Automatic whole cell organelle segmentation in volumetric electron microscopy
Posted on: 22 December 2020
Preprint posted on 16 November 2020
A huge leap for cell biology through automatic organelle segmentation in volume EM.
Selected by Mariana De NizCategories: cell biology
Background
Cells contain hundreds of distinct membrane-bound organelles and macromolecular assemblies that drive the cell’s activities. Although there is considerable knowledge regarding the biochemical and genetic information of these structures, there is little knowledge on the 3D distributions, morphologies, and interactions throughout a cell, at nanometric resolution. Until recently, the main hindrance to obtaining such ultrastructural detail were technological limitations. Modern focused ion beam scanning electron microscopy (FIB-SEM), however, is one of the tools that has addressed these limitations, and provides near-isotropic resolution of cellular structures with nanometric voxel sizes through an entire cell. In parallel, innovations allowing the automatic analysis of the very large volume EM data have provided a means for accessing this detailed information. In their work, Heinrich et al (1) developed an analysis pipeline based on deep learning architectures for segmentation, allowing comprehensive reconstruction and analysis of organelles within entire cells imaged by FIB-SEM.
Key findings and developments
To obtain ground truth annotation for training machine learning algorithms, the authors selected regions of interest for manual annotation of up to 35 different organelles and macromolecular structures, based on morphological features previously described in the literature. They distinguished structures such as mitochondria, microtubules, endoplasmic reticulum, nuclear envelope, and various components of the endolysosomal system, among others, and noted that each structure can be identified with different levels of complexity. Moreover, they mention that manual annotation of the entire cell would take a person 60 years, thus highlighting the need for automation. Noting that the performance of deep learning-based methods depends on representative training data, the authors wanted to generate a method for automatic segmentation that could generalize across cell types and variations in imaging parameters. Training blocks were used to train large, multi-channel 3D-U-Net architectures to predict boundary distances using binary labels for each organelle class. Using the trained networks, they predicted organelle segmentation on whole cells. The authors then developed a manual evaluation method based on pairwise comparisons of whole-cell predictions to determine the optimal training iteration and network architecture for unseen datasets, without a costly generation of validation blocks. They further assessed the performance of the machine learning method, and validated the manual approach in additional holdout blocks. Overall, the results showed good performance scores for organelles well represented in the training data, and setups including more organelles tended to perform slightly better. The authors report that for some features, further refinements were applied to improve segmentation quality, including smoothing, size filtering, watershed segmentation and agglomeration, and masking. They report that the reconstruction of some organelles yielded information previously unknown, for instance, the complexity of the Golgi apparatus.
Investigating microtubules. In the four cells (two HeLa cells, a Jurkat cell and a macrophage cell), the authors show the different quantitative information that can be obtained using the automated pipeline, including count, volume, and surface area of each organelle. Moreover, the possibility to segment out membrane-bound organelles as well as single microtubules allowed investigating the question of how many different organelles are contacting a single microtubule at any time. The authors note these are unprecedented findings that open up new questions related to how organelle distributions and motions are coordinated along a single microtubule.
Investigating the ER. As a further example, the authors quantified ER morphology, including its relationship to other organelles, and partitioned the ER into planar and tubular regions based on its curvature. The authors went on to compare the planar and tubular regions between HeLa cells and macrophages, and showed that a larger fraction of the planar ER regions are supported by mitochondria in the HeLa cells. Given the differences between planar and tubular ER, the authors examined also the relative abundance of ribosomes associated with these ER domains, and using contact site distance, categorized ribosomes as either ER-bound or cytosolic. It appeared that the percentage of ribosomes bound to tubular peripheral ER and the nuclear envelope was consistent across the four cells. Conversely, the relative percentage of ribosomes bound to planar peripheral ER was 3-fold higher in the macrophage than in the other cells.
4nm resolution vs. 8nm resolution. As many FIB-SEM data are acquired at 8nm resolution to reduce imaging time, the authors trained 8nm versions of some of the setups initially acquired at 4nm, by adding unsampling blocks to the 3D-U-Net architecture and randomly downsampling the raw data for training. They found the segmentation and results, satisfactory.
CLEM. Fluorescent light microscopy, in combination with highly specific molecular markers, provides complementary information to high resolution non-specific EM. Using the predictions for mitochondrial membranes, the authors automated the registration process for CLEM datasets, and demonstrated its use in a previously published COS-7 cell transiently expressing ER luminal and mitochondria membrane markers, imaged by PALM and SIM, and FIB-SEM. Comparing manual and automatic registration, the authors report that automatic registration differed from the human annotator by 0.11 µm, while human evaluators differed from each other by 0.03 µm. They conclude that the automated registration agrees with human evaluators in areas with clear mitochondrial signals. Comparing independent registrations of EM to both PALM and SIM showed that errors are small, especially near mitochondria, suggesting that registration is consistent across modalities. This led to the conclusion that automatic organelle segmentation can be used to successfully register CLEM images, making it accessible to less experienced users, and reducing the analysis time significantly.
The authors conclude that to be applicable across domains and new questions, automatic reconstruction methods must generalize robustly across cell types, tissues, and preparation methods. In their work, the authors present a method to fully automatically reconstruct a large number of cellular organelles from FIB-SEM volumes of diverse cell types. Large deep learning architectures were trained to simultaneously reconstruct a large number of cellular organelles and sub-organelle structures at different input resolutions. Moreover, the authors developed the repository “OpenOrganelle” openorganelle.janelia.org, where the raw datasets, training data, reconstructions, open source code, and models are available to the public. Finally, the authors encourage users to explore the datasets and further contribute to scientific discovery.
What I like about this preprint
I like that the authors developed an invaluable tool for cell biology. Often, while hardware based tools take important accelerated steps forward, for instance allowing imaging at resolution never before achieved, the software and analysis pipelines to handle such data often is a few steps back. I think it’s great the authors developed a tool to analyse these rich datasets, that they tested them in various cells, that they identified possible future needs, and that they made all of it publicly available with the hope that open science contributes to the advancement of scientific discovery.
Open questions
- This is a fantastic step for cell biology. You mention that producing training data that is robust enough to allow generalizations across cell types is important. We know that there are important differences across cell types – even more so in cell-cell interactions and upon comparing cells in health and disease. What parameters do you think are important to identify for this baseline training set to apply to as many cell types as possible?
- You mention that your training data contains no tissue data. This adds another step of complexity whereby cell lines differ from primary cells in their native tissues. Do you know how comparable are the parameters in cell lines as opposed to the primary cells they are models for?
- How does fixation and sample preparation influence possible changes in different organelles in different cells, and how do you control for this so as to avoid introducing ultrastructural changes in this process (and confusing it with the cell’s natural ultrastructure)?
- Many pathological conditions affect cell ultrastructure. In your conclusions, you invite the scientific community to explore the datasets here produced as well as the tools you have developed. How do you envisage in the future, that both baseline datasets, and automated FIB-SEM image analysis can be integrated to understand cellular changes in health and disease? For instance, integrating information already known from other types of microscopy to further understand organelle and molecular changes?
- You analysed 35 different organelles and macro-molecular structures. Can you expand further on important findings, unexpected findings/limitations, challenges or paradigm-shifting observations you were able to identify in your work?
References
- Heinrich et al, Automatic whole cell organelle segmentation in volumetric electron microscopy, bioRxiv, 2020.
doi: https://doi.org/10.1242/prelights.26599
Read preprintSign up to customise the site to your preferences and to receive alerts
Register hereAlso in the cell biology category:
Fis1 is required for the development of the dendritic mitochondrial network in pyramidal cortical neurons
Felipe Del Valle Batalla

Mechanical stresses govern myoblast fusion and myotube growth
Vibha SINGH, Prasanna Padmanaban

Polystyrene nanoplastics promote neurodegeneration by catalyzing TDP43 hyperphosphorylation
Manuel Lessi
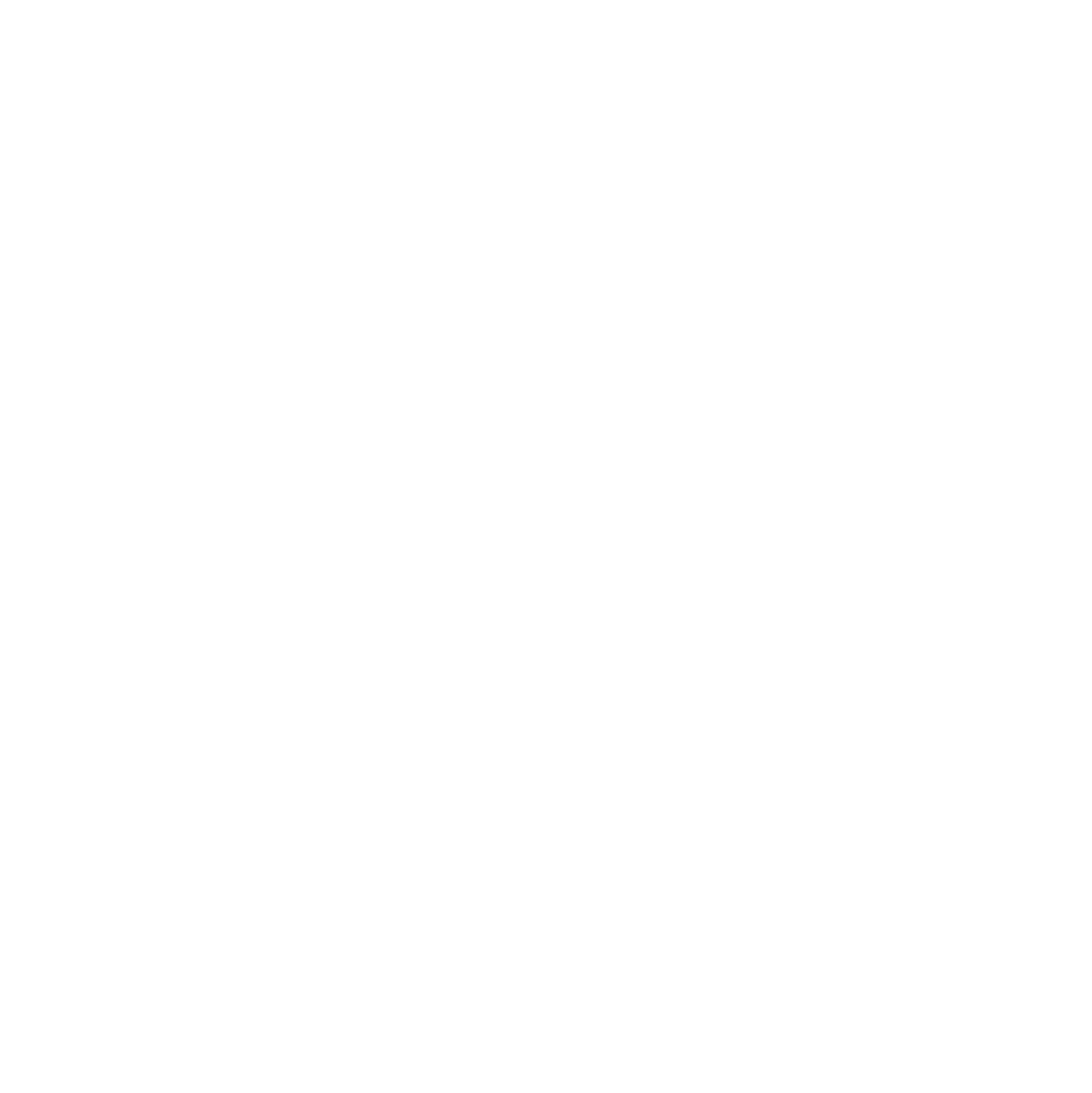
preListscell biology category:
in theCommunity-driven preList – Immunology
In this community-driven preList, a group of preLighters, with expertise in different areas of immunology have worked together to create this preprint reading list.
List by | Felipe Del Valle Batalla et al. |
January in preprints – the CellBio edition
A group of preLighters, with expertise in different areas of cell biology, have worked together to create this preprint reading lists for researchers with an interest in cell biology. This month, categories include: 1) biochemistry/metabolism 2) cell migration 3) cell organelles and organisation 4) cell signalling and mechanosensing 5) genetics/gene expression
List by | Barbora Knotkova et al. |
December in preprints – the CellBio edition
A group of preLighters, with expertise in different areas of cell biology, have worked together to create this preprint reading lists for researchers with an interest in cell biology. This month, categories include: 1) cell cycle and division 2) cell migration and cytoskeleton 3) cell organelles and organisation 4) cell signalling and mechanosensing 5) genetics/gene expression
List by | Matthew Davies et al. |
November in preprints – the CellBio edition
This is the first community-driven preList! A group of preLighters, with expertise in different areas of cell biology, have worked together to create this preprint reading lists for researchers with an interest in cell biology. Categories include: 1) cancer cell biology 2) cell cycle and division 3) cell migration and cytoskeleton 4) cell organelles and organisation 5) cell signalling and mechanosensing 6) genetics/gene expression
List by | Felipe Del Valle Batalla et al. |
BSCB-Biochemical Society 2024 Cell Migration meeting
This preList features preprints that were discussed and presented during the BSCB-Biochemical Society 2024 Cell Migration meeting in Birmingham, UK in April 2024. Kindly put together by Sara Morais da Silva, Reviews Editor at Journal of Cell Science.
List by | Reinier Prosee |
‘In preprints’ from Development 2022-2023
A list of the preprints featured in Development's 'In preprints' articles between 2022-2023
List by | Alex Eve, Katherine Brown |
preLights peer support – preprints of interest
This is a preprint repository to organise the preprints and preLights covered through the 'preLights peer support' initiative.
List by | preLights peer support |
The Society for Developmental Biology 82nd Annual Meeting
This preList is made up of the preprints discussed during the Society for Developmental Biology 82nd Annual Meeting that took place in Chicago in July 2023.
List by | Joyce Yu, Katherine Brown |
CSHL 87th Symposium: Stem Cells
Preprints mentioned by speakers at the #CSHLsymp23
List by | Alex Eve |
Journal of Cell Science meeting ‘Imaging Cell Dynamics’
This preList highlights the preprints discussed at the JCS meeting 'Imaging Cell Dynamics'. The meeting was held from 14 - 17 May 2023 in Lisbon, Portugal and was organised by Erika Holzbaur, Jennifer Lippincott-Schwartz, Rob Parton and Michael Way.
List by | Helen Zenner |
9th International Symposium on the Biology of Vertebrate Sex Determination
This preList contains preprints discussed during the 9th International Symposium on the Biology of Vertebrate Sex Determination. This conference was held in Kona, Hawaii from April 17th to 21st 2023.
List by | Martin Estermann |
Alumni picks – preLights 5th Birthday
This preList contains preprints that were picked and highlighted by preLights Alumni - an initiative that was set up to mark preLights 5th birthday. More entries will follow throughout February and March 2023.
List by | Sergio Menchero et al. |
CellBio 2022 – An ASCB/EMBO Meeting
This preLists features preprints that were discussed and presented during the CellBio 2022 meeting in Washington, DC in December 2022.
List by | Nadja Hümpfer et al. |
Fibroblasts
The advances in fibroblast biology preList explores the recent discoveries and preprints of the fibroblast world. Get ready to immerse yourself with this list created for fibroblasts aficionados and lovers, and beyond. Here, my goal is to include preprints of fibroblast biology, heterogeneity, fate, extracellular matrix, behavior, topography, single-cell atlases, spatial transcriptomics, and their matrix!
List by | Osvaldo Contreras |
EMBL Synthetic Morphogenesis: From Gene Circuits to Tissue Architecture (2021)
A list of preprints mentioned at the #EESmorphoG virtual meeting in 2021.
List by | Alex Eve |
FENS 2020
A collection of preprints presented during the virtual meeting of the Federation of European Neuroscience Societies (FENS) in 2020
List by | Ana Dorrego-Rivas |
Planar Cell Polarity – PCP
This preList contains preprints about the latest findings on Planar Cell Polarity (PCP) in various model organisms at the molecular, cellular and tissue levels.
List by | Ana Dorrego-Rivas |
BioMalPar XVI: Biology and Pathology of the Malaria Parasite
[under construction] Preprints presented at the (fully virtual) EMBL BioMalPar XVI, 17-18 May 2020 #emblmalaria
List by | Dey Lab, Samantha Seah |
1
Cell Polarity
Recent research from the field of cell polarity is summarized in this list of preprints. It comprises of studies focusing on various forms of cell polarity ranging from epithelial polarity, planar cell polarity to front-to-rear polarity.
List by | Yamini Ravichandran |
TAGC 2020
Preprints recently presented at the virtual Allied Genetics Conference, April 22-26, 2020. #TAGC20
List by | Maiko Kitaoka et al. |
3D Gastruloids
A curated list of preprints related to Gastruloids (in vitro models of early development obtained by 3D aggregation of embryonic cells). Updated until July 2021.
List by | Paul Gerald L. Sanchez and Stefano Vianello |
ECFG15 – Fungal biology
Preprints presented at 15th European Conference on Fungal Genetics 17-20 February 2020 Rome
List by | Hiral Shah |
ASCB EMBO Annual Meeting 2019
A collection of preprints presented at the 2019 ASCB EMBO Meeting in Washington, DC (December 7-11)
List by | Madhuja Samaddar et al. |
EMBL Seeing is Believing – Imaging the Molecular Processes of Life
Preprints discussed at the 2019 edition of Seeing is Believing, at EMBL Heidelberg from the 9th-12th October 2019
List by | Dey Lab |
Autophagy
Preprints on autophagy and lysosomal degradation and its role in neurodegeneration and disease. Includes molecular mechanisms, upstream signalling and regulation as well as studies on pharmaceutical interventions to upregulate the process.
List by | Sandra Malmgren Hill |
Lung Disease and Regeneration
This preprint list compiles highlights from the field of lung biology.
List by | Rob Hynds |
Cellular metabolism
A curated list of preprints related to cellular metabolism at Biorxiv by Pablo Ranea Robles from the Prelights community. Special interest on lipid metabolism, peroxisomes and mitochondria.
List by | Pablo Ranea Robles |
BSCB/BSDB Annual Meeting 2019
Preprints presented at the BSCB/BSDB Annual Meeting 2019
List by | Dey Lab |
MitoList
This list of preprints is focused on work expanding our knowledge on mitochondria in any organism, tissue or cell type, from the normal biology to the pathology.
List by | Sandra Franco Iborra |
Biophysical Society Annual Meeting 2019
Few of the preprints that were discussed in the recent BPS annual meeting at Baltimore, USA
List by | Joseph Jose Thottacherry |
ASCB/EMBO Annual Meeting 2018
This list relates to preprints that were discussed at the recent ASCB conference.
List by | Dey Lab, Amanda Haage |