, 2020. #TAGC20
List by | Maiko Kitaoka et al. |
Zebrafish immunology
A compilation of cutting-edge research that uses the zebrafish as a model system to elucidate novel immunological mechanisms in health and disease.
List by | Shikha Nayar |
Also in the systems biology category:
‘In preprints’ from Development 2022-2023
A list of the preprints featured in Development's 'In preprints' articles between 2022-2023
List by | Alex Eve, Katherine Brown |
EMBL Synthetic Morphogenesis: From Gene Circuits to Tissue Architecture (2021)
A list of preprints mentioned at the #EESmorphoG virtual meeting in 2021.
List by | Alex Eve |
Single Cell Biology 2020
A list of preprints mentioned at the Wellcome Genome Campus Single Cell Biology 2020 meeting.
List by | Alex Eve |
ASCB EMBO Annual Meeting 2019
A collection of preprints presented at the 2019 ASCB EMBO Meeting in Washington, DC (December 7-11)
List by | Madhuja Samaddar et al. |
EMBL Seeing is Believing – Imaging the Molecular Processes of Life
Preprints discussed at the 2019 edition of Seeing is Believing, at EMBL Heidelberg from the 9th-12th October 2019
List by | Dey Lab |
Pattern formation during development
The aim of this preList is to integrate results about the mechanisms that govern patterning during development, from genes implicated in the processes to theoritical models of pattern formation in nature.
List by | Alexa Sadier |