Lineage tracing on transcriptional landscapes links state to fate during differentiation
Posted on: 5 February 2019 , updated on: 6 February 2019
Preprint posted on 1 December 2018
Article now published in Science at http://dx.doi.org/10.1126/science.aaw3381
Lineage-traced scRNA-seq reveals that inheritable fate restriction occurring early in hematopoiesis is not detected computationally from the progenitor transcriptome.
Selected by Yen-Chung ChenCategories: bioinformatics, developmental biology, systems biology
Background and context
Developmental biologists have long been picturing development as a process starting from a homogeneous population which becomes more and more different and eventually gives rise to a variety of terminally differentiated cell types. Waddington envisioned that this process governed by an “epigenetic landscape” is defined by genes and their interactions. Some key genes in the specification processes have been discovered since then, but the major regulators for most of the states in developmental processes remain uncharted to date.
The advent of massively parallel profiling techniques for single cells allows capturing a snapshot of a huge number of cell states simultaneously. When capturing cells from different stages, the similarity between the cells can be utilized to determine cell types and to reconstruct the developmental paths leading to each type.
Single cell profiling techniques have helped in the discovery of otherwise unannotated cell types and genes that might tune how a cell commits to a particular fate. However, because the techniques only capture snapshots of different states but not how they change in time, it’s challenging to determine whether each cell decides their goal early on and progresses along that particular branch to become a certain type, or if the cells remain plastic and carry the potential to switch trajectories along the way before becoming terminally differentiated. As a result, the reconstructed developmental paths need to be validated by experiments like lineage tracing to understand the degree of coupling between an early state and a terminally differentiated state.
Key findings
Lineage tracing experiments are often of lower throughput and require a specific early marker. One way to address this challenge is to label developing cells with unique barcodes that are heritable through cell divisions, and infer later whether two cell types come from the same progenitor pool by calculating the degree of barcode overlap between the two mature cell types. In this preprint, Weinreb et al. first barcoded murine hematopoietic progenitor cells using lentivirus. Then, the cells were cultured in a condition enabling multilineage differentiation and were allowed to proliferate for two days. The cells were collected on day 2, day 4, and day 6 of in vitro differentiation. By matching barcodes, this technique gives transcriptome and clonal information for multiple stages of differentiation.
Collecting clonal and transcriptome information allowed the authors to examine two important questions: Whether the differentiating cells are really progressing through a branching process, in which one cell cannot contribute to alternative fates once it has passed the branching point, and whether the time of branching event can be determined from the transcriptome alone (See figure 1).
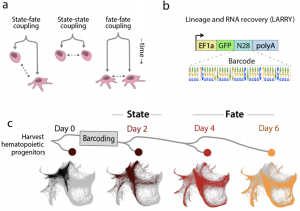
The authors first demonstrated that the cells of a particular transcriptionally defined type share most of their barcodes, indicating that transcriptionally determined cell types arose from different populations of progenitors. Then, the authors compared if transcriptomes of differentiated sister cells (“lateral prediction”) predict a cell’s fate better than the transcriptomes of progenitor sister cells (“forward prediction”), and discovered predictions from differentiated sister cells are more accurate. This difference in prediction power before and after differentiation could either be a result of the noisy nature of single cell RNA-seq which masks minor transcriptional differences, or it suggests that cellular properties other than the transcriptome also modulate cell fate decision.
To understand the exact point when a cell’s fate is specified, the authors used several computational methods to predict the time of diversification events, and compared the predicted time points to the point when different clones no longer contribute to the alternative fate. It turned out a cell’s ability to commit to other paths is restricted much earlier than what was predicted computationally based on the transcriptome alone.
Taken together, by combining clonal and transcriptome information, the authors showed that transcriptionally defined cell types in hematopoiesis are also clonally distinct. Furthermore, they showed that changes in the transcriptome correlate well with developmental time, and that fate restriction precedes overall transcriptomic discretion in development and might be better predicted by examining master cell fate regulators alone.
Why I like this preprint
The high-throughput nature of single cell profiling techniques enables researchers to study cells with different states with a high resolution. Nonetheless, it is challenging to understand how these states are related to each other because the profiling technique is essentially snapshot taking and provides no information about relationships. Multiple assumptions are required in developmental biology to learn the continuous process of development from single cell profiling. Two common assumptions are that the differentiation process is a branching and definite process, and that similarity in transcriptomes can be interpreted as temporal proximity. This preprint focuses on these key questions and benchmarks them experimentally. Although the necessity to separate sister cells limits the use of this technique, when applicable, it could be used to better capture development as a continuous process.
Open questions
- In a previous study [1], the authors utilized an elegant clonal tagging by an inducible transposable element in a mouse model, while lentiviral transduction was used in the preprint. I was wondering what the considerations behind the different methods chosen.
- The authors devised an elegant and user-friendly visualization tool for single cell profiling data [2], and in this preprint, the authors used SPRING plot to visualize multimodal (expression and clonal identity) data. I was wondering if it would be a plan to add this functionality to the tool, for it would be a great asset to the community.
References
- Sun, J. et al. Clonal dynamics of native haematopoiesis. Nature 514, 322–327 (2014).
- Weinreb, C., Wolock, S. & Klein, A. M. SPRING: A kinetic interface for visualizing high dimensional single-cell expression data. Bioinformatics 34, 1246–1248 (2018).
doi: https://doi.org/10.1242/prelights.8308
Read preprintHave your say
Sign up to customise the site to your preferences and to receive alerts
Register hereAlso in the bioinformatics category:
Enhancer-driven cell type comparison reveals similarities between the mammalian and bird pallium
Rodrigo Senovilla-Ganzo

Expressive modeling and fast simulation for dynamic compartments
Benjamin Dominik Maier

Transcriptional profiling of human brain cortex identifies novel lncRNA-mediated networks dysregulated in amyotrophic lateral sclerosis
Julio Molina Pineda
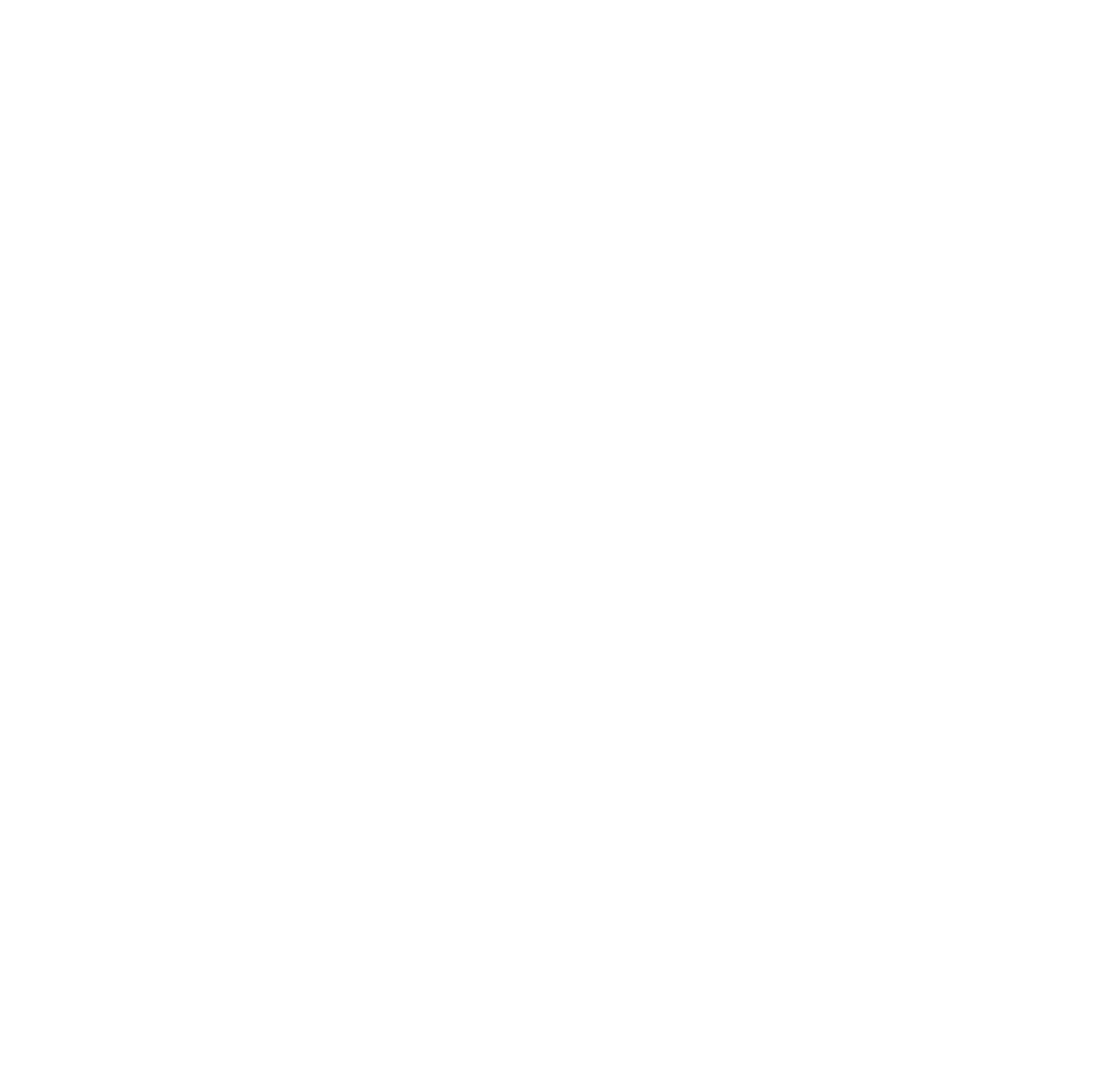
Also in the developmental biology category:
Gestational exposure to high heat-humidity conditions impairs mouse embryonic development
Girish Kale, preLights peer support

Modular control of time and space during vertebrate axis segmentation
AND
Natural genetic variation quantitatively regulates heart rate and dimension
Girish Kale, Jennifer Ann Black

Notch3 is a genetic modifier of NODAL signalling for patterning asymmetry during mouse heart looping
Bhaval Parmar

Also in the systems biology category:
Modular control of time and space during vertebrate axis segmentation
AND
Natural genetic variation quantitatively regulates heart rate and dimension
Girish Kale, Jennifer Ann Black

Expressive modeling and fast simulation for dynamic compartments
Benjamin Dominik Maier

Clusters of lineage-specific genes are anchored by ZNF274 in repressive perinucleolar compartments
Silvia Carvalho

preListsbioinformatics category:
in the‘In preprints’ from Development 2022-2023
A list of the preprints featured in Development's 'In preprints' articles between 2022-2023
List by | Alex Eve, Katherine Brown |
9th International Symposium on the Biology of Vertebrate Sex Determination
This preList contains preprints discussed during the 9th International Symposium on the Biology of Vertebrate Sex Determination. This conference was held in Kona, Hawaii from April 17th to 21st 2023.
List by | Martin Estermann |
Alumni picks – preLights 5th Birthday
This preList contains preprints that were picked and highlighted by preLights Alumni - an initiative that was set up to mark preLights 5th birthday. More entries will follow throughout February and March 2023.
List by | Sergio Menchero et al. |
Fibroblasts
The advances in fibroblast biology preList explores the recent discoveries and preprints of the fibroblast world. Get ready to immerse yourself with this list created for fibroblasts aficionados and lovers, and beyond. Here, my goal is to include preprints of fibroblast biology, heterogeneity, fate, extracellular matrix, behavior, topography, single-cell atlases, spatial transcriptomics, and their matrix!
List by | Osvaldo Contreras |
Single Cell Biology 2020
A list of preprints mentioned at the Wellcome Genome Campus Single Cell Biology 2020 meeting.
List by | Alex Eve |
Antimicrobials: Discovery, clinical use, and development of resistance
Preprints that describe the discovery of new antimicrobials and any improvements made regarding their clinical use. Includes preprints that detail the factors affecting antimicrobial selection and the development of antimicrobial resistance.
List by | Zhang-He Goh |
Also in the developmental biology category:
BSDB/GenSoc Spring Meeting 2024
A list of preprints highlighted at the British Society for Developmental Biology and Genetics Society joint Spring meeting 2024 at Warwick, UK.
List by | Joyce Yu, Katherine Brown |
GfE/ DSDB meeting 2024
This preList highlights the preprints discussed at the 2024 joint German and Dutch developmental biology societies meeting that took place in March 2024 in Osnabrück, Germany.
List by | Joyce Yu |
‘In preprints’ from Development 2022-2023
A list of the preprints featured in Development's 'In preprints' articles between 2022-2023
List by | Alex Eve, Katherine Brown |
preLights peer support – preprints of interest
This is a preprint repository to organise the preprints and preLights covered through the 'preLights peer support' initiative.
List by | preLights peer support |
The Society for Developmental Biology 82nd Annual Meeting
This preList is made up of the preprints discussed during the Society for Developmental Biology 82nd Annual Meeting that took place in Chicago in July 2023.
List by | Joyce Yu, Katherine Brown |
CSHL 87th Symposium: Stem Cells
Preprints mentioned by speakers at the #CSHLsymp23
List by | Alex Eve |
Journal of Cell Science meeting ‘Imaging Cell Dynamics’
This preList highlights the preprints discussed at the JCS meeting 'Imaging Cell Dynamics'. The meeting was held from 14 - 17 May 2023 in Lisbon, Portugal and was organised by Erika Holzbaur, Jennifer Lippincott-Schwartz, Rob Parton and Michael Way.
List by | Helen Zenner |
9th International Symposium on the Biology of Vertebrate Sex Determination
This preList contains preprints discussed during the 9th International Symposium on the Biology of Vertebrate Sex Determination. This conference was held in Kona, Hawaii from April 17th to 21st 2023.
List by | Martin Estermann |
Alumni picks – preLights 5th Birthday
This preList contains preprints that were picked and highlighted by preLights Alumni - an initiative that was set up to mark preLights 5th birthday. More entries will follow throughout February and March 2023.
List by | Sergio Menchero et al. |
CellBio 2022 – An ASCB/EMBO Meeting
This preLists features preprints that were discussed and presented during the CellBio 2022 meeting in Washington, DC in December 2022.
List by | Nadja Hümpfer et al. |
2nd Conference of the Visegrád Group Society for Developmental Biology
Preprints from the 2nd Conference of the Visegrád Group Society for Developmental Biology (2-5 September, 2021, Szeged, Hungary)
List by | Nándor Lipták |
Fibroblasts
The advances in fibroblast biology preList explores the recent discoveries and preprints of the fibroblast world. Get ready to immerse yourself with this list created for fibroblasts aficionados and lovers, and beyond. Here, my goal is to include preprints of fibroblast biology, heterogeneity, fate, extracellular matrix, behavior, topography, single-cell atlases, spatial transcriptomics, and their matrix!
List by | Osvaldo Contreras |
EMBL Synthetic Morphogenesis: From Gene Circuits to Tissue Architecture (2021)
A list of preprints mentioned at the #EESmorphoG virtual meeting in 2021.
List by | Alex Eve |
EMBL Conference: From functional genomics to systems biology
Preprints presented at the virtual EMBL conference "from functional genomics and systems biology", 16-19 November 2020
List by | Jesus Victorino |
Single Cell Biology 2020
A list of preprints mentioned at the Wellcome Genome Campus Single Cell Biology 2020 meeting.
List by | Alex Eve |
Society for Developmental Biology 79th Annual Meeting
Preprints at SDB 2020
List by | Irepan Salvador-Martinez, Martin Estermann |
FENS 2020
A collection of preprints presented during the virtual meeting of the Federation of European Neuroscience Societies (FENS) in 2020
List by | Ana Dorrego-Rivas |
Planar Cell Polarity – PCP
This preList contains preprints about the latest findings on Planar Cell Polarity (PCP) in various model organisms at the molecular, cellular and tissue levels.
List by | Ana Dorrego-Rivas |
Cell Polarity
Recent research from the field of cell polarity is summarized in this list of preprints. It comprises of studies focusing on various forms of cell polarity ranging from epithelial polarity, planar cell polarity to front-to-rear polarity.
List by | Yamini Ravichandran |
TAGC 2020
Preprints recently presented at the virtual Allied Genetics Conference, April 22-26, 2020. #TAGC20
List by | Maiko Kitaoka et al. |
3D Gastruloids
A curated list of preprints related to Gastruloids (in vitro models of early development obtained by 3D aggregation of embryonic cells). Updated until July 2021.
List by | Paul Gerald L. Sanchez and Stefano Vianello |
ASCB EMBO Annual Meeting 2019
A collection of preprints presented at the 2019 ASCB EMBO Meeting in Washington, DC (December 7-11)
List by | Madhuja Samaddar et al. |
EDBC Alicante 2019
Preprints presented at the European Developmental Biology Congress (EDBC) in Alicante, October 23-26 2019.
List by | Sergio Menchero et al. |
EMBL Seeing is Believing – Imaging the Molecular Processes of Life
Preprints discussed at the 2019 edition of Seeing is Believing, at EMBL Heidelberg from the 9th-12th October 2019
List by | Dey Lab |
SDB 78th Annual Meeting 2019
A curation of the preprints presented at the SDB meeting in Boston, July 26-30 2019. The preList will be updated throughout the duration of the meeting.
List by | Alex Eve |
Lung Disease and Regeneration
This preprint list compiles highlights from the field of lung biology.
List by | Rob Hynds |
Young Embryologist Network Conference 2019
Preprints presented at the Young Embryologist Network 2019 conference, 13 May, The Francis Crick Institute, London
List by | Alex Eve |
Pattern formation during development
The aim of this preList is to integrate results about the mechanisms that govern patterning during development, from genes implicated in the processes to theoritical models of pattern formation in nature.
List by | Alexa Sadier |
BSCB/BSDB Annual Meeting 2019
Preprints presented at the BSCB/BSDB Annual Meeting 2019
List by | Dey Lab |
Zebrafish immunology
A compilation of cutting-edge research that uses the zebrafish as a model system to elucidate novel immunological mechanisms in health and disease.
List by | Shikha Nayar |
Also in the systems biology category:
‘In preprints’ from Development 2022-2023
A list of the preprints featured in Development's 'In preprints' articles between 2022-2023
List by | Alex Eve, Katherine Brown |
EMBL Synthetic Morphogenesis: From Gene Circuits to Tissue Architecture (2021)
A list of preprints mentioned at the #EESmorphoG virtual meeting in 2021.
List by | Alex Eve |
Single Cell Biology 2020
A list of preprints mentioned at the Wellcome Genome Campus Single Cell Biology 2020 meeting.
List by | Alex Eve |
ASCB EMBO Annual Meeting 2019
A collection of preprints presented at the 2019 ASCB EMBO Meeting in Washington, DC (December 7-11)
List by | Madhuja Samaddar et al. |
EMBL Seeing is Believing – Imaging the Molecular Processes of Life
Preprints discussed at the 2019 edition of Seeing is Believing, at EMBL Heidelberg from the 9th-12th October 2019
List by | Dey Lab |
Pattern formation during development
The aim of this preList is to integrate results about the mechanisms that govern patterning during development, from genes implicated in the processes to theoritical models of pattern formation in nature.
List by | Alexa Sadier |
5 years
Allon Klein
Thanks for a great summary of our paper! I can suggest some points related to your open questions:
Q1: How to barcode? Inducible transposable elements or lentiviral transduction? The transposable elements are fantastic for non-perturbative barcoding in vivo. Their limitation is that they don’t create a barcode expressed in mRNA. So they can’t be read out by single cell RNA-Seq. The lentiviral approach has the big disadvantage of requiring a massive perturbation (namely isolating the cells and infecting them in vitro), but it allows reading out a barcode by scRNA-Seq.
Q2: Thanks for the great suggestion to visualize clonal data using SPRING! We don’t yet have an “all in one” package that goes from raw clonal data to completed analyses on SPRING yet. It’s a great idea.