Humans optimally anticipate and compensate for an uneven step during walking
Posted on: 29 January 2021
Preprint posted on 5 January 2021
Article now published in eLife at http://dx.doi.org/10.7554/eLife.65402
Maintaining constant speed when walking on an uneven terrain may be possible by minimizing mechanical work. This is observed in a tri-phasic trajectory of speed adjustments by anticipating and using a feedforward approach to the step.
Selected by Jonaz MorenoCategories: bioengineering, biophysics, neuroscience
Background
Human locomotion is associated with the minimization or maximization of different performance criteria. This concept is also referred as optimization of human locomotion and it is well established that when walking under preferred conditions, humans minimize their energy consumption. However, most of the studies have only tested this idea under even terrains like on a treadmill. Alternatively, it has been proposed that humans can plan ahead (feedforward) to preserve speed (momentum). Previous studies of an optimal control model of walking driven by a minimization of total push-off mechanical work can replicate human-like-walking patterns while maintaining the same speed. This is achieved by anticipating for the loss in time when taking a step up (Darici et al., 2018, 2020). So, the purpose of this study was to perform an experiment on humans to confirm whether minimization of total push-off work is a possible criterion to use when uneven terrain is present while walking.
Key findings
Based on the previous optimal control model of walking (Darici et al., 2020), the overall response was to speed up during the mid-stance of walking as soon as 2 steps before the approach to the step; an opposite response was observed when the model had to step-down (Figure 1). This model was driven by the walking dynamics while enforcing a steady, nominal speed. They ran the model with a nominal (no anticipation) and with an anticipatory strategy. The model showed a significant loss of speed during the nominal simulation (Figure 1a) compared to the compensatory approach (Figure 1b, 1c). The overall behavior of the model was consistent despite the changes in walking speed (faster and slower, Figure 1f).
Figure 1. Model predictions for walking on a surface that has a 7.5 cm up-step. (a) Walking speed fluctuations vs time of the model when it does not compensate for the up-step (constant push off). Speed is sampled at mid-stance of each step (prior to step-to-step transition) and denoted with filled symbols. (b) speed fluctuation for optimal up-step compensation that minimizes push-off work. Model anticipates the perturbation with feedforward adjustment to speed up ahead of time, loses momentum atop the perturbation, and then regains speed thereafter. (c) Speed fluctuation for optimal down-step compensation is nearly opposite sign to the up-step compensation. (d) Optimal control inputs are sequence of push-off work, shown for up- and down-step. Up-step requires more work, while down-step is the opposite (less work) to walk same distance in same time. (e) Cumulative time gained for Up and Down-step compensations. (f) Self-similarity of Up-step compensation for three different nominal speeds and two different step lengths (adopted from Darici & Kuo, 2021).
Results from the previous study using the model are the reference to confirm (or refute) whether humans have a similar approach to the step-up and step-down approach. A total of 12 healthy subjects were recruited for this study; they were encouraged to walk at consistent phase without providing any type of feedback. The intension was to mimic the unconstrained nature of daily living. Also, the model did not depend on a particular speed (Darici et al., 2021). Walking speed was measured using IMUs on each foot. The primary outcomes of this study were walking speed (Figure 2), step time, and step length. The average IMU speed measurements were then compared to the overall behavior of the model (Figure 3).
Figure 2. The graphs are organized by rows representing the nominal condition (no step, control), up-step, and down-step. The columns represent another grouping: the first column (left) Illustrates individual trials from three out of 12 subjects. The tick lines represent the average data from each of the three participants with the standard deviation (shaded regions ± 1 s.d.). The middle column is the average data of each individual subject (12 subjects, each different color). The right column Illustrates the average between subjects (all subjects) with the standard deviation of across all subjects (light shaded region), and standard deviation ignoring subject-dependent speed (darker shaded region) (adopted from Darici & Kuo, 2021).
Figure 3. Comparison of model and human walking speed fluctuations vs. time corresponding for the up-step (left column), and the down-steps (right column). The top row is the model speed fluctuations predicted to minimize push-off mechanical work, while the bottom row is the experimentally measured compensation strategies for humans showing average speed pattern across subjects (shaded regions denoted ± 1 s.d. after eliminating variations in average speed). The vertical dashed line denotes the first up-step (left column) and down-step (right column). The horizontal dashed line denotes the average walking speed (adopted from Darici & Kuo, 2021).
Their conclusion was that the normal response to changes in terrain are associated with an anticipatory approach. Humans increase their speed at about 2 steps before the observed change in the landscape to maintain the overall same speed. From the optimal control model of previous studies, this response is coupled with the minimization of push-off work, as the overall shape of speed curve of the model and human responses are very similar to each other. The authors concluded that this compensatory response was a systematic, tri-phasic pattern in walking speed fluctuation that may be related to the use of the central nervous system control with feedback and feedforward (anticipatory) components.
What I liked about this preprint
The area of human movement optimization has been around for very long time. The most predominant concept is that humans optimize energy consumption when walking under preferred conditions (i.e., preferred walking speed or stride frequency). One of the limitations to this area of research is that we are still unable to really understand how humans can behave under an optimal control framework. Continuing to test out whether optimal control theory is crucial for natural human walking patterns and can provide valuable information that can potentially lead to improving assistive devices, or have a new insight to develop new and different rehabilitation practices that can improve recovery on special populations that deviate from this optimal control framework.
Open Questions
- You have mentioned that one of the ways people can gather information to anticipate and provide a feedforward control is through Would it be possible to make changes in the visual system (i.e., make our vision blurry) and run this study again to see if we are only relying on the visual information? Any thoughts on the role of the vestibular system and its link to the visual system?
- Would you expect to see similar responses to different step heights?
- One of the main limitations around the optimal control theory is that the conclusions are typically drawn from laboratory experiments. I like the fact that you implemented IMUs to get your dependent variables. Do you intend to build upon this and maybe have people walk outside the laboratory?
References
Darici, O., Temeltas, H., & Kuo, A. D. (2018). Optimal regulation of bipedal walking speed despite an unexpected bump in the road. PLoS ONE, 13(9), 1–16. https://doi.org/10.1371/journal.pone.0204205
Darici, O., Temeltas, H., & Kuo, A. D. (2020). Anticipatory Control of Momentum for Bipedal Walking on Uneven Terrain. Scientific Reports, 10(1), 1–12. https://doi.org/10.1038/s41598-019-57156-6
doi: https://doi.org/10.1242/prelights.27200
Read preprintSign up to customise the site to your preferences and to receive alerts
Register hereAlso in the bioengineering category:
Scalable and efficient generation of mouse primordial germ cell-like cells
Carly Guiltinan

Generalized Biomolecular Modeling and Design with RoseTTAFold All-Atom
Saanjbati Adhikari
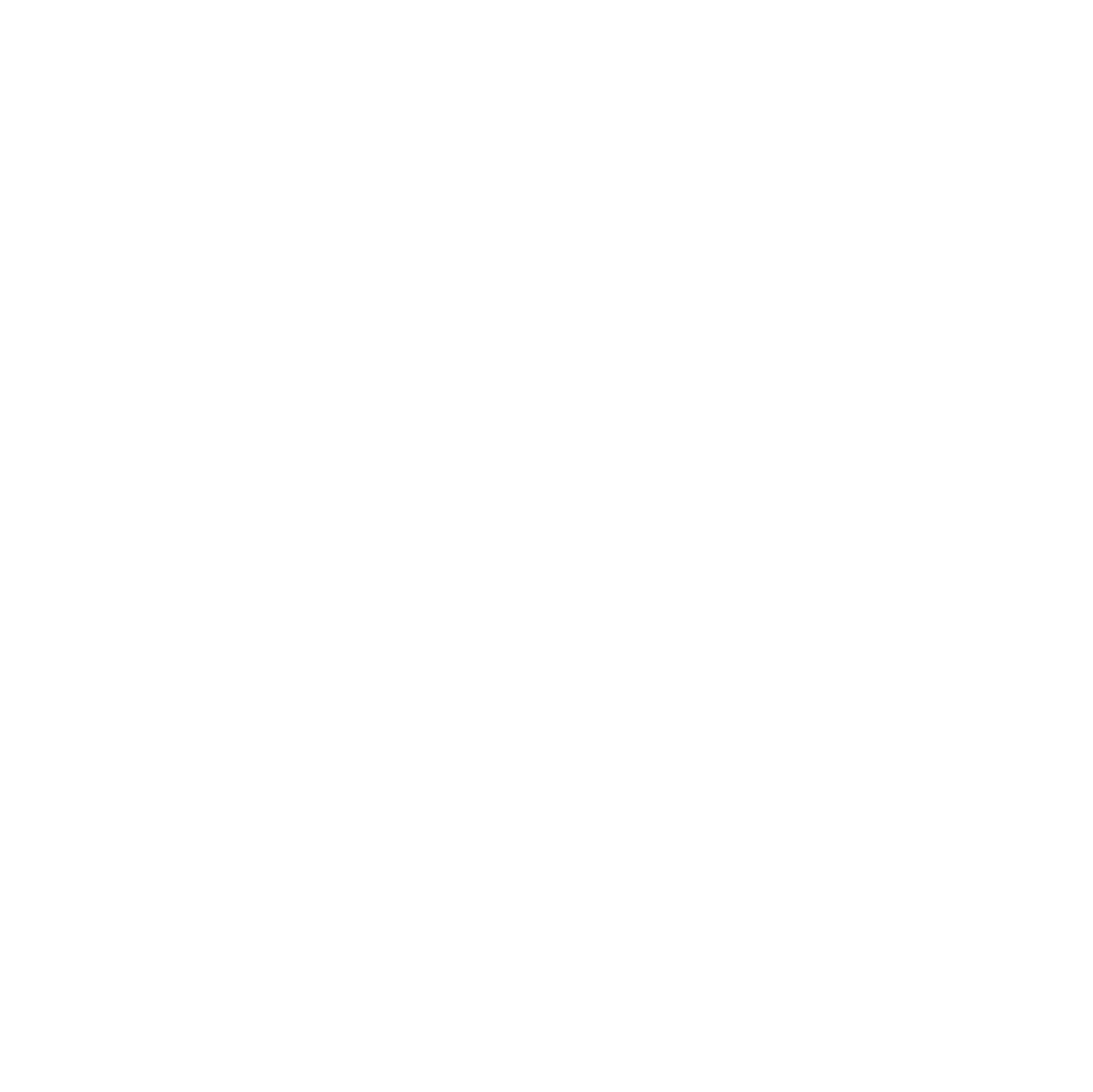
Multi-pass, single-molecule nanopore reading of long protein strands with single-amino acid sensitivity
Benjamin Dominik Maier, Samantha Seah
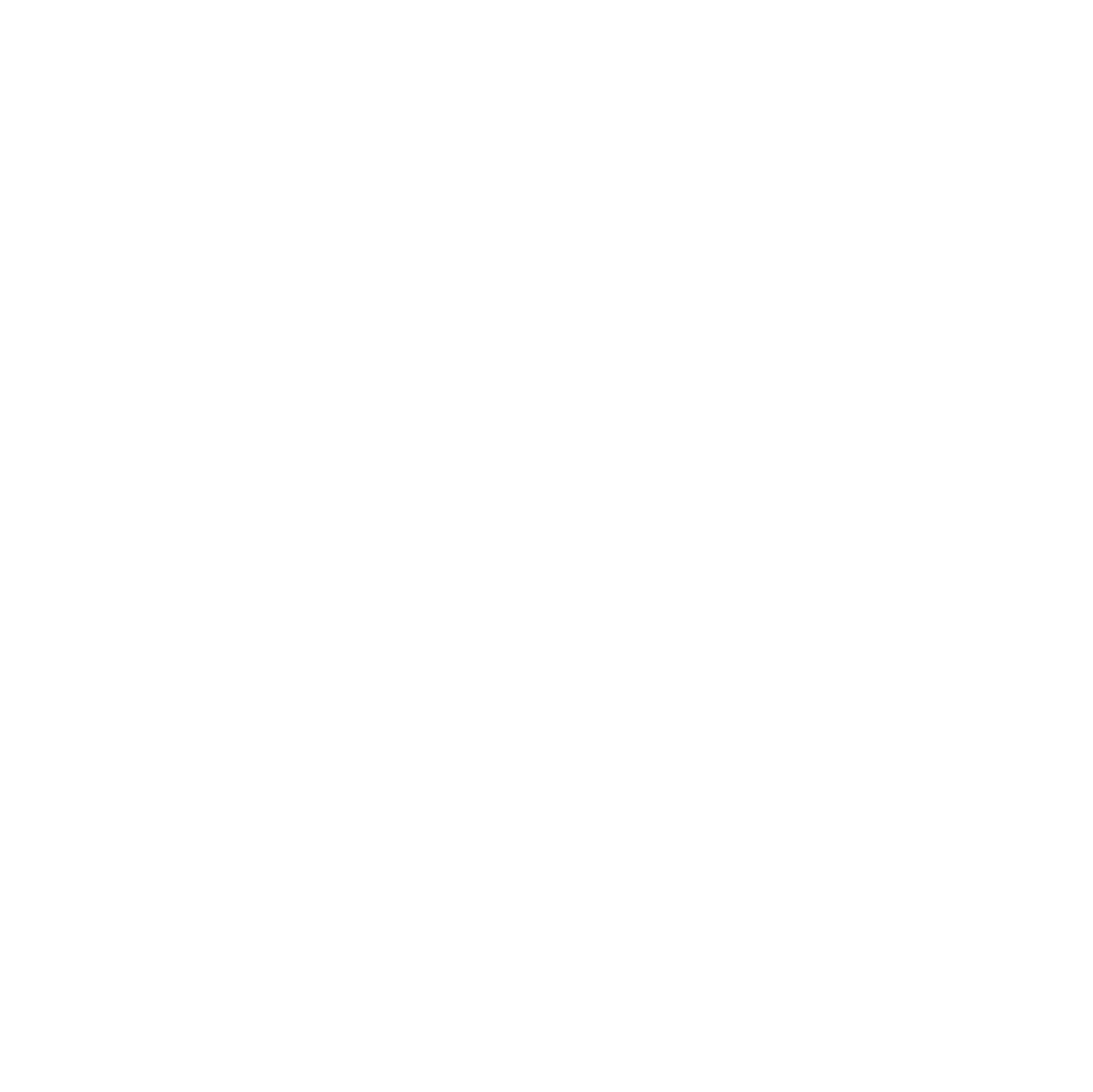
Also in the biophysics category:
Topology changes of the regenerating Hydra define actin nematic defects as mechanical organizers of morphogenesis
Rachel Mckeown

Structural basis of respiratory complexes adaptation to cold temperatures
Pamela Ornelas

Actin polymerization drives lumen formation in a human epiblast model
Megane Rayer, Rivka Shapiro

Also in the neuroscience category:
Sexually dimorphic role of diet and stress on behavior, energy metabolism, and the ventromedial hypothalamus
Jimeng Li
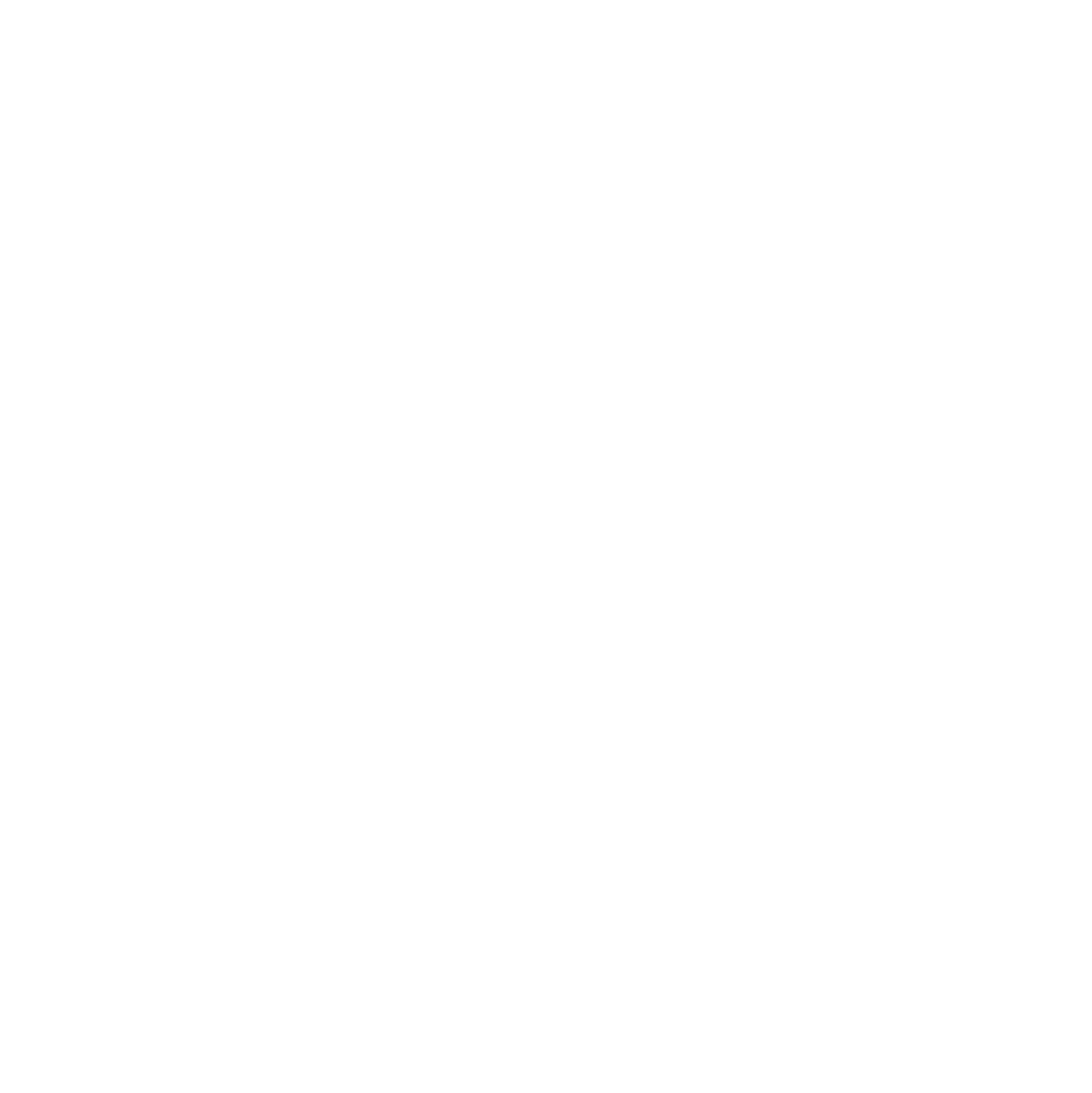
Enhancer-driven cell type comparison reveals similarities between the mammalian and bird pallium
Rodrigo Senovilla-Ganzo

Autism gene variants disrupt enteric neuron migration and cause gastrointestinal dysmotility
Rachel Mckeown
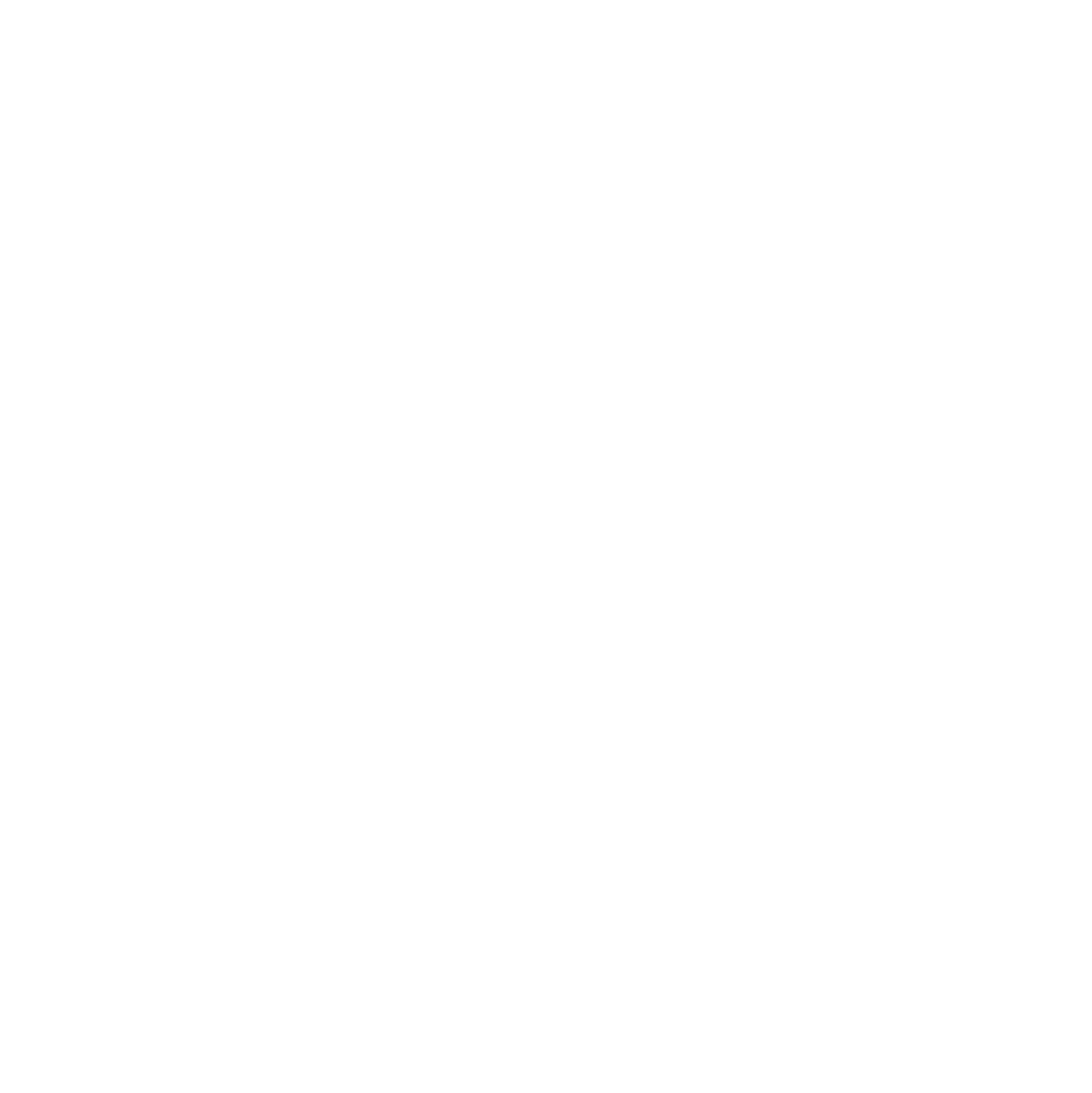
preListsbioengineering category:
in theCSHL 87th Symposium: Stem Cells
Preprints mentioned by speakers at the #CSHLsymp23
List by | Alex Eve |
EMBL Synthetic Morphogenesis: From Gene Circuits to Tissue Architecture (2021)
A list of preprints mentioned at the #EESmorphoG virtual meeting in 2021.
List by | Alex Eve |
3D Gastruloids
A curated list of preprints related to Gastruloids (in vitro models of early development obtained by 3D aggregation of embryonic cells). Updated until July 2021.
List by | Paul Gerald L. Sanchez and Stefano Vianello |
ASCB EMBO Annual Meeting 2019
A collection of preprints presented at the 2019 ASCB EMBO Meeting in Washington, DC (December 7-11)
List by | Madhuja Samaddar et al. |
EMBL Seeing is Believing – Imaging the Molecular Processes of Life
Preprints discussed at the 2019 edition of Seeing is Believing, at EMBL Heidelberg from the 9th-12th October 2019
List by | Dey Lab |
Lung Disease and Regeneration
This preprint list compiles highlights from the field of lung biology.
List by | Rob Hynds |
Advances in microscopy
This preList highlights exciting unpublished preprint articles describing advances in microscopy with a focus on light-sheet microscopy.
List by | Stephan Daetwyler |
Also in the biophysics category:
preLights peer support – preprints of interest
This is a preprint repository to organise the preprints and preLights covered through the 'preLights peer support' initiative.
List by | preLights peer support |
66th Biophysical Society Annual Meeting, 2022
Preprints presented at the 66th BPS Annual Meeting, Feb 19 - 23, 2022 (The below list is not exhaustive and the preprints are listed in no particular order.)
List by | Soni Mohapatra |
EMBL Synthetic Morphogenesis: From Gene Circuits to Tissue Architecture (2021)
A list of preprints mentioned at the #EESmorphoG virtual meeting in 2021.
List by | Alex Eve |
Biophysical Society Meeting 2020
Some preprints presented at the Biophysical Society Meeting 2020 in San Diego, USA.
List by | Tessa Sinnige |
ASCB EMBO Annual Meeting 2019
A collection of preprints presented at the 2019 ASCB EMBO Meeting in Washington, DC (December 7-11)
List by | Madhuja Samaddar et al. |
EMBL Seeing is Believing – Imaging the Molecular Processes of Life
Preprints discussed at the 2019 edition of Seeing is Believing, at EMBL Heidelberg from the 9th-12th October 2019
List by | Dey Lab |
Biomolecular NMR
Preprints related to the application and development of biomolecular NMR spectroscopy
List by | Reid Alderson |
Biophysical Society Annual Meeting 2019
Few of the preprints that were discussed in the recent BPS annual meeting at Baltimore, USA
List by | Joseph Jose Thottacherry |
Also in the neuroscience category:
‘In preprints’ from Development 2022-2023
A list of the preprints featured in Development's 'In preprints' articles between 2022-2023
List by | Alex Eve, Katherine Brown |
CSHL 87th Symposium: Stem Cells
Preprints mentioned by speakers at the #CSHLsymp23
List by | Alex Eve |
Journal of Cell Science meeting ‘Imaging Cell Dynamics’
This preList highlights the preprints discussed at the JCS meeting 'Imaging Cell Dynamics'. The meeting was held from 14 - 17 May 2023 in Lisbon, Portugal and was organised by Erika Holzbaur, Jennifer Lippincott-Schwartz, Rob Parton and Michael Way.
List by | Helen Zenner |
FENS 2020
A collection of preprints presented during the virtual meeting of the Federation of European Neuroscience Societies (FENS) in 2020
List by | Ana Dorrego-Rivas |
ASCB EMBO Annual Meeting 2019
A collection of preprints presented at the 2019 ASCB EMBO Meeting in Washington, DC (December 7-11)
List by | Madhuja Samaddar et al. |
SDB 78th Annual Meeting 2019
A curation of the preprints presented at the SDB meeting in Boston, July 26-30 2019. The preList will be updated throughout the duration of the meeting.
List by | Alex Eve |
Autophagy
Preprints on autophagy and lysosomal degradation and its role in neurodegeneration and disease. Includes molecular mechanisms, upstream signalling and regulation as well as studies on pharmaceutical interventions to upregulate the process.
List by | Sandra Malmgren Hill |
Young Embryologist Network Conference 2019
Preprints presented at the Young Embryologist Network 2019 conference, 13 May, The Francis Crick Institute, London
List by | Alex Eve |