Systematic reconstruction of the cellular trajectories of mammalian embryogenesis
Posted on: 18 June 2021
Preprint posted on 9 June 2021
An scRNA-seq #subwaymap of cellular trajectories in early mouse development, presented in this new, exciting preprint by @CXchengxiangQIU et al.!
Selected by Bobby RanjanCategories: bioinformatics, developmental biology
Introduction
A fundamental goal of developmental biology is understanding the relationships between cell types during embryogenesis and the molecular programs that underlie their emergence. Single-cell RNA-seq data generated during implantation (Cheng et al. 2019; Mohammed et al. 2017), gastrulation (Pijuan-Sala et al. 2019) and organogenesis (Cao et al. 2019) allows us to span mouse embryo development from dozens (E3.5) to millions (E13.5) of cells. However, integrating these datasets has been challenging due to unwanted variation in the data originating from laboratory, protocol and batch-specific differences.
Approach
To correct for this non-biological variation between datasets, the authors leveraged an anchor-based batch correction strategy proposed by Stuart et al. (2019). Briefly, this approach defines data from one timepoint as the “query” dataset and data from an adjacent timepoint as the “reference” dataset. Nearest neighbour cell pairings were identified between the two datasets (called anchors) and the expression profiles of cells in the query dataset were “corrected” along these anchors to integrate them with cells in the reference dataset.
Next, to connect cell states between timepoints, a k-nearest neighbour (k-NN) based heuristic was used. For a given cell state at a timepoint, the 5 closest cells from the antecedent timepoint were identified. After bootstrapping with down-sampling, the median proportion of such neighbours derived from each potential antecedent cell state was calculated. This process was performed in a pairwise manner for all 18 adjacent pairings (19 timepoints) to connect cell states between adjacent developmental stages.
The result of this integration of cell states from different timepoints can be represented as a directed, acyclic graph (Figure 1c) where each node represents a cell state (annotated cluster) and the edge weight represents the proportion of the cell state that is likely to originate from the potential antecedent cell state (also known as pseudo-ancestor). The trajectories of mammalian embryogenesis (TOME) thus obtained are largely consistent with our contemporary understanding of mammalian development. This graph was interrogated to study the maintenance of cellular phylogenies and to identify the transcription factors (TFs) and cis-regulatory motifs involved in in vivo cell type specification.
This integration strategy was then extended to perform a cross-species comparison of mouse, zebrafish and frog embryogenesis. To facilitate its exploration, the authors created an interactive website on which the nodes and edges shown in Figure 1c can be navigated (https://chengxiangqiu.github.io/tome/).
Figure 1. Systematic reconstruction of the cellular trajectories of mouse embryogenesis. c) Directed acyclic graph showing inferred relationships between cell states across early mouse development.
Key Findings
TOME molecular trajectories can recapitulate cellular phylogenies, with some caveats. The TOME graph (Figure 1c) has the following properties:
- The graph largely respects germ layers. There are no edges between extraembryonic and embryonic cell states and relatively few edges between embryonic cell states of different germ layers, which is consistent with our understanding of mammalian development.
- 80% of cell types are strongly linked to a single pseudo-ancestor when they first appear, and generally respect established lineage relationships.
- More than one pseudo-ancestor could be assigned to a cell state in the following scenarios:
- A cell type persists and contributes to another cell type over several consecutive timepoints. For example, hemoendothelial progenitors are recurrently assigned as pseudo-ancestors of endothelial cells at E7.75-E8.25.
- These convergences could be the result of incomplete separation between highly related cell types, rather than ongoing differentiation. For example, the several edges between notochord and definitive endoderm.
- The cell type may actually have multiple origins. For example, the two subtypes of E9.5 osteoblast progenitors have edges back to both E8.5 neural crest and E8.5 paraxial mesoderm, consistent with the literature (Tani et al. 2020).
- True lineage relationships for a given cell state can be obscured by presence of a similar cell state at the preceding timepoint. These can partially be resolved by RNA velocity analysis on the cell types in focus.
- The reliance on discrete entities i.e. cell states obscures aspects of developmental biology that are inherently continuous.
Systematic nomination of key transcription factors for cell type specification. Key TF candidates for specifying each newly emerging cell type were heuristically defined as those that were significantly and specifically upregulated in the newly generated cell type with respect to the pseudo-ancestor. For each such candidate, a normalized fold-change-based score was computed. Figure 4d demonstrates selected cellular trajectories from TOME, decorated with the top 5 scoring candidate key TFs for each edge.
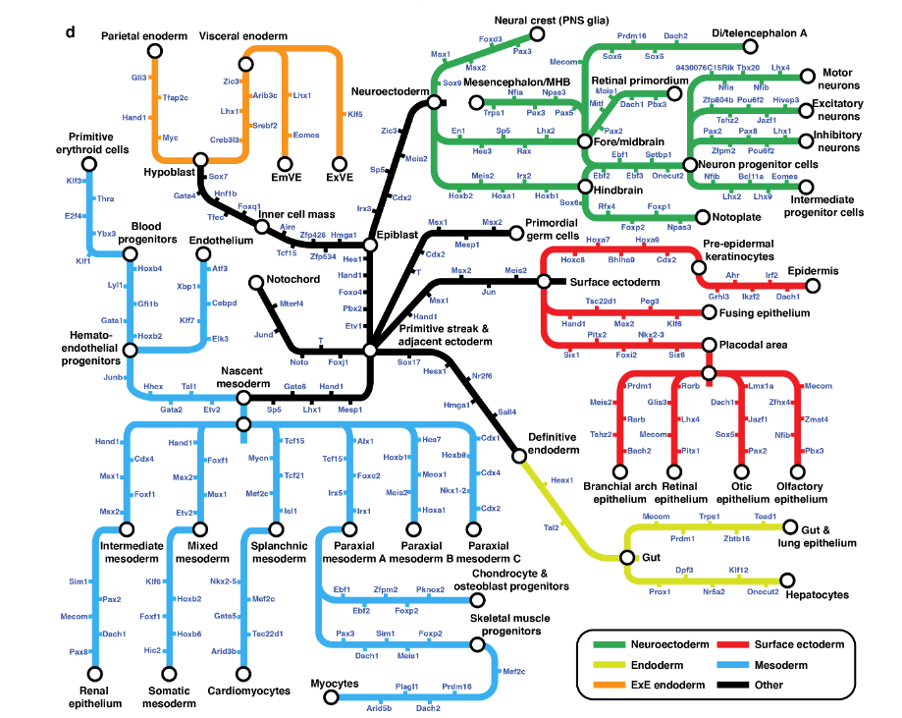
Identification of cis-regulatory motifs involved in in vivo cell type specification. The aforementioned TF selection approach was extended to identify all developmentally regulated genes. Using HOMER (Heinz et al. 2010), the authors discovered DNA sequence motifs that are specifically enriched in the core promoters of these key genes (-300 to +50 bp of TSS). At an FDR of 10%, 77 de novo and 100 previously documented promoter motifs were implicated in 41 and 30 mouse cell types respectively. Of these, 20 sequence motifs corresponded to binding sites of candidate key TFs for the same cell types, with 15 having consistent directionality between TF expression and target gene expression.
Systematic comparison of the cellular trajectories of mouse, zebrafish and frog embryogenesis. TOME was extended beyond the mammalian domain to facilitate systematic alignment of cell types across vertebrates. In zebrafish (D. rerio), data from 2 studies were integrated, together including 15 developmental stages (hpf3.3 – hpf 24). For frog (X. tropicalis), one dataset of 10 developmental stages (S8-S22) was analysed.
The authors used 3 strategies to systematically align cell types from each species to their cell type homologs in the other 2 species, which are detailed below:
- Each cell state at each timepoint was treated as a “pseudo-cell”, and pseudo-cells from all 3 species were integrated using anchor-based batch correction (Stuart et al. 2019). 15 major groups of pseudo-cells, each containing cell states from all 3 species, were identified. However, within each major group, the homology between specific cell types was generally ambiguous.
- Cell type correlation analysis via non-negative least squares (nnls) regression was performed to identify which cell types were the reciprocal best matches to one another. The highest ranking cell type pairings were manually reviewed for biological plausibility.
- Overlapping orthologous key candidate TFs between each possible interspecies cell type pairing were identified. Then, a permutation approach was adopted to identify instances in which an excess of orthologous candidate key TFs were shared between cell types. Finally, the cell type pairings were manually inspected for biological plausibility.
Overall, the authors were able to assign at least one cell type homolog to 48 of 77 embryonic mouse cell states, 52 of 59 zebrafish embryonic cell states, and 44 of 60 frog embryonic cell states.
Why I chose to highlight this preprint
TOME is the result of an elegant and systematic approach for constructing developmental trajectories, which provides us with a realistic strategy to fantasize about a complete integrated mammalian developmental roadmap in the future. While there are some caveats to the approach taken by the authors, this study is a considerable attempt to consolidate the numerous developmental scRNA-seq datasets in the field and provides readers with unique perspectives on integrating and analysing scRNA-seq data. In particular, the strategies described for cross-species comparisons can be transferred to study other evolutionarily conserved mechanisms. Thus, I believe this preprint is a valuable contribution to the field and will only increase in significance as more single-cell datasets continue to be generated.
References
- Cao, Junyue, Malte Spielmann, Xiaojie Qiu, Xingfan Huang, Daniel M. Ibrahim, Andrew J. Hill, Fan Zhang, et al. 2019. “The Single-Cell Transcriptional Landscape of Mammalian Organogenesis.” Nature 566 (7745): 496–502.
- Cheng, Shangli, Yu Pei, Liqun He, Guangdun Peng, Björn Reinius, Patrick P. L. Tam, Naihe Jing, and Qiaolin Deng. 2019. “Single-Cell RNA-Seq Reveals Cellular Heterogeneity of Pluripotency Transition and X Chromosome Dynamics during Early Mouse Development.” Cell Reports 26 (10): 2593–2607.e3.
- Heinz, Sven, Christopher Benner, Nathanael Spann, Eric Bertolino, Yin C. Lin, Peter Laslo, Jason X. Cheng, Cornelis Murre, Harinder Singh, and Christopher K. Glass. 2010. “Simple Combinations of Lineage-Determining Transcription Factors Prime Cis-Regulatory Elements Required for Macrophage and B Cell Identities.” Molecular Cell 38 (4): 576–89.
- Mohammed, Hisham, Irene Hernando-Herraez, Aurora Savino, Antonio Scialdone, Iain Macaulay, Carla Mulas, Tamir Chandra, et al. 2017. “Single-Cell Landscape of Transcriptional Heterogeneity and Cell Fate Decisions during Mouse Early Gastrulation.” Cell Reports 20 (5): 1215–28.
- Pijuan-Sala, Blanca, Jonathan A. Griffiths, Carolina Guibentif, Tom W. Hiscock, Wajid Jawaid, Fernando J. Calero-Nieto, Carla Mulas, et al. 2019. “A Single-Cell Molecular Map of Mouse Gastrulation and Early Organogenesis.” Nature 566 (7745): 490–95.
- Stuart, Tim, Andrew Butler, Paul Hoffman, Christoph Hafemeister, Efthymia Papalexi, William M. Mauck 3rd, Yuhan Hao, Marlon Stoeckius, Peter Smibert, and Rahul Satija. 2019. “Comprehensive Integration of Single-Cell Data.” Cell 177 (7): 1888–1902.e21.
- Tani, Shoichiro, Ung-Il Chung, Shinsuke Ohba, and Hironori Hojo. 2020. “Understanding Paraxial Mesoderm Development and Sclerotome Specification for Skeletal Repair.” Experimental & Molecular Medicine 52 (8): 1166–77.
doi: https://doi.org/10.1242/prelights.29626
Read preprintSign up to customise the site to your preferences and to receive alerts
Register hereAlso in the bioinformatics category:
Enhancer-driven cell type comparison reveals similarities between the mammalian and bird pallium
Rodrigo Senovilla-Ganzo

Expressive modeling and fast simulation for dynamic compartments
Benjamin Dominik Maier

Transcriptional profiling of human brain cortex identifies novel lncRNA-mediated networks dysregulated in amyotrophic lateral sclerosis
Julio Molina Pineda
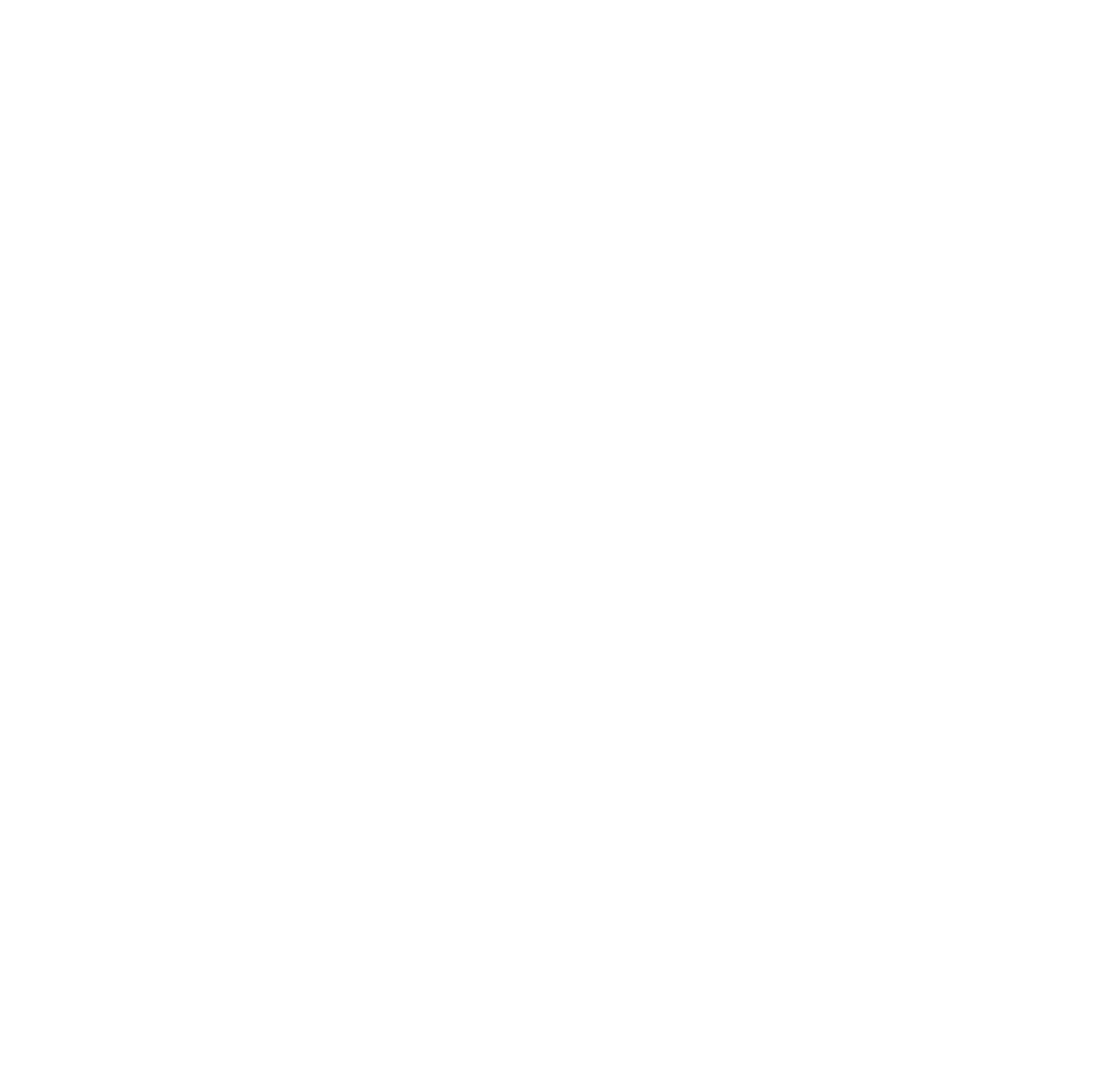
Also in the developmental biology category:
Gestational exposure to high heat-humidity conditions impairs mouse embryonic development
Girish Kale, preLights peer support

Modular control of time and space during vertebrate axis segmentation
AND
Natural genetic variation quantitatively regulates heart rate and dimension
Girish Kale, Jennifer Ann Black

Notch3 is a genetic modifier of NODAL signalling for patterning asymmetry during mouse heart looping
Bhaval Parmar

preListsbioinformatics category:
in the‘In preprints’ from Development 2022-2023
A list of the preprints featured in Development's 'In preprints' articles between 2022-2023
List by | Alex Eve, Katherine Brown |
9th International Symposium on the Biology of Vertebrate Sex Determination
This preList contains preprints discussed during the 9th International Symposium on the Biology of Vertebrate Sex Determination. This conference was held in Kona, Hawaii from April 17th to 21st 2023.
List by | Martin Estermann |
Alumni picks – preLights 5th Birthday
This preList contains preprints that were picked and highlighted by preLights Alumni - an initiative that was set up to mark preLights 5th birthday. More entries will follow throughout February and March 2023.
List by | Sergio Menchero et al. |
Fibroblasts
The advances in fibroblast biology preList explores the recent discoveries and preprints of the fibroblast world. Get ready to immerse yourself with this list created for fibroblasts aficionados and lovers, and beyond. Here, my goal is to include preprints of fibroblast biology, heterogeneity, fate, extracellular matrix, behavior, topography, single-cell atlases, spatial transcriptomics, and their matrix!
List by | Osvaldo Contreras |
Single Cell Biology 2020
A list of preprints mentioned at the Wellcome Genome Campus Single Cell Biology 2020 meeting.
List by | Alex Eve |
Antimicrobials: Discovery, clinical use, and development of resistance
Preprints that describe the discovery of new antimicrobials and any improvements made regarding their clinical use. Includes preprints that detail the factors affecting antimicrobial selection and the development of antimicrobial resistance.
List by | Zhang-He Goh |
Also in the developmental biology category:
BSDB/GenSoc Spring Meeting 2024
A list of preprints highlighted at the British Society for Developmental Biology and Genetics Society joint Spring meeting 2024 at Warwick, UK.
List by | Joyce Yu, Katherine Brown |
GfE/ DSDB meeting 2024
This preList highlights the preprints discussed at the 2024 joint German and Dutch developmental biology societies meeting that took place in March 2024 in Osnabrück, Germany.
List by | Joyce Yu |
‘In preprints’ from Development 2022-2023
A list of the preprints featured in Development's 'In preprints' articles between 2022-2023
List by | Alex Eve, Katherine Brown |
preLights peer support – preprints of interest
This is a preprint repository to organise the preprints and preLights covered through the 'preLights peer support' initiative.
List by | preLights peer support |
The Society for Developmental Biology 82nd Annual Meeting
This preList is made up of the preprints discussed during the Society for Developmental Biology 82nd Annual Meeting that took place in Chicago in July 2023.
List by | Joyce Yu, Katherine Brown |
CSHL 87th Symposium: Stem Cells
Preprints mentioned by speakers at the #CSHLsymp23
List by | Alex Eve |
Journal of Cell Science meeting ‘Imaging Cell Dynamics’
This preList highlights the preprints discussed at the JCS meeting 'Imaging Cell Dynamics'. The meeting was held from 14 - 17 May 2023 in Lisbon, Portugal and was organised by Erika Holzbaur, Jennifer Lippincott-Schwartz, Rob Parton and Michael Way.
List by | Helen Zenner |
9th International Symposium on the Biology of Vertebrate Sex Determination
This preList contains preprints discussed during the 9th International Symposium on the Biology of Vertebrate Sex Determination. This conference was held in Kona, Hawaii from April 17th to 21st 2023.
List by | Martin Estermann |
Alumni picks – preLights 5th Birthday
This preList contains preprints that were picked and highlighted by preLights Alumni - an initiative that was set up to mark preLights 5th birthday. More entries will follow throughout February and March 2023.
List by | Sergio Menchero et al. |
CellBio 2022 – An ASCB/EMBO Meeting
This preLists features preprints that were discussed and presented during the CellBio 2022 meeting in Washington, DC in December 2022.
List by | Nadja Hümpfer et al. |
2nd Conference of the Visegrád Group Society for Developmental Biology
Preprints from the 2nd Conference of the Visegrád Group Society for Developmental Biology (2-5 September, 2021, Szeged, Hungary)
List by | Nándor Lipták |
Fibroblasts
The advances in fibroblast biology preList explores the recent discoveries and preprints of the fibroblast world. Get ready to immerse yourself with this list created for fibroblasts aficionados and lovers, and beyond. Here, my goal is to include preprints of fibroblast biology, heterogeneity, fate, extracellular matrix, behavior, topography, single-cell atlases, spatial transcriptomics, and their matrix!
List by | Osvaldo Contreras |
EMBL Synthetic Morphogenesis: From Gene Circuits to Tissue Architecture (2021)
A list of preprints mentioned at the #EESmorphoG virtual meeting in 2021.
List by | Alex Eve |
EMBL Conference: From functional genomics to systems biology
Preprints presented at the virtual EMBL conference "from functional genomics and systems biology", 16-19 November 2020
List by | Jesus Victorino |
Single Cell Biology 2020
A list of preprints mentioned at the Wellcome Genome Campus Single Cell Biology 2020 meeting.
List by | Alex Eve |
Society for Developmental Biology 79th Annual Meeting
Preprints at SDB 2020
List by | Irepan Salvador-Martinez, Martin Estermann |
FENS 2020
A collection of preprints presented during the virtual meeting of the Federation of European Neuroscience Societies (FENS) in 2020
List by | Ana Dorrego-Rivas |
Planar Cell Polarity – PCP
This preList contains preprints about the latest findings on Planar Cell Polarity (PCP) in various model organisms at the molecular, cellular and tissue levels.
List by | Ana Dorrego-Rivas |
Cell Polarity
Recent research from the field of cell polarity is summarized in this list of preprints. It comprises of studies focusing on various forms of cell polarity ranging from epithelial polarity, planar cell polarity to front-to-rear polarity.
List by | Yamini Ravichandran |
TAGC 2020
Preprints recently presented at the virtual Allied Genetics Conference, April 22-26, 2020. #TAGC20
List by | Maiko Kitaoka et al. |
3D Gastruloids
A curated list of preprints related to Gastruloids (in vitro models of early development obtained by 3D aggregation of embryonic cells). Updated until July 2021.
List by | Paul Gerald L. Sanchez and Stefano Vianello |
ASCB EMBO Annual Meeting 2019
A collection of preprints presented at the 2019 ASCB EMBO Meeting in Washington, DC (December 7-11)
List by | Madhuja Samaddar et al. |
EDBC Alicante 2019
Preprints presented at the European Developmental Biology Congress (EDBC) in Alicante, October 23-26 2019.
List by | Sergio Menchero et al. |
EMBL Seeing is Believing – Imaging the Molecular Processes of Life
Preprints discussed at the 2019 edition of Seeing is Believing, at EMBL Heidelberg from the 9th-12th October 2019
List by | Dey Lab |
SDB 78th Annual Meeting 2019
A curation of the preprints presented at the SDB meeting in Boston, July 26-30 2019. The preList will be updated throughout the duration of the meeting.
List by | Alex Eve |
Lung Disease and Regeneration
This preprint list compiles highlights from the field of lung biology.
List by | Rob Hynds |
Young Embryologist Network Conference 2019
Preprints presented at the Young Embryologist Network 2019 conference, 13 May, The Francis Crick Institute, London
List by | Alex Eve |
Pattern formation during development
The aim of this preList is to integrate results about the mechanisms that govern patterning during development, from genes implicated in the processes to theoritical models of pattern formation in nature.
List by | Alexa Sadier |
BSCB/BSDB Annual Meeting 2019
Preprints presented at the BSCB/BSDB Annual Meeting 2019
List by | Dey Lab |
Zebrafish immunology
A compilation of cutting-edge research that uses the zebrafish as a model system to elucidate novel immunological mechanisms in health and disease.
List by | Shikha Nayar |