Generative modeling of single-cell population time series for inferring cell differentiation landscapes
Posted on: 6 November 2020
Preprint posted on 27 August 2020
Learn Waddington’s landscape and predict perturbed cell fate by computationally rolling cells on the landscape
Selected by Yen-Chung ChenCategories: bioinformatics, developmental biology, genomics, systems biology
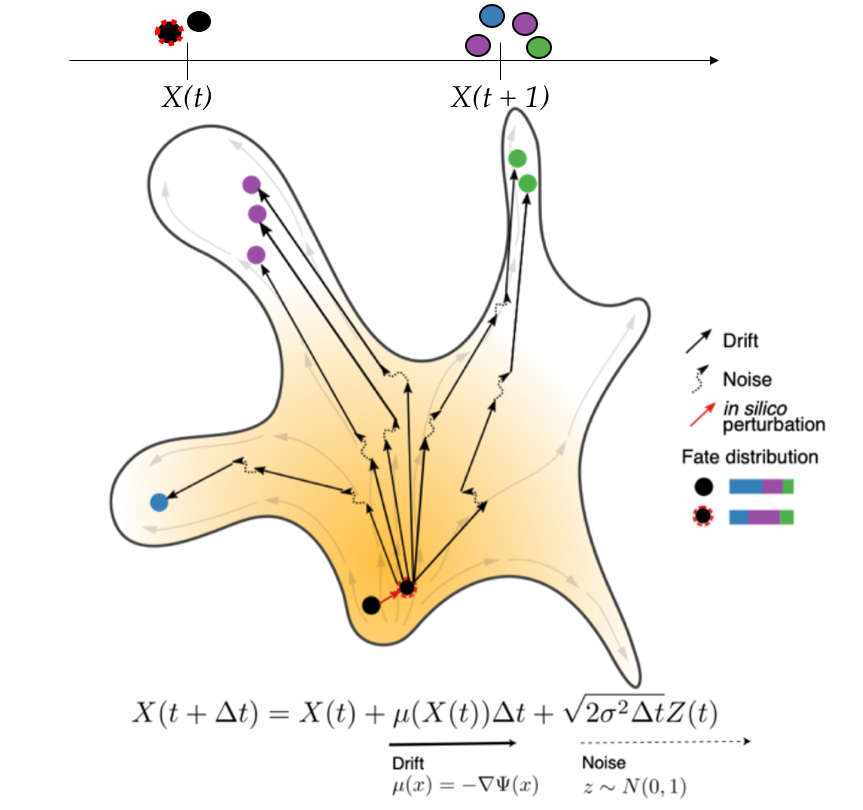
Background and context
How a zygote gives rise to countless types of cells forming different tissues and organs remains the greatest mystery of development. With recent technical advances, we are now able to observe the process of progenitor cells becoming mature cells with unprecedented resolution. In particular, single-cell RNA-seq allows the capture of gene expression of thousands of developing cells, so we can depict development as a gradual change of cellular states defined by all the genes expressed in the cell.
To become a mature cell, a progenitor cell is first specified so it becomes biased to undergo differentiation toward a specific cell type, or in other words, biased to accept a certain cell fate. The cell fate consolidates during development, meaning cells lose the ability to become other cell types even with perturbation. This model predicts that if transcriptomic profiling faithfully captures development, there must be an early state of gene expression that corresponds to specification in which the gene expression is sufficient to guide the cell to differentiate autonomously to a mature cell type. Similarly, there would be a later state following specification in which the change in gene expression restricts the developmental potential so the cell can no longer accept other fate even with genes are mis-expressed.
Transcriptomic profiling studies often aim to match single-cell transcriptomes to the stages of development and to link fate specification and commitment to gene expression. Many analysis methods summarize single-cell transcriptomes during development as a branching process and seek gene expression differences between the branches in the process [Reviewed in 1]; some other analyses try to couple earlier and later transcriptomes, and by comparing the early and later distribution of gene expression, we are able to learn the probability of a given early state giving rise to each cell type [2, 3].
Although the aforementioned techniques have shown their power in describing and identifying gene expression changes in development that are critical for the specification and commitment of cell fate, there are a few unresolved challenges. First, when samples are collected from different developmental stages, it is often difficult to integrate the “real time” that sample collection time represents into the analysis. Additionally, while the developmental process can be reconstructed, it is not straightforward to find genes that play larger roles in committing to a cell fate.
This preprint features a generative neural network that learns the developmental landscape from single-cell transcriptomes collected at different developmental stages. The model consists of the slope at each point on the landscape. Development of a cell is modeled by its movement on the landscape, and the direction and velocity is determined by the slope and a random noise. With this model, it is possible to pick an arbitrary transcriptome on the landscape and simulate its future developmental trajectory.
Key findings
PRESCIENT predicts unseen intermediate cell states
The model (Potential eneRgy undErlying Single Cell gradIENTs, or PRESCIENT) was tested on a dataset of hematopoiesis [4], in which lineage information is provided so cell fate can be resolved by identifying cell lineages by genetically encoded “barcodes”. The barcoded cell fate information provided a gold standard to evaluate the performance of the model. When trained only on day 2 and day 6 of differentiation, PRESCIENT successfully predicted the unseen transcriptomes at day 4 with a smaller prediction error than the cell fate prediction method Waddington-OT [5].
PRESCIENT predicts cell fate from progenitor transcriptomes with greater power when proliferation information included
PRESCIENT was then trained on data at all time points and used to predict the cell fate from progenitor transcriptomes. The prediction is presented as fate bias, the probability of a given progenitor to become each cell type. Fate bias is estimated by simulating 2,000 trajectories with PRESCIENT to see the ratio of simulations ending up as either a neutrophil or monocyte. When cell proliferation information was not provided, PRESCIENT predicted fate with a similar power as existing methods including Waddington-OT and FateID, while PRESCIENT outperformed Waddington-OT and FateID with cell proliferation provided.
PRESCIENT provides a generalizable model that predicts cell fate on unseen data and identifies potential fate regulators
To test if PRESCIENT can be applied on unseen data, the authors compared its performance of fate prediction when trained only on a subset of data versus all data. Regardless of whether PRESCIENT was trained on all data or a subset of the data, the performance was similar.
The authors went on to ask whether PRESCIENT predicts the fate of a transcriptome in which the expression of known cell fate regulators were perturbed in silico. The authors altered the expression of genes known to promote neutrophil generation computationally, and provided the perturbed transcriptome to PRESCIENT. With over-expression of neutrophil promoting genes, PRESCIENT predicted an increase of production of neutrophils, while knocking down was predicted to decrease neutrophil production.
A similar simulation was performed for known monocyte promoting genes and on another dataset of pancreas development, and the predictions were consistent with the prior knowledge. Notably, in the pancreas dataset, PRESCIENT not only successfully predicted the fate bias from perturbation but also the competent stages (stages before commitment when the perturbation can change the cell fate).
Finally, the authors performed a computational perturbation of 200+ transcription factors in pancreas development to screen for those capable of inducing fate biases. The screen resulted in 28 candidates including known regulators of the specification alpha and beta cells.
Brief summary
Yeo and Saksena et al. developed PRESCIENT, which depicts development as a landscape on which the cell moves according to the slope and random noise. The slope of the development landscape is learned with a neural network trained on gene expression at different developmental stages after dimensionality reduction with principal component analysis. This approach utilizes the sample collection time to represent real developmental time and is not dependent on inferring developmental “psuedotime” from the profiled cells. The generative nature of PRESCIENT makes it not only capable of identifying the progenitor-progeny relationship within the cells profiled, but also predict the terminal fate of a virtual progenitor that could be perturbed computationally.
Open questions
- It appears that the prediction power varies with the structure of the neural network and regularization strength. Would you suggest optimize these parameters per experiment (like different types of tissue or species)?
- PRESCIENT is implemented in the PCA space to learn the potential function (representing the force acting on the cell on a developmental landscape). Is the efficiency of PRESCIENT sensitive to the choice of dimensionality reduction techniques (Like PCA vs ICA or manifold learning)? If so, is there a reason why PCA is preferred?
- PRESCIENT takes real time into consideration in an efficient way. Although this gives PRESCIENT more power, the information might be very hard to obtain in some context. For example, neurogenesis is asynchronous in many species, and progenitors and neurons can be collected together during a wide time window (around 4 days in mouse spinal cord for example [6]). Asynchronous differentiation might make real time to deviate from the developmental time of individual cells. Would PRESCIENT still be applicable in this scenario?
References
- Saelens, W., Cannoodt, R., Todorov, H., Saeys, Y. (2019). A comparison of single-cell trajectory inference methods Nature Biotechnology 37(5), 547-554. https://dx.doi.org/10.1038/s41587-019-0071-9
- Schiebinger, G., Shu, J., Tabaka, M., Cleary, B., Subramanian, V., Solomon, A., Gould, J., Liu, S., Lin, S., Berube, P., Lee, L., Chen, J., Brumbaugh, J., Rigollet, P., Hochedlinger, K., Jaenisch, R., Regev, A., Lander, E. (2019). Optimal-Transport Analysis of Single-Cell Gene Expression Identifies Developmental Trajectories in Reprogramming Cell 176(4), 928-943.e22. https://dx.doi.org/10.1016/j.cell.2019.01.006
- Weinreb, C., Wolock, S., Tusi, B., Socolovsky, M., Klein, A. (2018). Fundamental limits on dynamic inference from single-cell snapshots Proceedings of the National Academy of Sciences 115(10), 201714723. https://dx.doi.org/10.1073/pnas.1714723115
- Weinreb, C., Rodriguez-Fraticelli, A., Camargo, F., Klein, A. (2020). Lineage tracing on transcriptional landscapes links state to fate during differentiation. Science (New York, N.Y.) 367(6479), eaaw3381. https://dx.doi.org/10.1126/science.aaw3381
- Schiebinger, G., Shu, J., Tabaka, M., Cleary, B., Subramanian, V., Solomon, A., Gould, J., Liu, S., Lin, S., Berube, P., Lee, L., Chen, J., Brumbaugh, J., Rigollet, P., Hochedlinger, K., Jaenisch, R., Regev, A., Lander, E. (2019). Optimal-Transport Analysis of Single-Cell Gene Expression Identifies Developmental Trajectories in Reprogramming Cell 176(4), 928-943.e22. https://dx.doi.org/10.1016/j.cell.2019.01.006
- Delile, J., Rayon, T., Melchionda, M., Edwards, A., Briscoe, J., Sagner, A. (2019). Single cell transcriptomics reveals spatial and temporal dynamics of gene expression in the developing mouse spinal cord Development 146(12), dev173807. https://dx.doi.org/10.1242/dev.173807
doi: https://doi.org/10.1242/prelights.25656
Read preprintSign up to customise the site to your preferences and to receive alerts
Register hereAlso in the bioinformatics category:
Enhancer-driven cell type comparison reveals similarities between the mammalian and bird pallium
Rodrigo Senovilla-Ganzo

Expressive modeling and fast simulation for dynamic compartments
Benjamin Dominik Maier

Transcriptional profiling of human brain cortex identifies novel lncRNA-mediated networks dysregulated in amyotrophic lateral sclerosis
Julio Molina Pineda
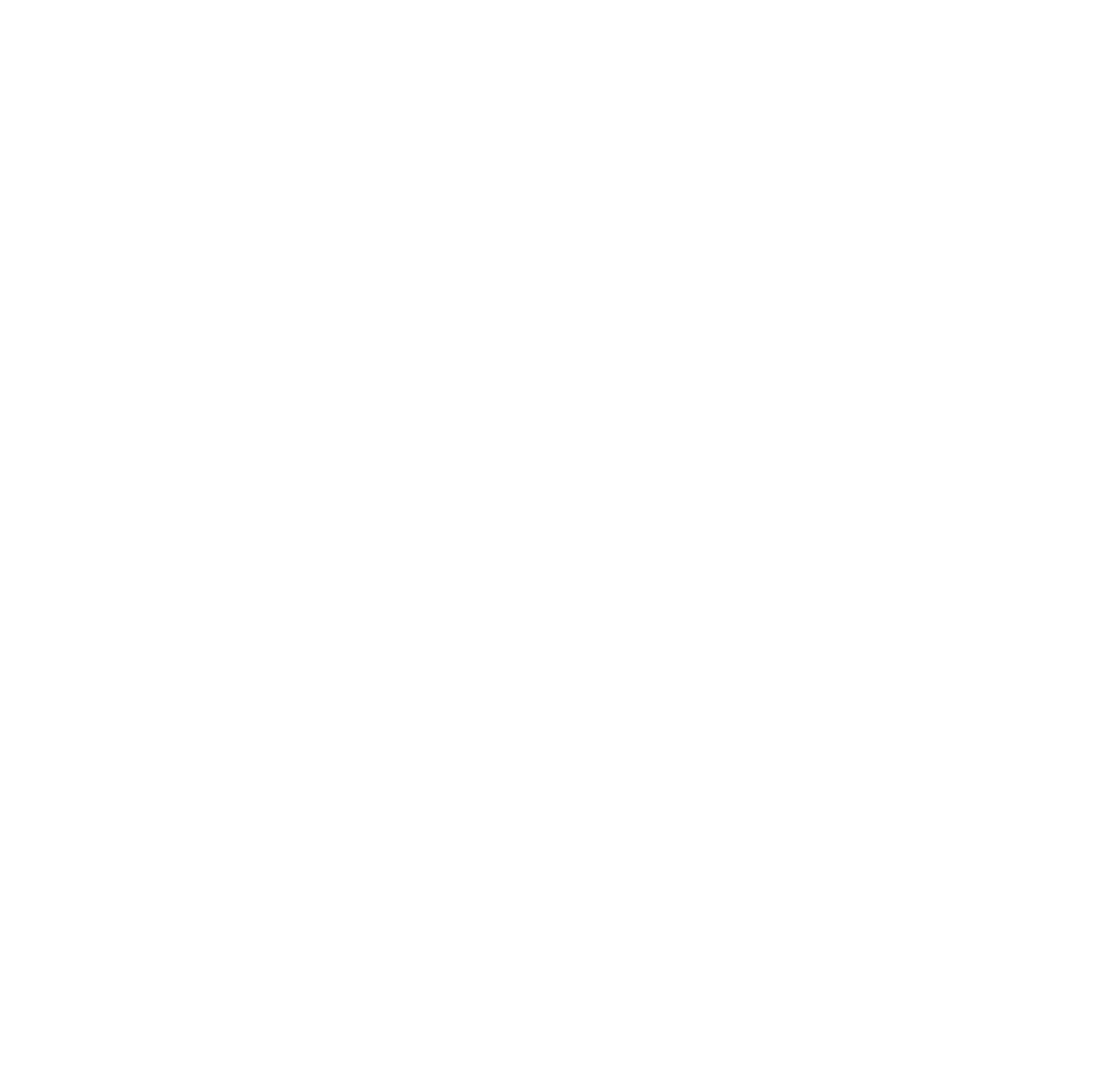
Also in the developmental biology category:
Gestational exposure to high heat-humidity conditions impairs mouse embryonic development
Girish Kale, preLights peer support

Modular control of time and space during vertebrate axis segmentation
AND
Natural genetic variation quantitatively regulates heart rate and dimension
Girish Kale, Jennifer Ann Black

Notch3 is a genetic modifier of NODAL signalling for patterning asymmetry during mouse heart looping
Bhaval Parmar

Also in the genomics category:
A fine kinetic balance of interactions directs transcription factor hubs to genes
Deevitha Balasubramanian
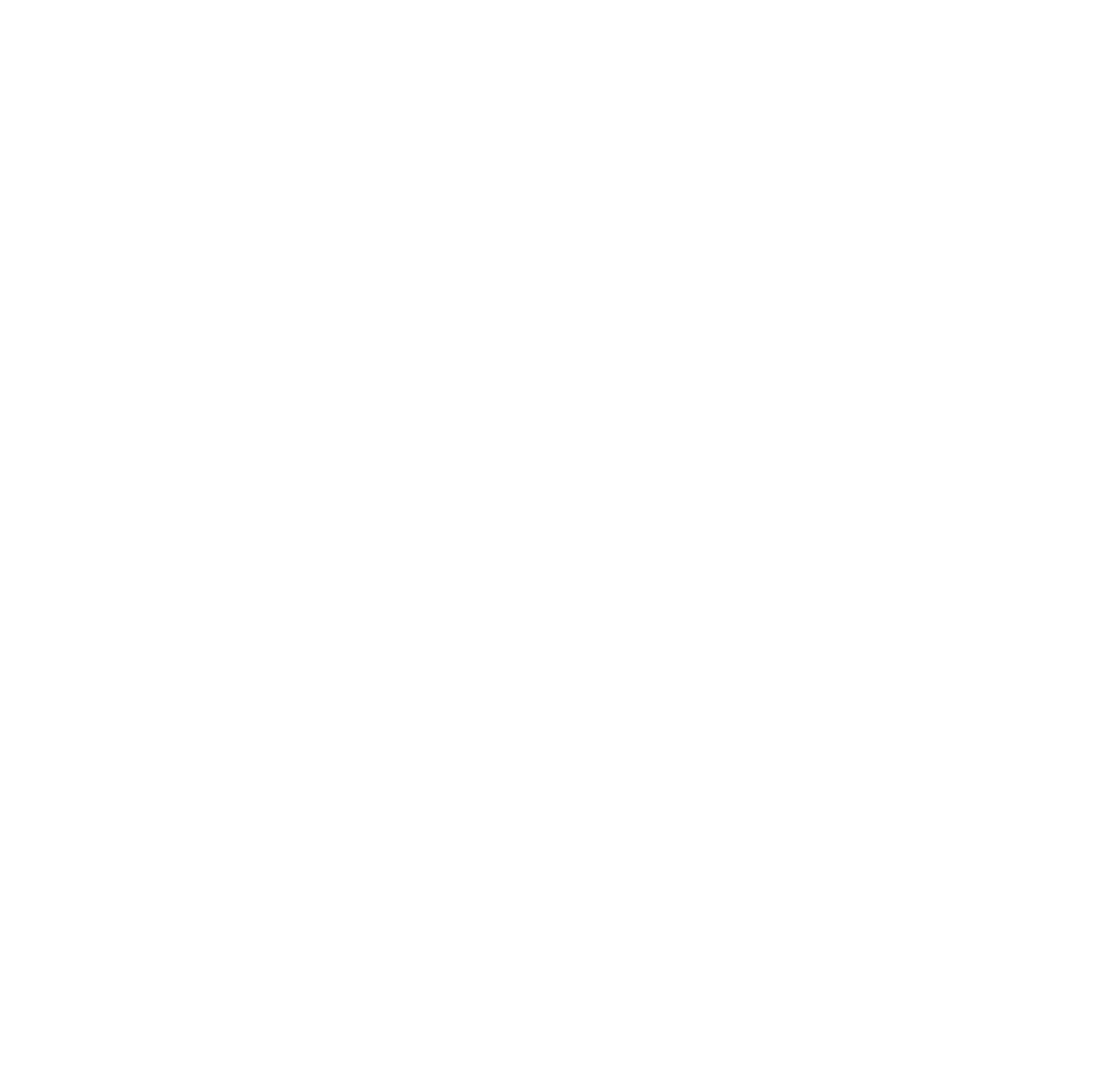
Enhancer-driven cell type comparison reveals similarities between the mammalian and bird pallium
Rodrigo Senovilla-Ganzo

Modular control of time and space during vertebrate axis segmentation
AND
Natural genetic variation quantitatively regulates heart rate and dimension
Girish Kale, Jennifer Ann Black

Also in the systems biology category:
Expressive modeling and fast simulation for dynamic compartments
Benjamin Dominik Maier

Clusters of lineage-specific genes are anchored by ZNF274 in repressive perinucleolar compartments
Silvia Carvalho

Holimap: an accurate and efficient method for solving stochastic gene network dynamics
Benjamin Dominik Maier

preListsbioinformatics category:
in the‘In preprints’ from Development 2022-2023
A list of the preprints featured in Development's 'In preprints' articles between 2022-2023
List by | Alex Eve, Katherine Brown |
9th International Symposium on the Biology of Vertebrate Sex Determination
This preList contains preprints discussed during the 9th International Symposium on the Biology of Vertebrate Sex Determination. This conference was held in Kona, Hawaii from April 17th to 21st 2023.
List by | Martin Estermann |
Alumni picks – preLights 5th Birthday
This preList contains preprints that were picked and highlighted by preLights Alumni - an initiative that was set up to mark preLights 5th birthday. More entries will follow throughout February and March 2023.
List by | Sergio Menchero et al. |
Fibroblasts
The advances in fibroblast biology preList explores the recent discoveries and preprints of the fibroblast world. Get ready to immerse yourself with this list created for fibroblasts aficionados and lovers, and beyond. Here, my goal is to include preprints of fibroblast biology, heterogeneity, fate, extracellular matrix, behavior, topography, single-cell atlases, spatial transcriptomics, and their matrix!
List by | Osvaldo Contreras |
Single Cell Biology 2020
A list of preprints mentioned at the Wellcome Genome Campus Single Cell Biology 2020 meeting.
List by | Alex Eve |
Antimicrobials: Discovery, clinical use, and development of resistance
Preprints that describe the discovery of new antimicrobials and any improvements made regarding their clinical use. Includes preprints that detail the factors affecting antimicrobial selection and the development of antimicrobial resistance.
List by | Zhang-He Goh |
Also in the developmental biology category:
BSDB/GenSoc Spring Meeting 2024
A list of preprints highlighted at the British Society for Developmental Biology and Genetics Society joint Spring meeting 2024 at Warwick, UK.
List by | Joyce Yu, Katherine Brown |
GfE/ DSDB meeting 2024
This preList highlights the preprints discussed at the 2024 joint German and Dutch developmental biology societies meeting that took place in March 2024 in Osnabrück, Germany.
List by | Joyce Yu |
‘In preprints’ from Development 2022-2023
A list of the preprints featured in Development's 'In preprints' articles between 2022-2023
List by | Alex Eve, Katherine Brown |
preLights peer support – preprints of interest
This is a preprint repository to organise the preprints and preLights covered through the 'preLights peer support' initiative.
List by | preLights peer support |
The Society for Developmental Biology 82nd Annual Meeting
This preList is made up of the preprints discussed during the Society for Developmental Biology 82nd Annual Meeting that took place in Chicago in July 2023.
List by | Joyce Yu, Katherine Brown |
CSHL 87th Symposium: Stem Cells
Preprints mentioned by speakers at the #CSHLsymp23
List by | Alex Eve |
Journal of Cell Science meeting ‘Imaging Cell Dynamics’
This preList highlights the preprints discussed at the JCS meeting 'Imaging Cell Dynamics'. The meeting was held from 14 - 17 May 2023 in Lisbon, Portugal and was organised by Erika Holzbaur, Jennifer Lippincott-Schwartz, Rob Parton and Michael Way.
List by | Helen Zenner |
9th International Symposium on the Biology of Vertebrate Sex Determination
This preList contains preprints discussed during the 9th International Symposium on the Biology of Vertebrate Sex Determination. This conference was held in Kona, Hawaii from April 17th to 21st 2023.
List by | Martin Estermann |
Alumni picks – preLights 5th Birthday
This preList contains preprints that were picked and highlighted by preLights Alumni - an initiative that was set up to mark preLights 5th birthday. More entries will follow throughout February and March 2023.
List by | Sergio Menchero et al. |
CellBio 2022 – An ASCB/EMBO Meeting
This preLists features preprints that were discussed and presented during the CellBio 2022 meeting in Washington, DC in December 2022.
List by | Nadja Hümpfer et al. |
2nd Conference of the Visegrád Group Society for Developmental Biology
Preprints from the 2nd Conference of the Visegrád Group Society for Developmental Biology (2-5 September, 2021, Szeged, Hungary)
List by | Nándor Lipták |
Fibroblasts
The advances in fibroblast biology preList explores the recent discoveries and preprints of the fibroblast world. Get ready to immerse yourself with this list created for fibroblasts aficionados and lovers, and beyond. Here, my goal is to include preprints of fibroblast biology, heterogeneity, fate, extracellular matrix, behavior, topography, single-cell atlases, spatial transcriptomics, and their matrix!
List by | Osvaldo Contreras |
EMBL Synthetic Morphogenesis: From Gene Circuits to Tissue Architecture (2021)
A list of preprints mentioned at the #EESmorphoG virtual meeting in 2021.
List by | Alex Eve |
EMBL Conference: From functional genomics to systems biology
Preprints presented at the virtual EMBL conference "from functional genomics and systems biology", 16-19 November 2020
List by | Jesus Victorino |
Single Cell Biology 2020
A list of preprints mentioned at the Wellcome Genome Campus Single Cell Biology 2020 meeting.
List by | Alex Eve |
Society for Developmental Biology 79th Annual Meeting
Preprints at SDB 2020
List by | Irepan Salvador-Martinez, Martin Estermann |
FENS 2020
A collection of preprints presented during the virtual meeting of the Federation of European Neuroscience Societies (FENS) in 2020
List by | Ana Dorrego-Rivas |
Planar Cell Polarity – PCP
This preList contains preprints about the latest findings on Planar Cell Polarity (PCP) in various model organisms at the molecular, cellular and tissue levels.
List by | Ana Dorrego-Rivas |
Cell Polarity
Recent research from the field of cell polarity is summarized in this list of preprints. It comprises of studies focusing on various forms of cell polarity ranging from epithelial polarity, planar cell polarity to front-to-rear polarity.
List by | Yamini Ravichandran |
TAGC 2020
Preprints recently presented at the virtual Allied Genetics Conference, April 22-26, 2020. #TAGC20
List by | Maiko Kitaoka et al. |
3D Gastruloids
A curated list of preprints related to Gastruloids (in vitro models of early development obtained by 3D aggregation of embryonic cells). Updated until July 2021.
List by | Paul Gerald L. Sanchez and Stefano Vianello |
ASCB EMBO Annual Meeting 2019
A collection of preprints presented at the 2019 ASCB EMBO Meeting in Washington, DC (December 7-11)
List by | Madhuja Samaddar et al. |
EDBC Alicante 2019
Preprints presented at the European Developmental Biology Congress (EDBC) in Alicante, October 23-26 2019.
List by | Sergio Menchero et al. |
EMBL Seeing is Believing – Imaging the Molecular Processes of Life
Preprints discussed at the 2019 edition of Seeing is Believing, at EMBL Heidelberg from the 9th-12th October 2019
List by | Dey Lab |
SDB 78th Annual Meeting 2019
A curation of the preprints presented at the SDB meeting in Boston, July 26-30 2019. The preList will be updated throughout the duration of the meeting.
List by | Alex Eve |
Lung Disease and Regeneration
This preprint list compiles highlights from the field of lung biology.
List by | Rob Hynds |
Young Embryologist Network Conference 2019
Preprints presented at the Young Embryologist Network 2019 conference, 13 May, The Francis Crick Institute, London
List by | Alex Eve |
Pattern formation during development
The aim of this preList is to integrate results about the mechanisms that govern patterning during development, from genes implicated in the processes to theoritical models of pattern formation in nature.
List by | Alexa Sadier |
BSCB/BSDB Annual Meeting 2019
Preprints presented at the BSCB/BSDB Annual Meeting 2019
List by | Dey Lab |
Zebrafish immunology
A compilation of cutting-edge research that uses the zebrafish as a model system to elucidate novel immunological mechanisms in health and disease.
List by | Shikha Nayar |
Also in the genomics category:
BSCB-Biochemical Society 2024 Cell Migration meeting
This preList features preprints that were discussed and presented during the BSCB-Biochemical Society 2024 Cell Migration meeting in Birmingham, UK in April 2024. Kindly put together by Sara Morais da Silva, Reviews Editor at Journal of Cell Science.
List by | Reinier Prosee |
9th International Symposium on the Biology of Vertebrate Sex Determination
This preList contains preprints discussed during the 9th International Symposium on the Biology of Vertebrate Sex Determination. This conference was held in Kona, Hawaii from April 17th to 21st 2023.
List by | Martin Estermann |
Semmelweis Symposium 2022: 40th anniversary of international medical education at Semmelweis University
This preList contains preprints discussed during the 'Semmelweis Symposium 2022' (7-9 November), organised around the 40th anniversary of international medical education at Semmelweis University covering a wide range of topics.
List by | Nándor Lipták |
20th “Genetics Workshops in Hungary”, Szeged (25th, September)
In this annual conference, Hungarian geneticists, biochemists and biotechnologists presented their works. Link: http://group.szbk.u-szeged.hu/minikonf/archive/prg2021.pdf
List by | Nándor Lipták |
EMBL Conference: From functional genomics to systems biology
Preprints presented at the virtual EMBL conference "from functional genomics and systems biology", 16-19 November 2020
List by | Jesus Victorino |
TAGC 2020
Preprints recently presented at the virtual Allied Genetics Conference, April 22-26, 2020. #TAGC20
List by | Maiko Kitaoka et al. |
Also in the systems biology category:
‘In preprints’ from Development 2022-2023
A list of the preprints featured in Development's 'In preprints' articles between 2022-2023
List by | Alex Eve, Katherine Brown |
EMBL Synthetic Morphogenesis: From Gene Circuits to Tissue Architecture (2021)
A list of preprints mentioned at the #EESmorphoG virtual meeting in 2021.
List by | Alex Eve |
Single Cell Biology 2020
A list of preprints mentioned at the Wellcome Genome Campus Single Cell Biology 2020 meeting.
List by | Alex Eve |
ASCB EMBO Annual Meeting 2019
A collection of preprints presented at the 2019 ASCB EMBO Meeting in Washington, DC (December 7-11)
List by | Madhuja Samaddar et al. |
EMBL Seeing is Believing – Imaging the Molecular Processes of Life
Preprints discussed at the 2019 edition of Seeing is Believing, at EMBL Heidelberg from the 9th-12th October 2019
List by | Dey Lab |
Pattern formation during development
The aim of this preList is to integrate results about the mechanisms that govern patterning during development, from genes implicated in the processes to theoritical models of pattern formation in nature.
List by | Alexa Sadier |