Elucidating the molecular determinants of Aβ aggregation with deep mutational scanning
Posted on: 18 July 2019
Preprint posted on 6 June 2019
Article now published in G3: Genes|Genomes|Genetics at http://dx.doi.org/10.1534/g3.119.400535
Deep mutational scanning reveals the relationships between sequence, structure, and aggregation of the amyloid beta peptide important for the development of Alzheimer’s disease.
Selected by Suzanne McDermottCategories: genomics, molecular biology, neuroscience
Background:
Aggregation of the amyloid β (Aβ) peptide in the brain is thought to play a critical role in the formation of amyloid plaques and the pathogenesis of Alzheimer’s disease. There are several Aβ isoforms that are generated by imprecise cleavage of the Aβ precursor protein at different positions. One of these isoforms, Aβ42, is more prone to aggregation and is the major component of amyloid plaques in Alzheimer’s disease brains. However, our understanding of the relationships between peptide sequence and structure and Aβ aggregation has been limited to in vitro mutagenesis studies of Aβ, and these were not carried out with the disease-relevant Aβ42.
Here, the authors use an unbiased technique called deep mutational scanning to interrogate most if not all possible single amino acid substitutions at every residue of Aβ42 and their effects on aggregation. This powerful approach uses high-throughput sequencing to assess the function of a large number of unique protein variants in parallel (Fowler and Fields 2014; Shin and Cho 2015; Kinney and McCandlish 2019). In general, a library of many protein variants is introduced into a system where the genotype of each variant is linked to a selectable phenotype, and selection is imposed. Variants with high activity increase in frequency, whereas variants with low activity decrease in frequency. The change in the frequency of each variant is measured using high-throughput sequencing and serves as a measure of function. In this study the authors develop a novel yeast-based system where Aβ42 is fused to the essential dihydrofolate reductase (DHFR) protein. Aβ42 aggregation reduces DHFR activity, which slows the growth of yeast cells in the presence of the DHFR inhibitor methotrexate. Thus, cells containing aggregating variants grow slowly upon selection with methotrexate while those containing soluble variants grow rapidly (Figure 1). The frequencies of different Aβ42 variants can be measured before and after methotrexate selection using sequencing and will reflect their solubilities.
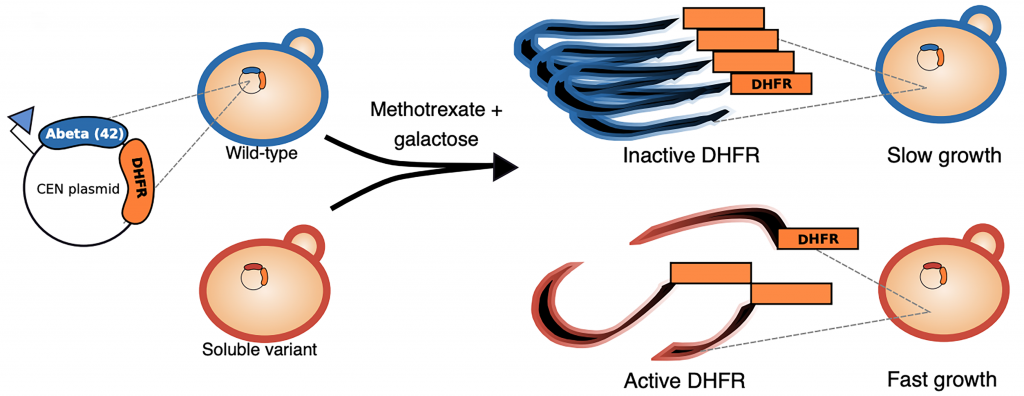
Key findings:
- The group combine a yeast cell-based aggregation assay with deep mutational scanning for the first time, which could be used in the future to study other disease relevant aggregation-prone proteins such a-synuclein (Parkinson’s disease) and transthyretin (transthyretin amyloidosis; ATTR).
- 791 out of a possible 798 single amino acid substitutions across the 42 residues of Aβ42 were interrogated for their effects on aggregation, resulting in a comprehensive Aβ42 sequence-aggregation map.
- While most (75%) substitutions maintained or increased Aβ42 aggregation, the approach revealed 11 positions whose substitution strongly disrupted Aβ42 aggregation, particularly when changed to hydrophilic or charged residues.
- There is some disagreement between the results presented here and previous in vitro mutagenesis studies of different Aβ isoforms. The results of this study are more consistent with structural models based on in vivo derived fibrils than those based on laboratory grown fibrils. These findings therefore highlight the utility of studying the disease-relevant isoform and using a cell-based assay.
What I like about this preprint/why I think it is important:
Mutagenesis can provide functional insight into proteins, but the ability to choose the most informative mutations to make can be limited, for example in highly divergent proteins that lack homology to known protein domains. Unbiased and saturating deep mutational scanning overcomes this limitation, allows many variants to be studied in parallel, and may reveal unexpected results. I believe that this is a powerful approach that is widely applicable to diverse proteins in different systems, as long as function can be assayed in a manner that couples genotype and phenotype. For example, it has been used to probe protein-protein (Starita et al. 2015), protein-nucleic acid, and protein-ligand (Tinberg et al. 2013) interactions, protein stabilities (Matreyek et al. 2018), and enzymatic activities (Starita et al. 2015). More recently, it has been used to understand the phenotypic consequences of many possible missense mutations in human disease genes (Starita et al. 2015; Weile et al. 2017; Matreyek et al. 2018; Stein et al. 2019), to profile mutations that result in drug resistance (Dingens et al. 2019), and to profile viral host tropism (Setoh et al. 2019), replication (Haddox et al. 2016) and evolution (Lee et al. 2018). Furthermore, it is also becoming clear that these types of experiments are key to realizing the potential of directed protein evolution and de novo protein design (Acevedo-Rocha et al. 2018). Elegant assay development is critical for the success of this approach and I am excited to see an example of a novel functional assay described in this preprint, which could be used to study other disease relevant aggregation-prone proteins in the future. This study also highlights the utility of deep mutational scanning data for the evaluation and prediction of protein structure and structural models. This provides an important layer of data that can be potentially added to recently developed integrative structural modeling tools (Hicks et al. 2019; Wollacott et al. 2019).
Future directions and questions for the authors:
- Most of the discussion focuses on the substitutions that result in decreased Aβ42 aggregation. Are any of substitutions associated with increased aggregation in this assay also associated with cases of familial or sporadic Alzheimer’s disease?
- Do you think that this work could be used to rationally design therapeutic peptides or monoclonal antibodies that could potentially inhibit Aβ42 aggregation? Your cell-based assay could also be used to assess the effects of such peptides and antibodies on aggregation, and even to evolve them further using deep mutational scanning.
- Does your deep mutational scanning data, particularly for the C-terminus of the peptide, provide any insight or support any hypothesis as to why Aβ42 is more prone to aggregation than other Aβ isoforms?
References
Acevedo-Rocha CG, Ferla M, Reetz MT. 2018. Directed Evolution of Proteins Based on Mutational Scanning. Methods in molecular biology 1685: 87-128.
Dingens AS, Arenz D, Overbaugh J, Bloom JD. 2019. Massively Parallel Profiling of HIV-1 Resistance to the Fusion Inhibitor Enfuvirtide. Viruses 11.
Fowler DM, Fields S. 2014. Deep mutational scanning: a new style of protein science. Nat Methods 11: 801-807.
Haddox HK, Dingens AS, Bloom JD. 2016. Experimental Estimation of the Effects of All Amino-Acid Mutations to HIV’s Envelope Protein on Viral Replication in Cell Culture. PLoS Pathog 12: e1006114.
Hicks M, Bartha I, di Iulio J, Venter JC, Telenti A. 2019. Functional characterization of 3D protein structures informed by human genetic diversity. Proceedings of the National Academy of Sciences of the United States of America 116: 8960-8965.
Kinney JB, McCandlish DM. 2019. Massively Parallel Assays and Quantitative Sequence-Function Relationships. Annu Rev Genomics Hum Genet.
Lee JM, Huddleston J, Doud MB, Hooper KA, Wu NC, Bedford T, Bloom JD. 2018. Deep mutational scanning of hemagglutinin helps predict evolutionary fates of human H3N2 influenza variants. Proceedings of the National Academy of Sciences of the United States of America 115: E8276-E8285.
Matreyek KA, Starita LM, Stephany JJ, Martin B, Chiasson MA, Gray VE, Kircher M, Khechaduri A, Dines JN, Hause RJ et al. 2018. Multiplex assessment of protein variant abundance by massively parallel sequencing. Nat Genet 50: 874-882.
Setoh YX, Amarilla AA, Peng NYG, Griffiths RE, Carrera J, Freney ME, Nakayama E, Ogawa S, Watterson D, Modhiran N et al. 2019. Determinants of Zika virus host tropism uncovered by deep mutational scanning. Nat Microbiol 4: 876-887.
Shin H, Cho BK. 2015. Rational Protein Engineering Guided by Deep Mutational Scanning. Int J Mol Sci 16: 23094-23110.
Starita LM, Young DL, Islam M, Kitzman JO, Gullingsrud J, Hause RJ, Fowler DM, Parvin JD, Shendure J, Fields S. 2015. Massively Parallel Functional Analysis of BRCA1 RING Domain Variants. Genetics 200: 413-422.
Stein A, Fowler DM, Hartmann-Petersen R, Lindorff-Larsen K. 2019. Biophysical and Mechanistic Models for Disease-Causing Protein Variants. Trends in biochemical sciences.
Tinberg CE, Khare SD, Dou J, Doyle L, Nelson JW, Schena A, Jankowski W, Kalodimos CG, Johnsson K, Stoddard BL et al. 2013. Computational design of ligand-binding proteins with high affinity and selectivity. Nature 501: 212-216.
Weile J, Sun S, Cote AG, Knapp J, Verby M, Mellor JC, Wu Y, Pons C, Wong C, van Lieshout N et al. 2017. A framework for exhaustively mapping functional missense variants. Mol Syst Biol 13: 957.
Wollacott AM, Robinson LN, Ramakrishnan B, Tissire H, Viswanathan K, Shriver Z, Babcock GJ. 2019. Structural prediction of antibody-APRIL complexes by computational docking constrained by antigen saturation mutagenesis library data. J Mol Recognit 32: e2778.
doi: https://doi.org/10.1242/prelights.12146
Read preprintSign up to customise the site to your preferences and to receive alerts
Register hereAlso in the genomics category:
A fine kinetic balance of interactions directs transcription factor hubs to genes
Deevitha Balasubramanian
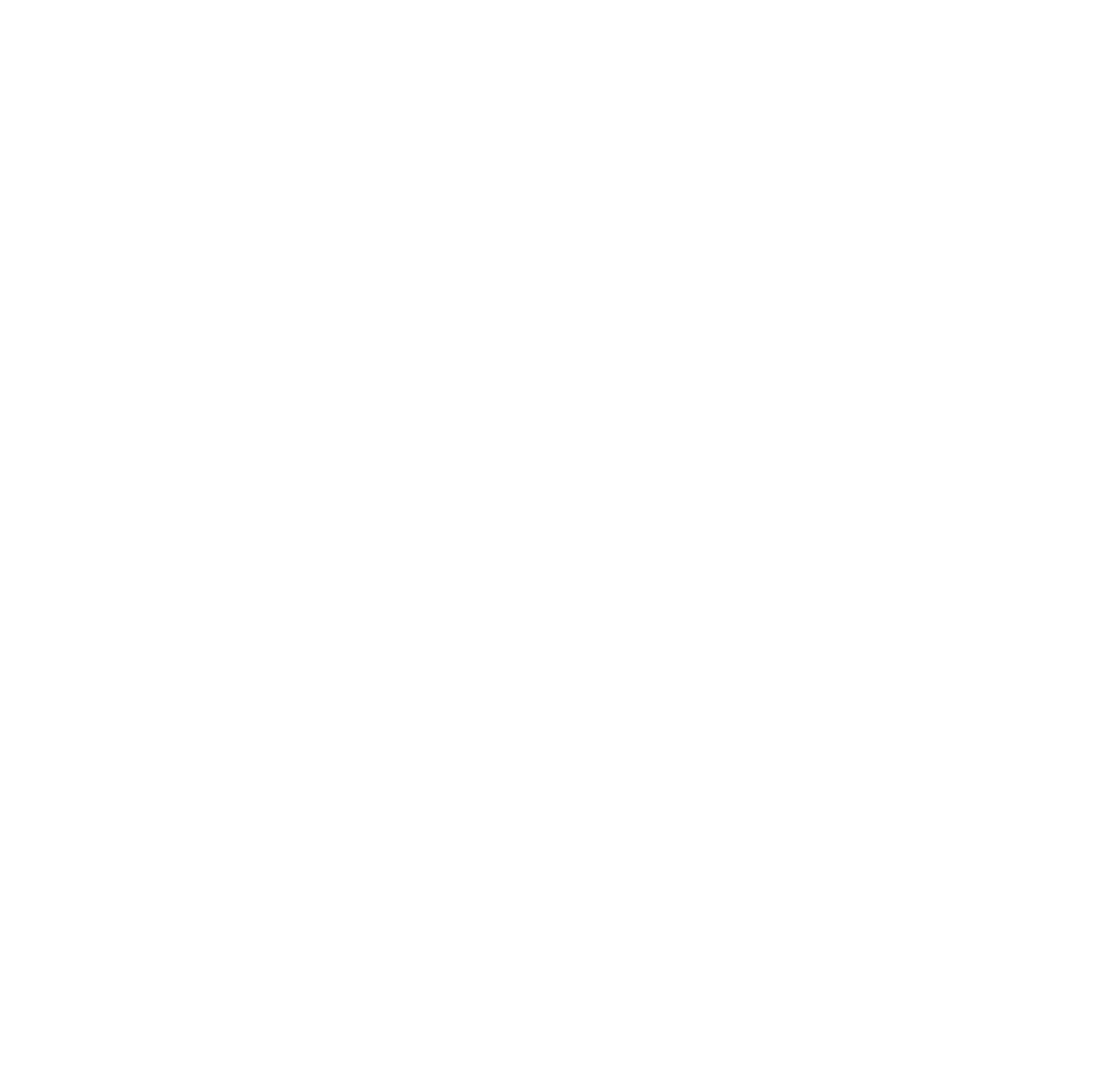
Enhancer-driven cell type comparison reveals similarities between the mammalian and bird pallium
Rodrigo Senovilla-Ganzo

Modular control of time and space during vertebrate axis segmentation
AND
Natural genetic variation quantitatively regulates heart rate and dimension
Girish Kale, Jennifer Ann Black

Also in the molecular biology category:
Cell cycle-dependent mRNA localization in P-bodies
Mohammed JALLOH

Notch3 is a genetic modifier of NODAL signalling for patterning asymmetry during mouse heart looping
Bhaval Parmar

Fetal brain response to maternal inflammation requires microglia
Manuel Lessi
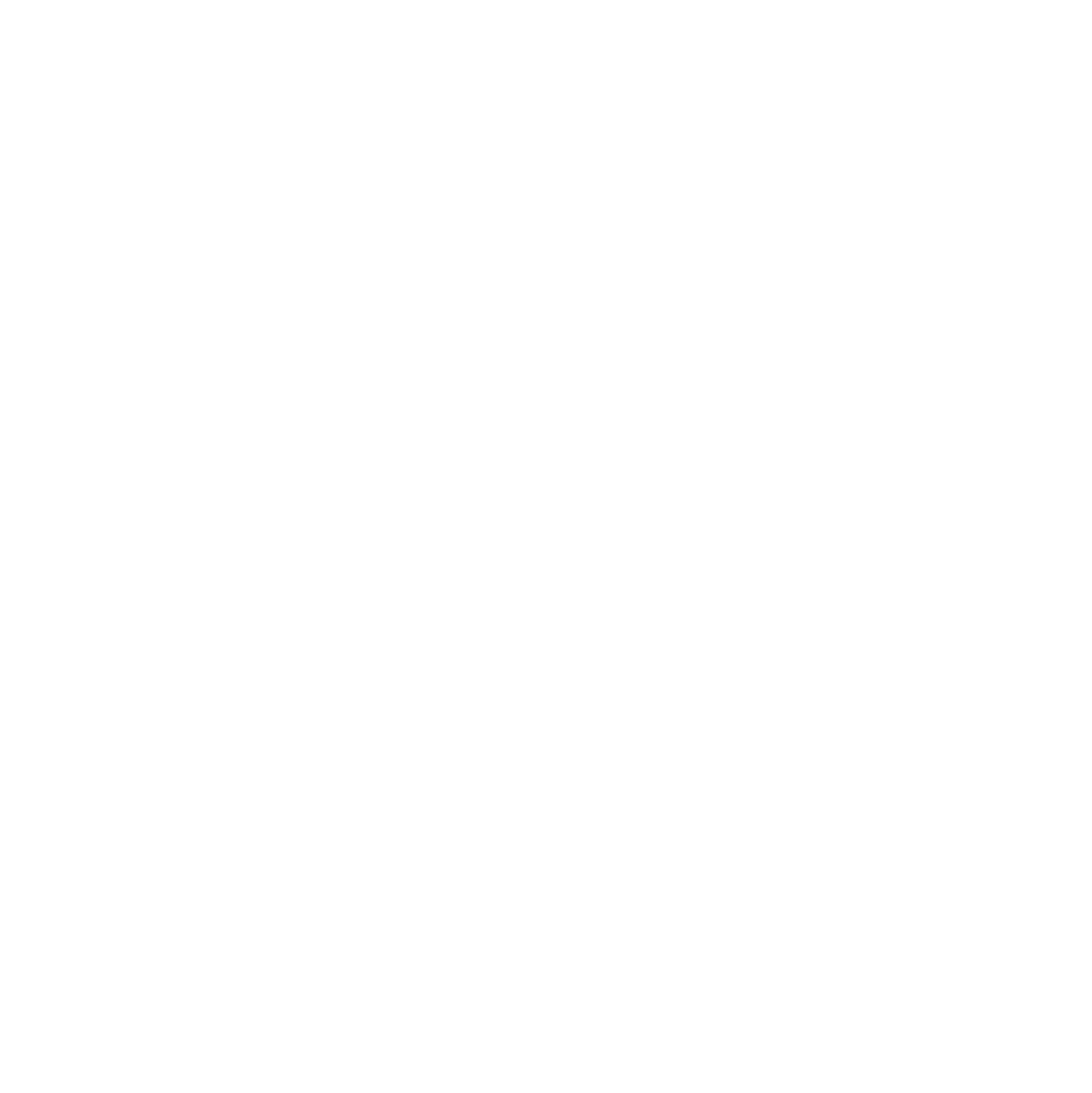
Also in the neuroscience category:
Sexually dimorphic role of diet and stress on behavior, energy metabolism, and the ventromedial hypothalamus
Jimeng Li
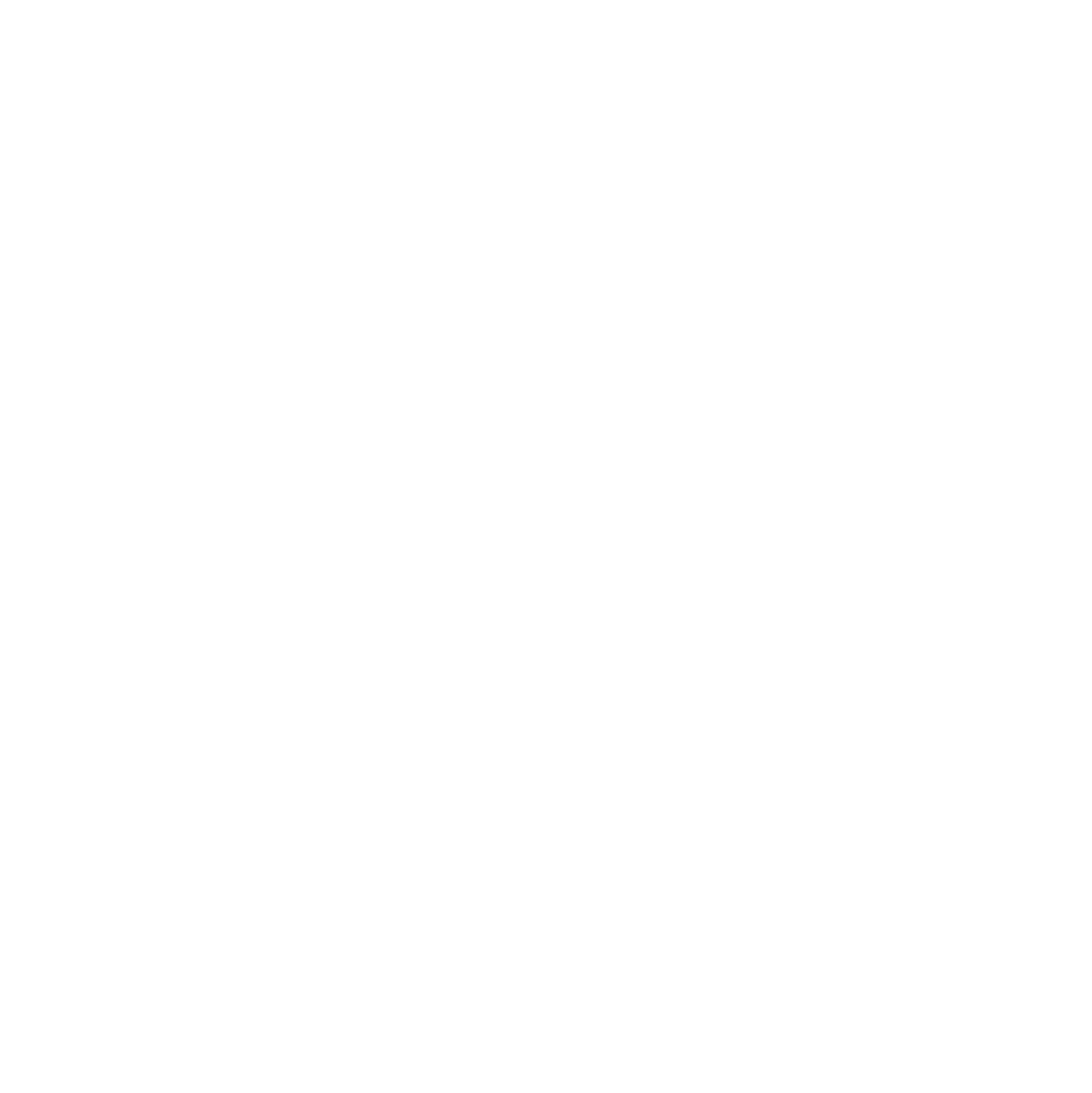
Enhancer-driven cell type comparison reveals similarities between the mammalian and bird pallium
Rodrigo Senovilla-Ganzo

Autism gene variants disrupt enteric neuron migration and cause gastrointestinal dysmotility
Rachel Mckeown
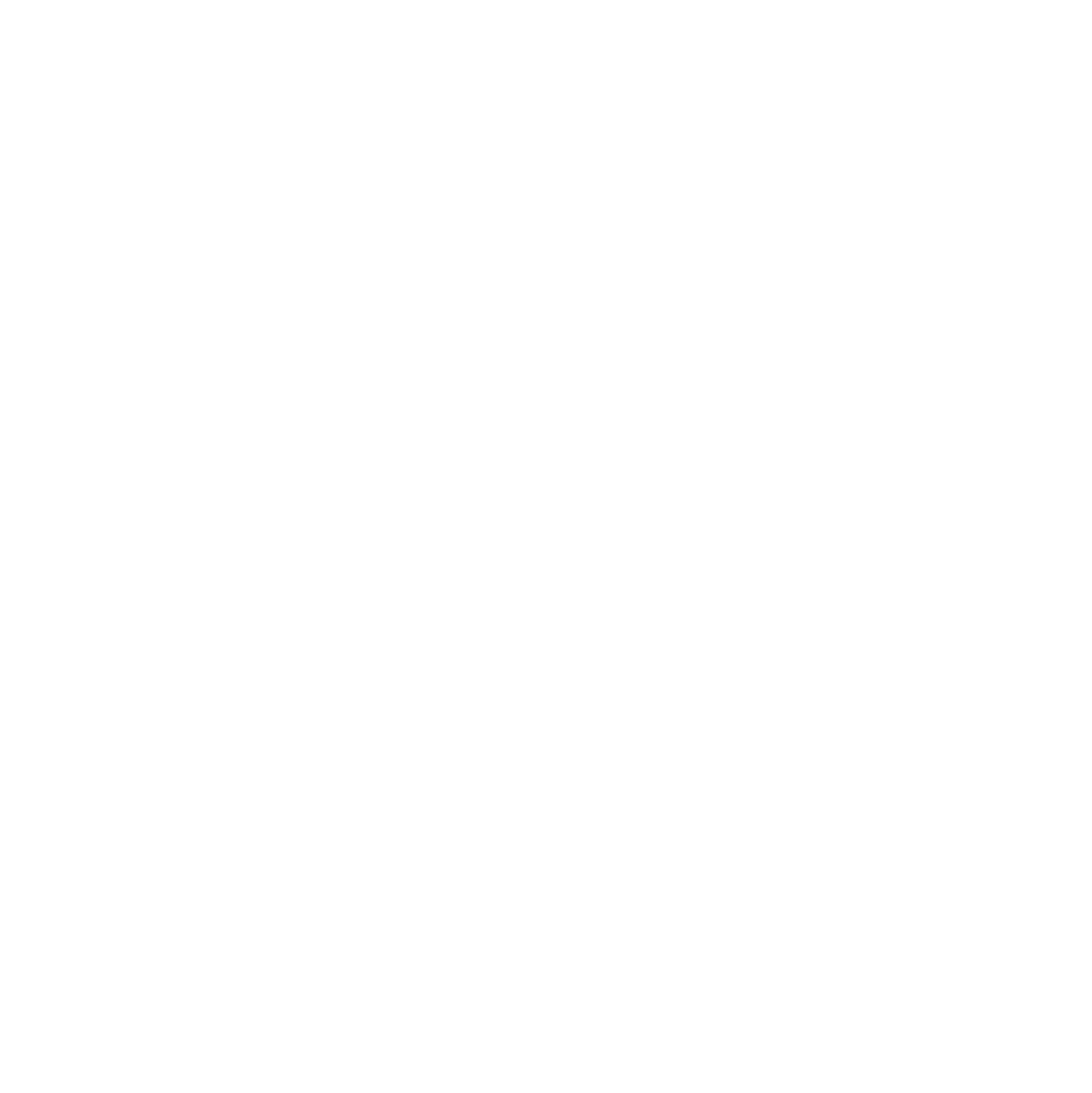
preListsgenomics category:
in theBSCB-Biochemical Society 2024 Cell Migration meeting
This preList features preprints that were discussed and presented during the BSCB-Biochemical Society 2024 Cell Migration meeting in Birmingham, UK in April 2024. Kindly put together by Sara Morais da Silva, Reviews Editor at Journal of Cell Science.
List by | Reinier Prosee |
9th International Symposium on the Biology of Vertebrate Sex Determination
This preList contains preprints discussed during the 9th International Symposium on the Biology of Vertebrate Sex Determination. This conference was held in Kona, Hawaii from April 17th to 21st 2023.
List by | Martin Estermann |
Semmelweis Symposium 2022: 40th anniversary of international medical education at Semmelweis University
This preList contains preprints discussed during the 'Semmelweis Symposium 2022' (7-9 November), organised around the 40th anniversary of international medical education at Semmelweis University covering a wide range of topics.
List by | Nándor Lipták |
20th “Genetics Workshops in Hungary”, Szeged (25th, September)
In this annual conference, Hungarian geneticists, biochemists and biotechnologists presented their works. Link: http://group.szbk.u-szeged.hu/minikonf/archive/prg2021.pdf
List by | Nándor Lipták |
EMBL Conference: From functional genomics to systems biology
Preprints presented at the virtual EMBL conference "from functional genomics and systems biology", 16-19 November 2020
List by | Jesus Victorino |
TAGC 2020
Preprints recently presented at the virtual Allied Genetics Conference, April 22-26, 2020. #TAGC20
List by | Maiko Kitaoka et al. |
Zebrafish immunology
A compilation of cutting-edge research that uses the zebrafish as a model system to elucidate novel immunological mechanisms in health and disease.
List by | Shikha Nayar |
Also in the molecular biology category:
BSCB-Biochemical Society 2024 Cell Migration meeting
This preList features preprints that were discussed and presented during the BSCB-Biochemical Society 2024 Cell Migration meeting in Birmingham, UK in April 2024. Kindly put together by Sara Morais da Silva, Reviews Editor at Journal of Cell Science.
List by | Reinier Prosee |
‘In preprints’ from Development 2022-2023
A list of the preprints featured in Development's 'In preprints' articles between 2022-2023
List by | Alex Eve, Katherine Brown |
CSHL 87th Symposium: Stem Cells
Preprints mentioned by speakers at the #CSHLsymp23
List by | Alex Eve |
9th International Symposium on the Biology of Vertebrate Sex Determination
This preList contains preprints discussed during the 9th International Symposium on the Biology of Vertebrate Sex Determination. This conference was held in Kona, Hawaii from April 17th to 21st 2023.
List by | Martin Estermann |
Alumni picks – preLights 5th Birthday
This preList contains preprints that were picked and highlighted by preLights Alumni - an initiative that was set up to mark preLights 5th birthday. More entries will follow throughout February and March 2023.
List by | Sergio Menchero et al. |
CellBio 2022 – An ASCB/EMBO Meeting
This preLists features preprints that were discussed and presented during the CellBio 2022 meeting in Washington, DC in December 2022.
List by | Nadja Hümpfer et al. |
EMBL Synthetic Morphogenesis: From Gene Circuits to Tissue Architecture (2021)
A list of preprints mentioned at the #EESmorphoG virtual meeting in 2021.
List by | Alex Eve |
FENS 2020
A collection of preprints presented during the virtual meeting of the Federation of European Neuroscience Societies (FENS) in 2020
List by | Ana Dorrego-Rivas |
ECFG15 – Fungal biology
Preprints presented at 15th European Conference on Fungal Genetics 17-20 February 2020 Rome
List by | Hiral Shah |
ASCB EMBO Annual Meeting 2019
A collection of preprints presented at the 2019 ASCB EMBO Meeting in Washington, DC (December 7-11)
List by | Madhuja Samaddar et al. |
Lung Disease and Regeneration
This preprint list compiles highlights from the field of lung biology.
List by | Rob Hynds |
MitoList
This list of preprints is focused on work expanding our knowledge on mitochondria in any organism, tissue or cell type, from the normal biology to the pathology.
List by | Sandra Franco Iborra |
Also in the neuroscience category:
‘In preprints’ from Development 2022-2023
A list of the preprints featured in Development's 'In preprints' articles between 2022-2023
List by | Alex Eve, Katherine Brown |
CSHL 87th Symposium: Stem Cells
Preprints mentioned by speakers at the #CSHLsymp23
List by | Alex Eve |
Journal of Cell Science meeting ‘Imaging Cell Dynamics’
This preList highlights the preprints discussed at the JCS meeting 'Imaging Cell Dynamics'. The meeting was held from 14 - 17 May 2023 in Lisbon, Portugal and was organised by Erika Holzbaur, Jennifer Lippincott-Schwartz, Rob Parton and Michael Way.
List by | Helen Zenner |
FENS 2020
A collection of preprints presented during the virtual meeting of the Federation of European Neuroscience Societies (FENS) in 2020
List by | Ana Dorrego-Rivas |
ASCB EMBO Annual Meeting 2019
A collection of preprints presented at the 2019 ASCB EMBO Meeting in Washington, DC (December 7-11)
List by | Madhuja Samaddar et al. |
SDB 78th Annual Meeting 2019
A curation of the preprints presented at the SDB meeting in Boston, July 26-30 2019. The preList will be updated throughout the duration of the meeting.
List by | Alex Eve |
Autophagy
Preprints on autophagy and lysosomal degradation and its role in neurodegeneration and disease. Includes molecular mechanisms, upstream signalling and regulation as well as studies on pharmaceutical interventions to upregulate the process.
List by | Sandra Malmgren Hill |
Young Embryologist Network Conference 2019
Preprints presented at the Young Embryologist Network 2019 conference, 13 May, The Francis Crick Institute, London
List by | Alex Eve |