Deep Learning Enables Individual Xenograft Cell Classification in Histological Images by Analysis of Contextual Features
Posted on: 15 December 2020
Preprint posted on 4 November 2020
Article now published in Journal of Mammary Gland Biology and Neoplasia at http://dx.doi.org/10.1007/s10911-021-09485-4
Categories: cell biology
Background
Breast cancer is the most commonly diagnosed tumour in women, and its incidence worldwide has seen important increases over time. Most of our understanding of mammary gland development and breast carcinogenesis has derived from experiments in animal models, with mice being the most commonly used model. Nevertheless, a high proportion of potential oncology drugs fail in clinical trials, partly because of probable differences and incompatibilities between the rodent models and humans, raising concerns over the suitability of rodent models for some questions of cancer research. To address this potential gap, patient derived xenografts (PDXs) have emerged as an interesting tool. PDXs are preclinical models developed by transplanting human-derived cells into immunosuppressed or humanized mice, and various studies have shown that they successfully recapitulate the complexity of human tissues, which has resulted in this model gaining momentum for translational research. A further advance overcoming some caveats in PDXs is the Mouse IntraDuctal Model (MIND), which consists on the injection of primary human-derived breast cells (HDBCs) directly into the mouse mammary ductal tree via cleaved teat. In the intraductal microenvironment HDBCs and breast cancer cells grow independently of any hormone supplementations, while retaining hormone responsiveness and hormone receptor signature. A caveat of PDXs and MIND models is that it is important to discriminate between xenografted and host cells, but histological analyses of the resulting tissues are usually confounded by the presence of cells of the different species, which can give rise to data misinterpretation. To circumvent this hurdle, Juppet et al (1) developed a Single Cell Classifier (SCC), a data-driven deep learning-based computational tool which allows performing automated species classification of individual cells in H&E stained sections. It is available as a plugin in ImageJ/Fiji, and can be downloaded at the link: https://github.com/Biomedical-Imaging-Group/SingleCellClassifier.
.
Key findings and developments
Method development for cell classification
Slides of tissue sections prepared for H&E staining were scanned using an Olympus VS120-L100 slide scanner with a 20x/0.75 objective connected to a colour camera. The resulting images were loaded into QuPath, and are publicly available in Zenodo (https://zenodo.org/record/3960270).
The authors then developed a method for data extraction, whereby a version of each image was extracted to define areas likely to contain ducts- defined as large, densely packed cell regions having a dense Eosin signal. This information was used to extract them using Fiji’s Color Deconvolution with the built-in “H&E DAB” vectors. The extracted signal was filtered before thresholding with ImageJ’s Default method. Finally, a connected components analysis (AnalyzeParticles) was used to obtain ROIs. The bounding boxes of these ROIs were extracted and enlarged so that no ducts were touching the edge of the image.
To define boundaries between neighbouring cells, nuclei segmentation was used as a first step, using StarDist. A StarDist model was trained with multiple images extracted from H&E-stained sections of normal human breast xenografted mammary glands. Following this, the estimation of the cells was computed according to two criteria (which can be edited according to user needs: a) cells should not overlap with each other, and b) their thickness (i.e. distance to the nuclei) should not exceed 2µm). These criteria can be represented as masks that can be integrated to delineate each cell. Following this, 484 features (were extracted from the detected nuclei and cells. These included 47 cell-intrinsic features such as shape and size, area, ratio between nuclei and cells, colour-related features (eg. mean variation of each channel), and texture-related features (eg. entropy and contrast). It also included 376 contextual features, (i.e. features of the neighbouring cells), and 61 features related to the organization of the neighbours. Altogether these features included position of a given cell within a cluster given their mean and variance of their distance, defining borders, alignment, orientation, whether the cells were connected to a chain of cells, tortuosity, and size, and shape of the cluster. What motivated the extraction of contextual features was evidence that cells that are physically connected share common features.
To classify the cells based on their features, the authors trained a neural network model by supervised learning using Python. Multiple images were extracted from H&E-stained xenografted mouse mammary glands, containing either only human or only mouse cells. A human-specific E-cadherin antibody was used to uniquely probe for xenografted non-malignant cells. Tumour cells were uniquely detected using a human-specific cytokeratin 7 (CK7) antibody.
An ImageJ/Fiji plugin names “Single Cell Classifier” was implemented to allow users to a) perform classifications using the methods previously described and b) adopt other models in addition to the built-in ones, and/or edit the parameters of the methods in a manner that is user-specific. The plugins depend on 3 other plugins: StarDist, MorphoLibJ and CSBDeep (the latter of which executes the classification neural network).
Classification of normal and malignant tissues
The authors went on to characterize the impact of each feature on the neural network, to assess the morphology of the engrafted human cells in the intraductal environment. Shape and size of individual cells poorly discriminated between human and mouse cells, however, the contextual features for shape and size achieved a better correlation. Moreover, texture features seemed to offer better help for discriminating cell species than shape and size. Yet, contextual features without any texture information still allowed for classification with almost 90% accuracy (while shape and size alone reached almost 67% accuracy). The SCC reached up to 96% classification accuracy between both species in both normal and malignant tissues, across different breast cancer subtypes. They overall conclude that SCC will facilitate the interpretation of H&E stained histological sections in an automated manner, and that it has the advantage that it allows the implementation of new in-house built models for further classification tasks for these or other applications.
What I like about this preprint
I like that the authors provide a novel tool to bridge a gap within histology, which is a very broad field with impact on many scientific disciplines. I like that the authors made everything open, which allows for other scientists to apply and/or adapt the model to their own needs. This is consistent with the principles of open access and open science, which I (like many other scientists), favour.
Open questions
- You mention that texture-specific features allowed reaching the highest accuracy. What was the limitation of colour-specific features, particularly if using species-specific antibodies?
- Your SCC development seems really an excellent tool. What would be further limitations you identified, that authors should be aware of? You made the tool open and editable to adapt for user-specific needs, therefore, what would be specific points you would suggest users to be aware of while considering editing different parameters?
- You tested and trained the SCC in your work on breast cancer models, allowing you to differentiate between cancer subtypes, and between normal and malignant tissue. How easily applicable are the same parameters to other types of xenograft and other types of cancer modelled in a similar manner in mice?
- Being a very useful tool, it wouldn’t be surprising if it were also useful to other model organisms. Would you expect much changes would be needed in the adaptation of the SCC for different organisms (than the mouse/human combination you developed it originally for)?
References
- Juppet et al, Deep learning enables individual xenograft cell classification in histological images by analysis of contextual features, bioRxiv,2020.
doi: https://doi.org/10.1242/prelights.26466
Read preprintSign up to customise the site to your preferences and to receive alerts
Register hereAlso in the cell biology category:
Germplasm stability in zebrafish requires maternal Tdrd6a and Tdrd6c
Justin Gutkowski

Leukocytes use endothelial membrane tunnels to extravasate the vasculature
Felipe Del Valle Batalla

Platelet-derived LPA16:0 inhibits adult neurogenesis and stress resilience in anxiety disorder
Harvey Roweth
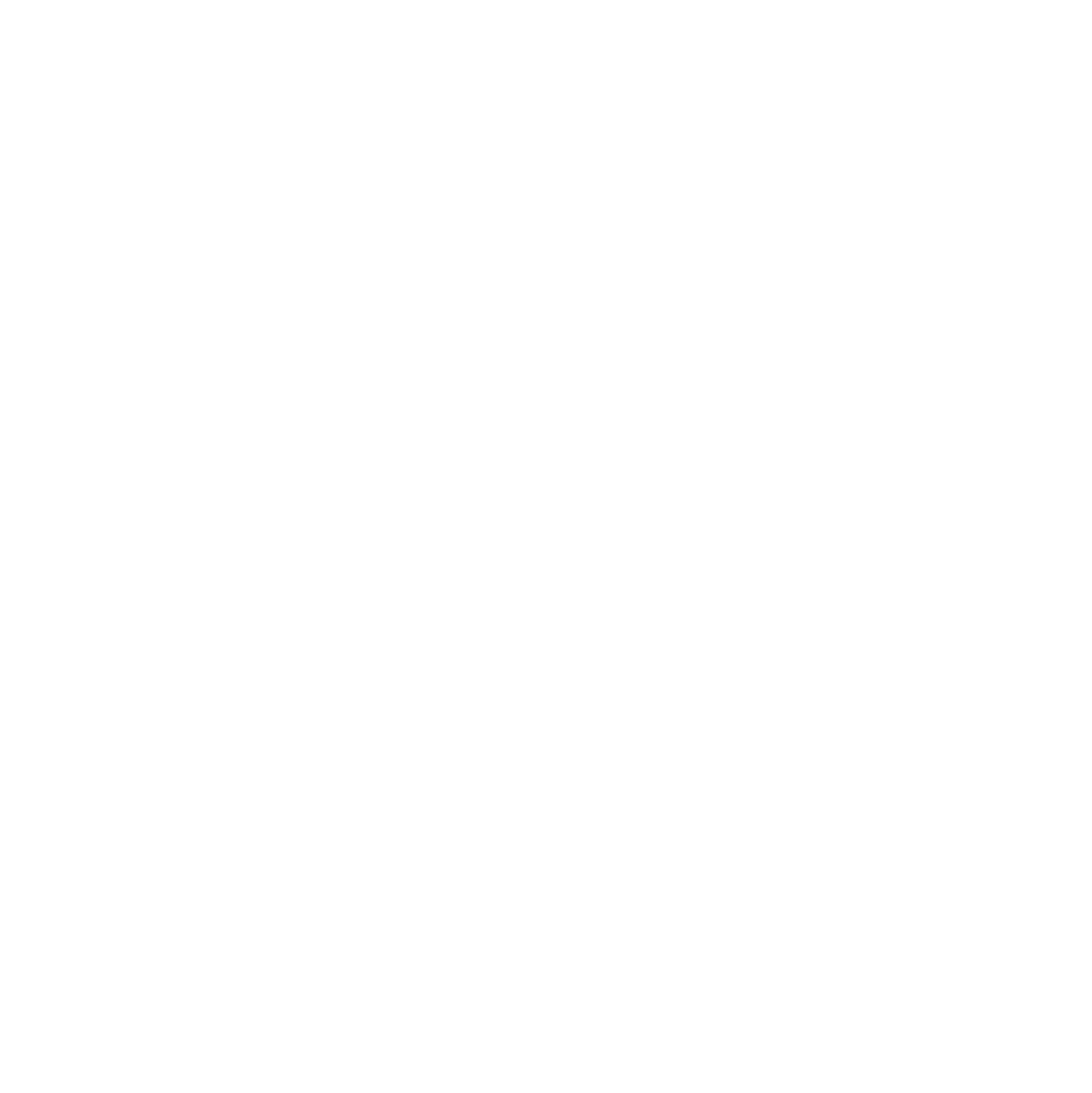
preListscell biology category:
in theNovember in preprints – the CellBio edition
This is the first community-driven preList! A group of preLighters, with expertise in different areas of cell biology, have worked together to create this preprint reading lists for researchers with an interest in cell biology. Categories include: 1) cancer cell biology 2) cell cycle and division 3) cell migration and cytoskeleton 4) cell organelles and organisation 5) cell signalling and mechanosensing 6) genetics/gene expression
List by | Felipe Del Valle Batalla et al. |
BSCB-Biochemical Society 2024 Cell Migration meeting
This preList features preprints that were discussed and presented during the BSCB-Biochemical Society 2024 Cell Migration meeting in Birmingham, UK in April 2024. Kindly put together by Sara Morais da Silva, Reviews Editor at Journal of Cell Science.
List by | Reinier Prosee |
‘In preprints’ from Development 2022-2023
A list of the preprints featured in Development's 'In preprints' articles between 2022-2023
List by | Alex Eve, Katherine Brown |
preLights peer support – preprints of interest
This is a preprint repository to organise the preprints and preLights covered through the 'preLights peer support' initiative.
List by | preLights peer support |
The Society for Developmental Biology 82nd Annual Meeting
This preList is made up of the preprints discussed during the Society for Developmental Biology 82nd Annual Meeting that took place in Chicago in July 2023.
List by | Joyce Yu, Katherine Brown |
CSHL 87th Symposium: Stem Cells
Preprints mentioned by speakers at the #CSHLsymp23
List by | Alex Eve |
Journal of Cell Science meeting ‘Imaging Cell Dynamics’
This preList highlights the preprints discussed at the JCS meeting 'Imaging Cell Dynamics'. The meeting was held from 14 - 17 May 2023 in Lisbon, Portugal and was organised by Erika Holzbaur, Jennifer Lippincott-Schwartz, Rob Parton and Michael Way.
List by | Helen Zenner |
9th International Symposium on the Biology of Vertebrate Sex Determination
This preList contains preprints discussed during the 9th International Symposium on the Biology of Vertebrate Sex Determination. This conference was held in Kona, Hawaii from April 17th to 21st 2023.
List by | Martin Estermann |
Alumni picks – preLights 5th Birthday
This preList contains preprints that were picked and highlighted by preLights Alumni - an initiative that was set up to mark preLights 5th birthday. More entries will follow throughout February and March 2023.
List by | Sergio Menchero et al. |
CellBio 2022 – An ASCB/EMBO Meeting
This preLists features preprints that were discussed and presented during the CellBio 2022 meeting in Washington, DC in December 2022.
List by | Nadja Hümpfer et al. |
Fibroblasts
The advances in fibroblast biology preList explores the recent discoveries and preprints of the fibroblast world. Get ready to immerse yourself with this list created for fibroblasts aficionados and lovers, and beyond. Here, my goal is to include preprints of fibroblast biology, heterogeneity, fate, extracellular matrix, behavior, topography, single-cell atlases, spatial transcriptomics, and their matrix!
List by | Osvaldo Contreras |
EMBL Synthetic Morphogenesis: From Gene Circuits to Tissue Architecture (2021)
A list of preprints mentioned at the #EESmorphoG virtual meeting in 2021.
List by | Alex Eve |
FENS 2020
A collection of preprints presented during the virtual meeting of the Federation of European Neuroscience Societies (FENS) in 2020
List by | Ana Dorrego-Rivas |
Planar Cell Polarity – PCP
This preList contains preprints about the latest findings on Planar Cell Polarity (PCP) in various model organisms at the molecular, cellular and tissue levels.
List by | Ana Dorrego-Rivas |
BioMalPar XVI: Biology and Pathology of the Malaria Parasite
[under construction] Preprints presented at the (fully virtual) EMBL BioMalPar XVI, 17-18 May 2020 #emblmalaria
List by | Dey Lab, Samantha Seah |
1
Cell Polarity
Recent research from the field of cell polarity is summarized in this list of preprints. It comprises of studies focusing on various forms of cell polarity ranging from epithelial polarity, planar cell polarity to front-to-rear polarity.
List by | Yamini Ravichandran |
TAGC 2020
Preprints recently presented at the virtual Allied Genetics Conference, April 22-26, 2020. #TAGC20
List by | Maiko Kitaoka et al. |
3D Gastruloids
A curated list of preprints related to Gastruloids (in vitro models of early development obtained by 3D aggregation of embryonic cells). Updated until July 2021.
List by | Paul Gerald L. Sanchez and Stefano Vianello |
ECFG15 – Fungal biology
Preprints presented at 15th European Conference on Fungal Genetics 17-20 February 2020 Rome
List by | Hiral Shah |
ASCB EMBO Annual Meeting 2019
A collection of preprints presented at the 2019 ASCB EMBO Meeting in Washington, DC (December 7-11)
List by | Madhuja Samaddar et al. |
EMBL Seeing is Believing – Imaging the Molecular Processes of Life
Preprints discussed at the 2019 edition of Seeing is Believing, at EMBL Heidelberg from the 9th-12th October 2019
List by | Dey Lab |
Autophagy
Preprints on autophagy and lysosomal degradation and its role in neurodegeneration and disease. Includes molecular mechanisms, upstream signalling and regulation as well as studies on pharmaceutical interventions to upregulate the process.
List by | Sandra Malmgren Hill |
Lung Disease and Regeneration
This preprint list compiles highlights from the field of lung biology.
List by | Rob Hynds |
Cellular metabolism
A curated list of preprints related to cellular metabolism at Biorxiv by Pablo Ranea Robles from the Prelights community. Special interest on lipid metabolism, peroxisomes and mitochondria.
List by | Pablo Ranea Robles |
BSCB/BSDB Annual Meeting 2019
Preprints presented at the BSCB/BSDB Annual Meeting 2019
List by | Dey Lab |
MitoList
This list of preprints is focused on work expanding our knowledge on mitochondria in any organism, tissue or cell type, from the normal biology to the pathology.
List by | Sandra Franco Iborra |
Biophysical Society Annual Meeting 2019
Few of the preprints that were discussed in the recent BPS annual meeting at Baltimore, USA
List by | Joseph Jose Thottacherry |
ASCB/EMBO Annual Meeting 2018
This list relates to preprints that were discussed at the recent ASCB conference.
List by | Dey Lab, Amanda Haage |