Genome-wide molecular recording using Live-seq
Posted on: 26 April 2021 , updated on: 30 April 2021
Preprint posted on 24 March 2021
Live-seq: The Deplancke and Vorholt lab analyse the transcriptomes of live cells by directly sampling RNA from cell cytosols.
Selected by Irepan Salvador-Martinez, Samantha Seah, Tamara Sternlieb, Nozomu TakataCategories: molecular biology
Introduction
In recent years, single-cell RNA seq (scRNAseq) technologies have transformed biology. The possibility of dissociating a tissue or even a whole animal and elucidating the genes expressed by each cell has not only allowed the characterisation of known cell types, but also enabled the discovery of new cell types/states.
In 2018, La Manno et al [1] showed that RNA velocity could be used in scRNAseq studies to predict the future state of individual cells over a timescale of hours. RNA velocity is the time derivative of the gene expression state, determined by the relative abundance of unspliced and spliced mRNA. Since then, RNA velocity has become a common practice in cell differentiation scRNAseq studies. Other trajectory inference methods (e.g. pseudotime analysis) infer the order of sampled cells along dynamic differentiation trajectories (cell cycle, developmental processes) based on similarities in gene expression [2].
These inference methods attempt to circumvent an obvious and apparently unavoidable limitation of scRNAseq studies: that only end-point measurements are feasible as cells do not survive the process. This limitation has now been finally overcome by Live-seq, a new approach that allows transcriptomic profiling of living cells using fluidic force microscopy (FluidFM) to carry out cytoplasmic extraction at a picoliter scale, in a recent preprint presented by Chen et al.
Live-seq Method
Live-seq uses FluidFM to sample a small volume of cytoplasm from single cells coupled with a low-input RNA-seq strategy. This permits it to function as a transcriptomic recorder, where “past” transcriptomes can be linked with subsequent transcriptomes or cellular phenotypes. FluidFM combines atomic force microscopy with microscopic channels that can be used to handle femtoliter-scale volumes [3].
Buffer containing RNase inhibitors is first loaded into the FluidFM probe, before specific cells are targeted and a small volume of cytoplasm (an average of 1.1pL) is extracted. The extract is then released into a microliter droplet and the probe is washed to prevent cross-contamination, before another cell is sampled.
A viability assay showed that 85-89% of cells survived the extraction of 1.1 pL of cytoplasm. The cells could even divide a few hours post-sampling without any significant cellular perturbations, in a similar fashion to non-extracted cells. Given the low RNA input, the authors optimised the downstream RNA processing and improved the efficiency of the Smart-seq2 workflow, to minimise RNA degradation and maximise output. In addition, they implemented image-based cell tracking to permit sequential RNA sampling from the same cells (sequential Live-seq).
Proof of principle (Macrophage study)
As a proof of principle, the authors first used Live-seq to analyse different cell types: IBA cells, primary mouse adipose stem and progenitor cells (ASPCs) and monocyte/macrophage-like RAW cells. RAW cells were also activated with lipopolysaccharides (LPS) or treated with PBS as a control. Simultaneously, the response to LPS, as indicated by Tnf-mCherry expression, was monitored with fluorescence microscopy.
The 175 samples that passed their quality filtering criteria clustered into four distinct clusters in a t-SNE plot, clearly indicating a separation of cell types and states (Figure 1 left).
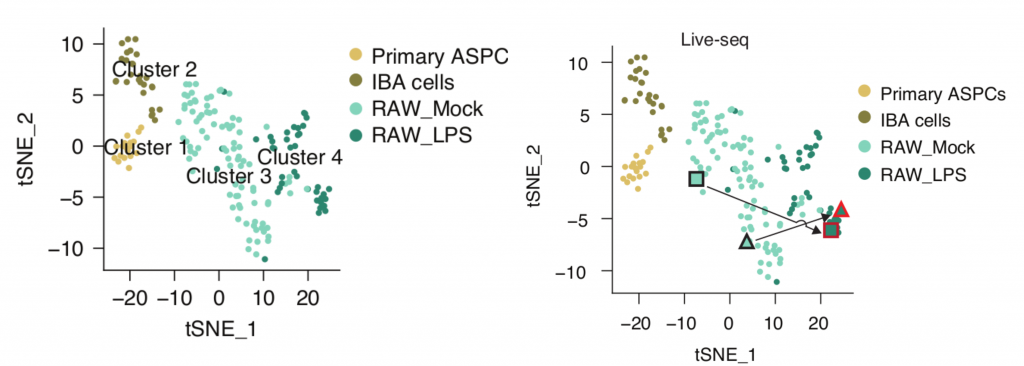
The question remained as to how well Live-seq captures molecular information in comparison with existing methods. To reveal this, they compared Live-seq and conventional scRNA-seq data, integrating these data in the same t-SNE plots. The result showed Live-seq gives similar results to conventional scRNAseq without the need to lyse or fix cells. The authors illustrated that multiple RNAseq data (Live-seq + typical scRNA-seq) can be displayed together as shown in Figure 2, reflecting the same clustering for all cell types and states.
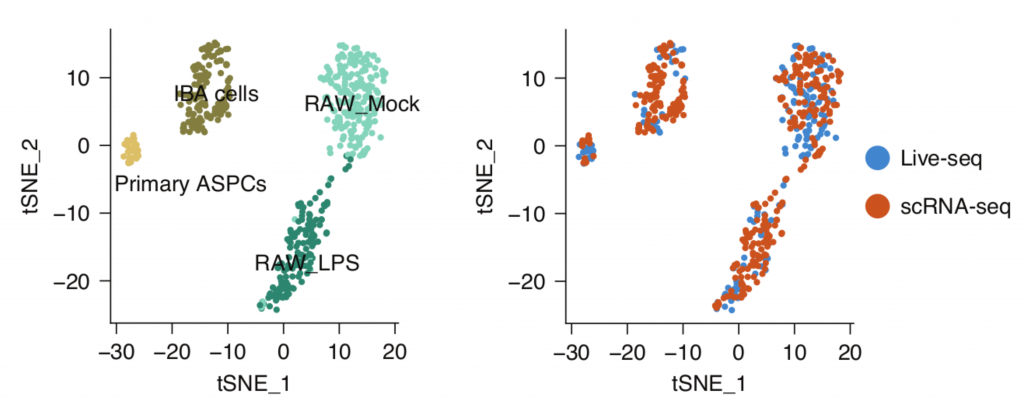
Subsequently, Live-seq was used to investigate the molecular factors that drive heterogeneity in macrophage responses to LPS. RAW cell transcriptomes were profiled prior to LPS activation, with Tnf-mCherry expression utilised as the phenotypic readout. This enabled the identification of various genes, most notably Nfkbia, that drive the heterogeneity in macrophage LPS responses, illustrating how Live-seq can be used for transcriptomic studies prior to phenotypic changes.
Finally, to record a cell’s molecular signature before and after cell state transitioning, the authors carried out Live-seq on RAW cells both prior to and after LPS stimulation. These sequential measurements yielded two distinct points in the same t-SNE map , clearly showing the transition of each cell from a pre-treatment (Mock) to a post-treatment (LPS) state (Figure 1b). This demonstrates that sequential Live-seq can capture the transitional change at a transcriptomic level, making it possible to observe the trajectory of single cells for the first time.
While inference-based methods may potentially create several spurious connections between cell states, Live-seq allows the acquisition of transcriptomic dynamics from the same cell and thus enables a direct readout of cell state trajectories.
How we think this moves the field forward
We believe that Live-seq is an outstanding technological innovation that will revolutionise the field of single-cell transcriptomics, as it overcomes one of the key limitations of conventional scRNA-seq. Namely, it provides the possibility of sampling the same cells across multiple timepoints and to directly observe cell state trajectories, as opposed to conventional scRNA-seq methods, which utilise end-point measurements where cell trajectories can only be inferred.
The seamless integration of Live-seq with conventional scRNAseq and other phenotypic readouts will be useful to assess how population heterogeneity affects the cells’ response after an external stimulus or over time, as cleverly shown in the proof of principle study involving macrophage cells.
This groundbreaking study comes from the Laboratory of Systems Biology and Genetics, also known as the Deplancke lab ( link to Deplancke lab web page), which has an excellent track record of papers relevant to gene regulation. This new method can be applied to further investigate the molecular histories of various cell types, as it is critical to understand a cell’s current state and the path through which it arrived at that state, to fully comprehend cellular responses and development in all its diversity. We are very excited to see how this technology will advance the field of single-cell transcriptomics.
Questions for the authors
- How accessible is the technology for other labs (e.g. is there a lot of technical expertise or equipment required?)
- Have the genes discovered to be involved with LPS stimulation been validated with knockdowns, knockouts or overexpression?
- Would it be possible to use Live-seq in the study of cells before and after the introduction of macromolecules like CRISPR/Cas9?
- Could dynamic transcriptomic changes during cell death be studied? We assume that sampling dying cells is probably challenging.
- Do you think there could be a way to adapt this method to apply it to cells in suspension?
References
[1] La Manno, G., Soldatov, R., Zeisel, A. et al. RNA velocity of single cells. Nature 560, 494–498 (2018). https://doi.org/10.1038/s41586-018-0414-6
[2] Saelens, W., Cannoodt, R., Todorov, H. et al. A comparison of single-cell trajectory inference methods. Nat Biotechnol 37, 547–554 (2019). https://doi.org/10.1038/s41587-019-0071-9
[3] Amarouch MY, El Hilaly J, Mazouzi D. AFM and FluidFM Technologies: Recent Applications in Molecular and Cellular Biology. Scanning, 7801274 (2018). https://doi.org/10.1155/2018/7801274
doi: https://doi.org/10.1242/prelights.28685
Read preprintHave your say
Sign up to customise the site to your preferences and to receive alerts
Register hereAlso in the molecular biology category:
Cell cycle-dependent mRNA localization in P-bodies
Mohammed JALLOH

Notch3 is a genetic modifier of NODAL signalling for patterning asymmetry during mouse heart looping
Bhaval Parmar

Fetal brain response to maternal inflammation requires microglia
Manuel Lessi
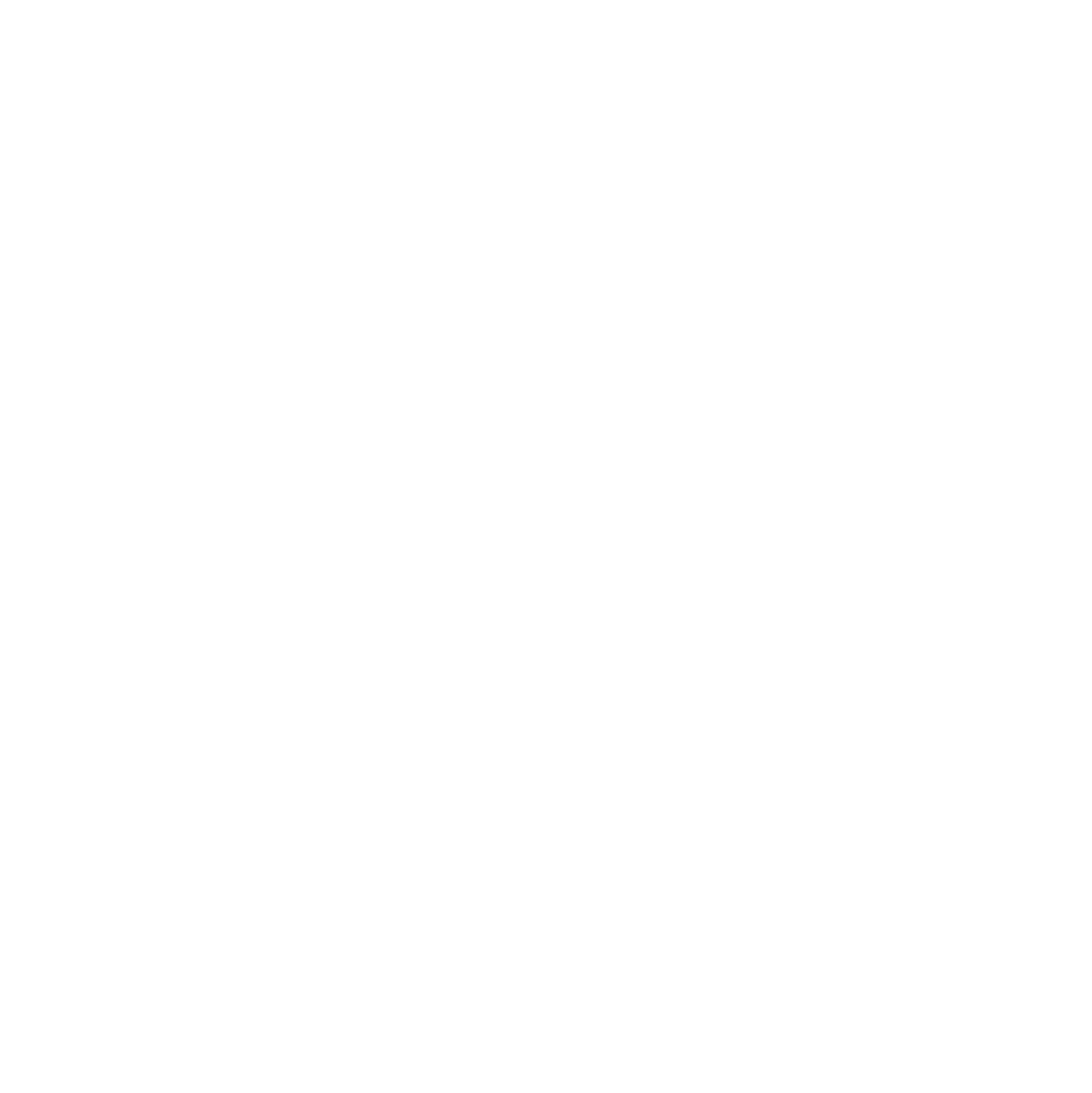
preListsmolecular biology category:
in theBSCB-Biochemical Society 2024 Cell Migration meeting
This preList features preprints that were discussed and presented during the BSCB-Biochemical Society 2024 Cell Migration meeting in Birmingham, UK in April 2024. Kindly put together by Sara Morais da Silva, Reviews Editor at Journal of Cell Science.
List by | Reinier Prosee |
‘In preprints’ from Development 2022-2023
A list of the preprints featured in Development's 'In preprints' articles between 2022-2023
List by | Alex Eve, Katherine Brown |
CSHL 87th Symposium: Stem Cells
Preprints mentioned by speakers at the #CSHLsymp23
List by | Alex Eve |
9th International Symposium on the Biology of Vertebrate Sex Determination
This preList contains preprints discussed during the 9th International Symposium on the Biology of Vertebrate Sex Determination. This conference was held in Kona, Hawaii from April 17th to 21st 2023.
List by | Martin Estermann |
Alumni picks – preLights 5th Birthday
This preList contains preprints that were picked and highlighted by preLights Alumni - an initiative that was set up to mark preLights 5th birthday. More entries will follow throughout February and March 2023.
List by | Sergio Menchero et al. |
CellBio 2022 – An ASCB/EMBO Meeting
This preLists features preprints that were discussed and presented during the CellBio 2022 meeting in Washington, DC in December 2022.
List by | Nadja Hümpfer et al. |
EMBL Synthetic Morphogenesis: From Gene Circuits to Tissue Architecture (2021)
A list of preprints mentioned at the #EESmorphoG virtual meeting in 2021.
List by | Alex Eve |
FENS 2020
A collection of preprints presented during the virtual meeting of the Federation of European Neuroscience Societies (FENS) in 2020
List by | Ana Dorrego-Rivas |
ECFG15 – Fungal biology
Preprints presented at 15th European Conference on Fungal Genetics 17-20 February 2020 Rome
List by | Hiral Shah |
ASCB EMBO Annual Meeting 2019
A collection of preprints presented at the 2019 ASCB EMBO Meeting in Washington, DC (December 7-11)
List by | Madhuja Samaddar et al. |
Lung Disease and Regeneration
This preprint list compiles highlights from the field of lung biology.
List by | Rob Hynds |
MitoList
This list of preprints is focused on work expanding our knowledge on mitochondria in any organism, tissue or cell type, from the normal biology to the pathology.
List by | Sandra Franco Iborra |
3 years
Myong-Hee (Mia) Sung
This is a very important achievement. Congratulations!
A similar S phase effect on NF-kappaB signaling has been noted by Michael White’s group in UK: https://elifesciences.org/articles/10473
Striking that the basal concentration of IkB alpha gene is the top predictor of LPS response in RAW G9 cells.