Can the Insect Path Integration Memory be a Bump Attractor?
Posted on: 11 May 2022 , updated on: 20 June 2022
Preprint posted on 7 April 2022
How can insects remember how far they’ve travelled if there are no landmarks? Investigating the “bump attractor” hypothesis.
Selected by T. W. SchwanitzCategories: animal behavior and cognition, biophysics, neuroscience
Background: A “bump attractor” is effectively the neuronal equivalent of that little line in videogames that tracks how far you go, a stamina bar—connected neurons are lined up together topologically with both inhibitory and excitatory synapses. Excitatory synapses link nearby neurons along the line, while inhibitory synapses link neurons that are further away. Any given neuron along the line excites its immediate neighbors and inhibits those further down the line in both directions, thus creating a “bump” of activity. This type of network therefore has a placeholder function: neuronal excitation remains centered on one spot, which could then be moved by external neuronal stimulation that overrides the balancing act along the line (see Fig. 1B in the preprint).
Bump attractor models are a common explanation for the food-seeking behavior of Cataglyphis fortis, a Saharan ant that forages far from its nest in a desert that is (presumably) devoid of landmarks it can use for orienting itself. Ants of this species travel hundreds of meters away from the nest in a non-linear search, yet they manage to head back home in a straight line. To do so, it is argued that they use some kind of path integration, or “dead-reckoning,” where the ants always keep in mind an invisible line that leads to their nest. Creating such a mental tether requires the ants to maintain a log of both the distances and directions they travel. That is where the bump attractor comes in—it is a theoretical neural network model that could explain how the ants actually track distance travelled. Intriguingly, there are even candidate structures for such a network in the central complex of the ant brain.
In this preprint, Pisokas and Hennig investigate the plausibility of the bump attractor model as an explanation for insect navigation. Despite using their own models coupled with data from two separate studies, they find little support for this neat hypothesis, demonstrating the need for a new hypothesis to explain the neuronal basis for path integration in insects.
Key findings:
- If ants were using a bump attractor neural network, stochastic drift would lead to the neuronal activity bump shifting at a constant rate. Ants were experimentally prevented from returning to their nest for different time periods in two separate empirical studies, and the authors’ analyses show that indeed their homing distance accuracy does deteriorate at a constant rate. This finding is consistent with the bump-attractor hypothesis.
- However, the required number of neurons needed for such a network far exceed what is reasonable for an ant. The authors devise models that try to fit the empirically observed rate of drift to an approximate number of neurons, and the resulting quantities of neurons are simply too high to be biologically reasonable, e.g., 47,000 neurons (for context Drosophila melanogaster has around 200,000 neurons in its entire brain).
- Smaller and more plausible networks (on the order of hundreds of neurons) might be possible if the neurons can sustain their action potentials for longer periods of time; however, the possibility of this has only been demonstrated in mammalian neurons in vitro. It is not clear if this is a biologically relevant possibility for ants in vivo.
- The observed decay rate of homing distance accuracy in ants is biased toward shorter distances, and this bias becomes more pronounced over time. The bump attractor neural network predicts that they should be equally likely to incorrectly make longer trips as they would incorrectly make shorter trips. Trying to correct for this by systematically biasing the neural network toward shorter trips creates a constant distance decay rate, but this does not match with the accelerating decay rate in ants.
- In short, modeled ant behavior based on the bump attractor hypothesis is different from observed ant behavior.
Questions for the authors:
- Your estimate of the required number of neuronal circuits depends upon “reasonable” assumptions about the underlying biology and wiring of an ant’s brain. How big a range of possibilities deviates from the reasonable assumptions you make? Are there any conceivable (albeit perhaps unlikely) sets of assumptions that would lead to a much smaller set of necessary neurons other than the longer action potentials that you discuss?
- Could you speculate on a conceivable alternative model to the bump attractor?
- Is it possible that the desert ants are using landmarks that we simply do not notice because they are small or otherwise difficult for us to detect? Could some combination of landmarks plus a simple bump attractor-based path integration be feasible?
- How can a constant decrease in homing distance accuracy create an accelerating decrease in homing distance?
Why I think the work is important:
Models can help guide empirical studies, yet implausible models could potentially lead to empirical studies that produce less useful data than if it had been gathered with a more likely model in mind. By examining the flaws in an attractive but ultimately unlikely model, the authors save empiricists time and give theoreticians additional impetus to develop a better hypothesis. Falsification of existing hypotheses clears the ground for better ideas.
The problem of how ants (and insects more broadly) can actually handle path integration on a neuronal level is an interesting one, and, since their central nervous systems are smaller, it may be a more tractable problem than similar ones in the larger nervous systems of vertebrates. Furthermore, lessons learned in invertebrates could provide transferrable insights into how neural networks manage to make such calculations.
doi: https://doi.org/10.1242/prelights.31985
Read preprintSign up to customise the site to your preferences and to receive alerts
Register hereAlso in the animal behavior and cognition category:
A depth map of visual space in the primary visual cortex
Wing Gee Shum, Phoebe Reynolds
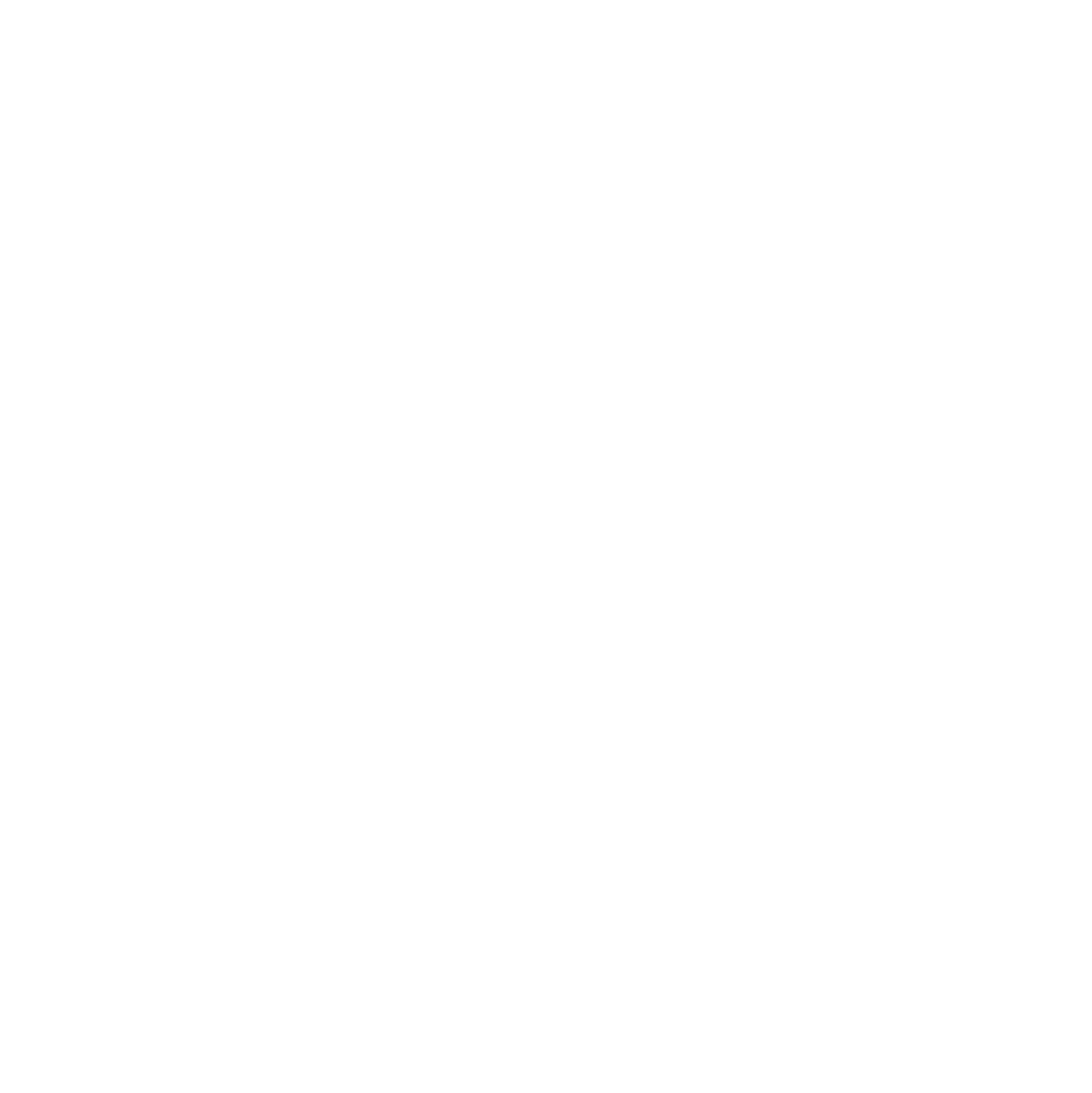
Neural Basis of Number Sense in Larval Zebrafish
Muhammed Sinan Malik

Pharyngeal neuronal mechanisms governing sour taste perception in Drosophila melanogaster
Matthew Davies

Also in the biophysics category:
Motor Clustering Enhances Kinesin-driven Vesicle Transport
Sharvari Pitke

Global coordination of protrusive forces in migrating immune cells
yohalie kalukula

Engineered Nanotopographies Induce Transient Openings in the Nuclear Membrane
Sristilekha Nath
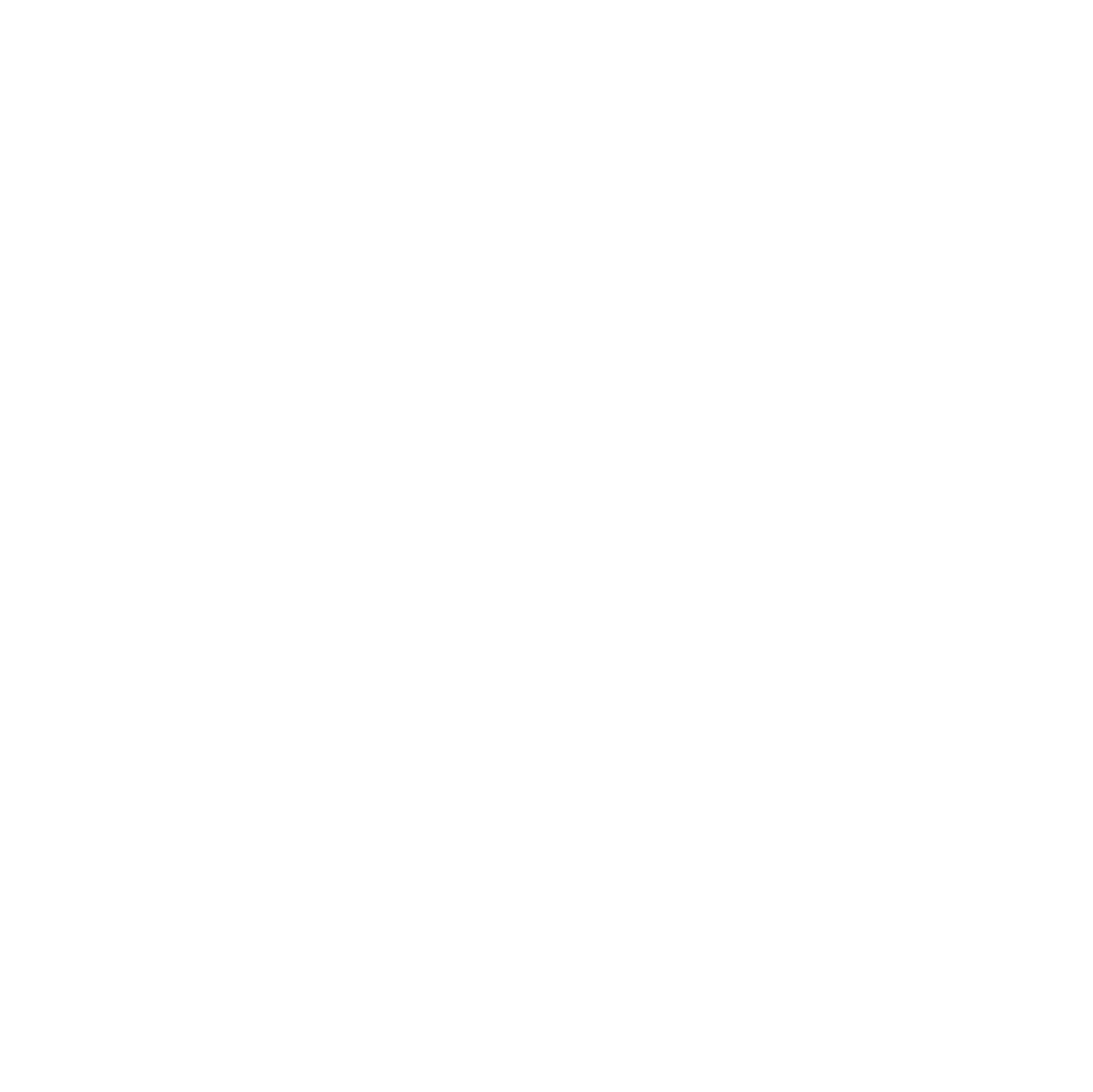
Also in the neuroscience category:
Hippocampal neuroinflammation causes sex-specific disruptions in action selection, food approach memories, and neuronal activation
Nicole Bertola
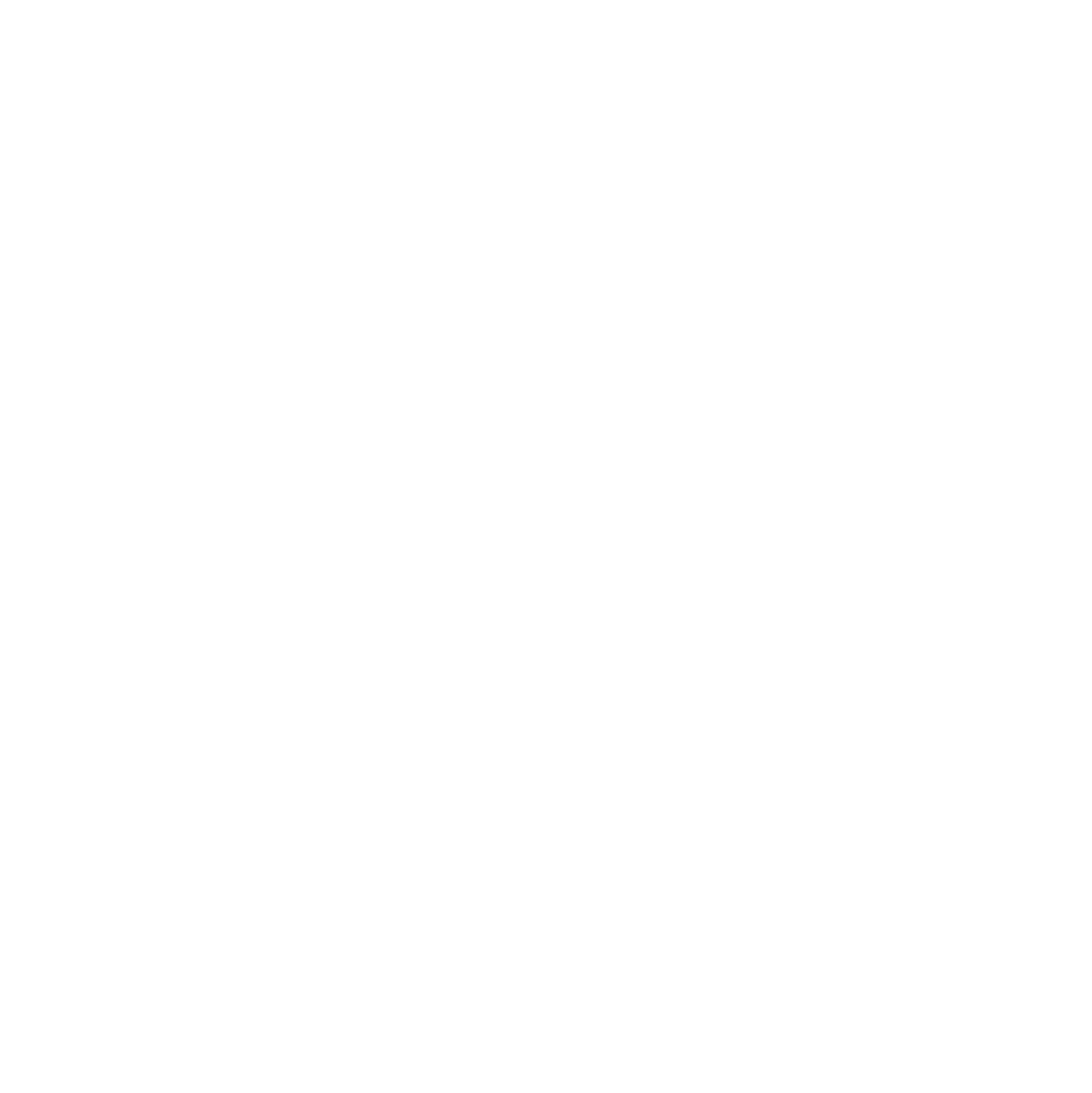
A depth map of visual space in the primary visual cortex
Wing Gee Shum, Phoebe Reynolds
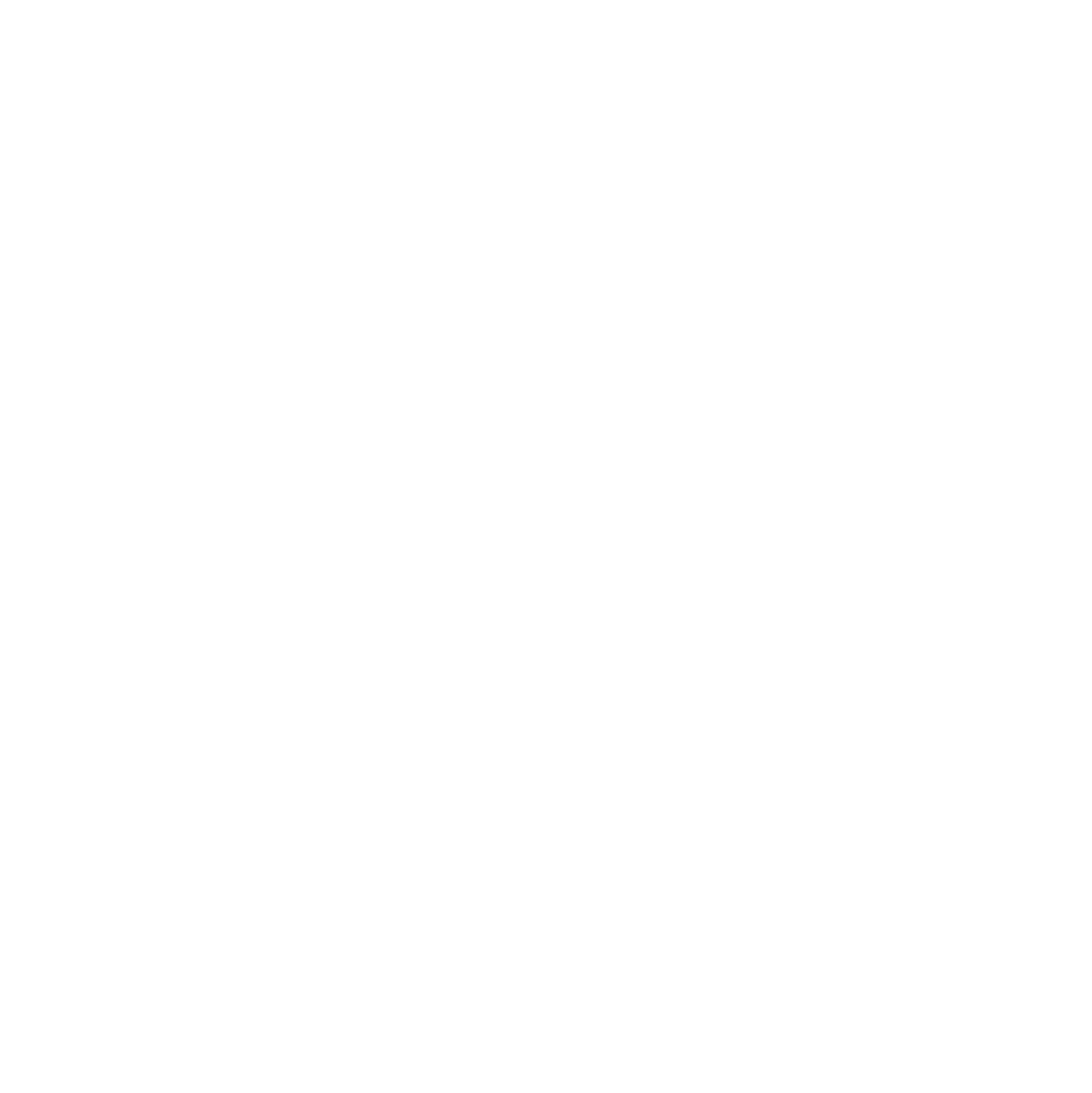
Neural Basis of Number Sense in Larval Zebrafish
Muhammed Sinan Malik

preListsanimal behavior and cognition category:
in the9th International Symposium on the Biology of Vertebrate Sex Determination
This preList contains preprints discussed during the 9th International Symposium on the Biology of Vertebrate Sex Determination. This conference was held in Kona, Hawaii from April 17th to 21st 2023.
List by | Martin Estermann |
Bats
A list of preprints dealing with the ecology, evolution and behavior of bats
List by | Baheerathan Murugavel |
FENS 2020
A collection of preprints presented during the virtual meeting of the Federation of European Neuroscience Societies (FENS) in 2020
List by | Ana Dorrego-Rivas |
Also in the biophysics category:
preLights peer support – preprints of interest
This is a preprint repository to organise the preprints and preLights covered through the 'preLights peer support' initiative.
List by | preLights peer support |
66th Biophysical Society Annual Meeting, 2022
Preprints presented at the 66th BPS Annual Meeting, Feb 19 - 23, 2022 (The below list is not exhaustive and the preprints are listed in no particular order.)
List by | Soni Mohapatra |
EMBL Synthetic Morphogenesis: From Gene Circuits to Tissue Architecture (2021)
A list of preprints mentioned at the #EESmorphoG virtual meeting in 2021.
List by | Alex Eve |
Biophysical Society Meeting 2020
Some preprints presented at the Biophysical Society Meeting 2020 in San Diego, USA.
List by | Tessa Sinnige |
ASCB EMBO Annual Meeting 2019
A collection of preprints presented at the 2019 ASCB EMBO Meeting in Washington, DC (December 7-11)
List by | Madhuja Samaddar et al. |
EMBL Seeing is Believing – Imaging the Molecular Processes of Life
Preprints discussed at the 2019 edition of Seeing is Believing, at EMBL Heidelberg from the 9th-12th October 2019
List by | Dey Lab |
Biomolecular NMR
Preprints related to the application and development of biomolecular NMR spectroscopy
List by | Reid Alderson |
Biophysical Society Annual Meeting 2019
Few of the preprints that were discussed in the recent BPS annual meeting at Baltimore, USA
List by | Joseph Jose Thottacherry |
Also in the neuroscience category:
2024 Hypothalamus GRC
This 2024 Hypothalamus GRC (Gordon Research Conference) preList offers an overview of cutting-edge research focused on the hypothalamus, a critical brain region involved in regulating homeostasis, behavior, and neuroendocrine functions. The studies included cover a range of topics, including neural circuits, molecular mechanisms, and the role of the hypothalamus in health and disease. This collection highlights some of the latest advances in understanding hypothalamic function, with potential implications for treating disorders such as obesity, stress, and metabolic diseases.
List by | Nathalie Krauth |
‘In preprints’ from Development 2022-2023
A list of the preprints featured in Development's 'In preprints' articles between 2022-2023
List by | Alex Eve, Katherine Brown |
CSHL 87th Symposium: Stem Cells
Preprints mentioned by speakers at the #CSHLsymp23
List by | Alex Eve |
Journal of Cell Science meeting ‘Imaging Cell Dynamics’
This preList highlights the preprints discussed at the JCS meeting 'Imaging Cell Dynamics'. The meeting was held from 14 - 17 May 2023 in Lisbon, Portugal and was organised by Erika Holzbaur, Jennifer Lippincott-Schwartz, Rob Parton and Michael Way.
List by | Helen Zenner |
FENS 2020
A collection of preprints presented during the virtual meeting of the Federation of European Neuroscience Societies (FENS) in 2020
List by | Ana Dorrego-Rivas |
ASCB EMBO Annual Meeting 2019
A collection of preprints presented at the 2019 ASCB EMBO Meeting in Washington, DC (December 7-11)
List by | Madhuja Samaddar et al. |
SDB 78th Annual Meeting 2019
A curation of the preprints presented at the SDB meeting in Boston, July 26-30 2019. The preList will be updated throughout the duration of the meeting.
List by | Alex Eve |
Autophagy
Preprints on autophagy and lysosomal degradation and its role in neurodegeneration and disease. Includes molecular mechanisms, upstream signalling and regulation as well as studies on pharmaceutical interventions to upregulate the process.
List by | Sandra Malmgren Hill |
Young Embryologist Network Conference 2019
Preprints presented at the Young Embryologist Network 2019 conference, 13 May, The Francis Crick Institute, London
List by | Alex Eve |