Fully Unsupervised Probabilistic Noise2Void
Posted on: 11 January 2020
Preprint posted on 11 January 2020
Advancing image denoising: Improvements to Probabilistic Noise2Void (PN2V), a method to train convolutional neural networks for image denoising.
Selected by Mariana De NizCategories: bioinformatics, cell biology, microbiology, pathology, physiology, systems biology
Background
Image denoising is the first step in many biomedical image analysis pipelines, and it boils down to separating an image into its two components: the signal s, and the signal-degrading noise n. For this purpose, discriminative deep learning based methods are currently best performing. Previously, methods achieved denoising by training on pairs of noisy images, and corresponding clean target images. Later, a method was proposed which is capable of using independent pairs of noisy images: Noise2Noise (N2N). This approach has the advantage that it removes the need for strenuous collection of clean data. An improvement towards making this step more efficient, came in early 2019 with a method called Noise2Void (N2V) (1), which is a self-supervised training scheme, namely, it does not require noisy image pairs, nor clean target images. It allows training directly on the body of data to be denoised. This method was applied to various types of biomedical images, including fluorescence microscopy, and cryo-EM. A couple of limitations identified in N2V were a) the assumed predictability of signal s – (i.e. if a pixel’s signal is difficult to predict from its surroundings, more errors will appear in N2V predictions); and b) that N2V could not distinguish between the signal and structured noise that violates the assumption that for any given signal the noise is pixel-wise independent (1). Furthermore, the fact that self-supervised methods such as N2V are not competitive with models trained on image pairs raised the question how they could further be improved.
This is because self-supervised training assumes that the noise is pixel-wise independent given the ground-truth signal, and that the true intensity of the pixel can be predicted from local image context, excluding blind spots (single missing pixels) (1). To address this, Laine et al (2) proposed using a Gaussian noise model and predicting Gaussian intensity distributions per pixel. With a method called Probabilistic Noise 2 Void (PN2V), Laine et al (2) and Krug et al (3) proposed a way to leverage information to the network’s blind spots. PN2V puts a probabilistic model for each pixel in place, from which it is possible to infer better denoising results after choose a statistical estimator,i.e. MMSE. The required PN2V noise models are generated from a sequence of noisy calibration images, and characterize the distribution of noisy pixels around their respective ground truth signal value. The current preprint by Prakash et al. goes yet another step further, making PN2V fully unsupervised, hence not requiring to image calibration data any more (4). (Figure 1).
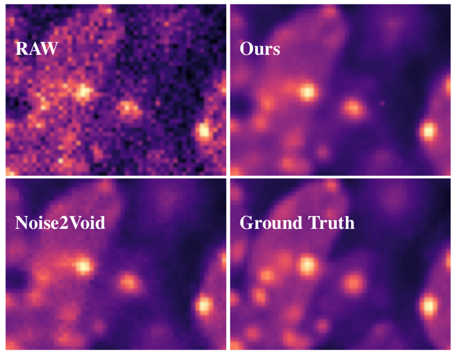
Key findings and developments
General summary
The current work is an improvement over Noise2Void, Noise2Self, and Probabilistic Noise2Void. The previously published Probabilistic Noise2Void (PN2V) required additional noise models for which calibration data is required. The improvement to PN2V presented here replaces histogram based noise models by parametric noise models (using Gaussian Mixture Models (GMM)), and shows how suitable noise models can be created even in the absence of calibration data (i.e. Bootstrapped PN2V), hence rendering PN2V fully unsupervised.
The GMM-based variation of PN2V noise models can lead to higher reconstruction quality, even with imperfect calibration data. The bootstrapping scheme allows PN2V to be trained fully unsupervised, making use of only the data to be denoised. The denoising quality of bootstrapped PN2V was close to fully supervised CARE and outperformed N2V notably.
The authors applied the model to three image sets including a Convalaria dataset, a mouse skull nuclei dataset and a mouse actin dataset, all consisting of calibration and denoised sets. Consistent with open science, the authors make the calibration data and noisy image data publicly available together with the code.
The improvement to PN2V will help to make high quality deep learning-based denoising an easily applicable tool that does not require the acquisition of paired training data or calibration data. This is particularly relevant to experiments where photosensitivity or fast dynamics hinder the acquisition of image pairs.
What I like about this paper
I liked most about this paper the usefulness of the tool presented, and the continuity they give to the work they had previously presented. This is a tool useful to many labs across the biomedical science community. I like the fact that their development is a good example of open science, and of considering the needs of the scientific community- as the authors discuss the process since this tools’ predecessors. I also like that the full datasets are made available, and that the full basis of the improvements to PN2V are explained in detail.
Open questions
1. In your preprint you touch on the question why self-supervised methods are not competitive with models trained on image pairs. Can you expand further on your explanation?
2. You tested the improvements you made on images acquired from spinning disc and point scanning confocal microscopy with great success. Do you expect equal success on images obtained by MRI or EM (as you showed in your previous paper, where you first introduced PN2V)?
3. What are general limitations you would expect using different types of samples (eg. live vs. fixed; strong markers vs. weak), or different imaging methods (eg. MRI, EM, cryo-EM, SR, etc)?
4. Are there specific cases where your improved PN2V model is still unsuccessful and image pairs are still necessary?
5. Could you expand further on the way Gaussian Mixture Models work, for non-specialists?
References
- Krull A, Vicar T, Jug F, Probabilistic Noise2Void: Unsupervised content-aware denoising (2019), arXiv:1906.00651v2
- Laine S, Karras T, Lehtinen J, Aila T, High-quality self-supervised deep image denoising (2019), arXiv:1901.10277
- Krull A, Buchholz TO, Jug F, Noise2Void – Learning denoising from single noisy images (2018), arXiv:1811.10980v2
- Prakash M, Lalit M, Tomancak P, Krull A, Jug F, Fully unsupervised probabilistic Noise2Void (2019), arXiv:1911.12291v1
- Weigert M, Schmidt U, Boothe T, Müller A, Dibrov A, Jain A, Wilhelm B, Schmidt D, Broaddus C, Culley S, Rocha-Martins M, Segovia-Miranda F, Norden C, Henriques R, Zerial M, Solimena M, Rink J, Tomancak P, Royer L, Jug F, Myers EW, Content-aware image restoration: pushing the limits of fluorescence microscopy, (2018), Nature Methods, 15(12):1090-1097, doi: 10.1038/s41592-018-0216-7.
Acknowledgement
I thank Mate Palfy for his feedback on this preLight highlight, and to Florian Jug, Alexander Krull, Mangal Prakash, and Manan Lalit for their feedback and kindly answering questions related to their preprint.
doi: https://doi.org/10.1242/prelights.16203
Read preprintSign up to customise the site to your preferences and to receive alerts
Register hereAlso in the bioinformatics category:
Decoding the Molecular Language of Proteins with Evolla
Jawdat Sandakly

Tidyplots empowers life scientists with easy code-based data visualization
Felipe Del Valle Batalla

IMMClock reveals immune aging and T cell function at single-cell resolution
Jessica Chevallier

Also in the cell biology category:
Deletion of PIEZO1 in adult cardiomyocytes accelerates cardiac aging and causes premature death
Theodora Stougiannou

Megakaryocytes assemble a three-dimensional cage of extracellular matrix that controls their maturation and anchoring to the vascular niche
Simon Cleary

Fis1 is required for the development of the dendritic mitochondrial network in pyramidal cortical neurons
Felipe Del Valle Batalla

Also in the microbiology category:
Green synthesized silver nanoparticles from Moringa: Potential for preventative treatment of SARS-CoV-2 contaminated water
Safieh Shah, Benjamin Dominik Maier
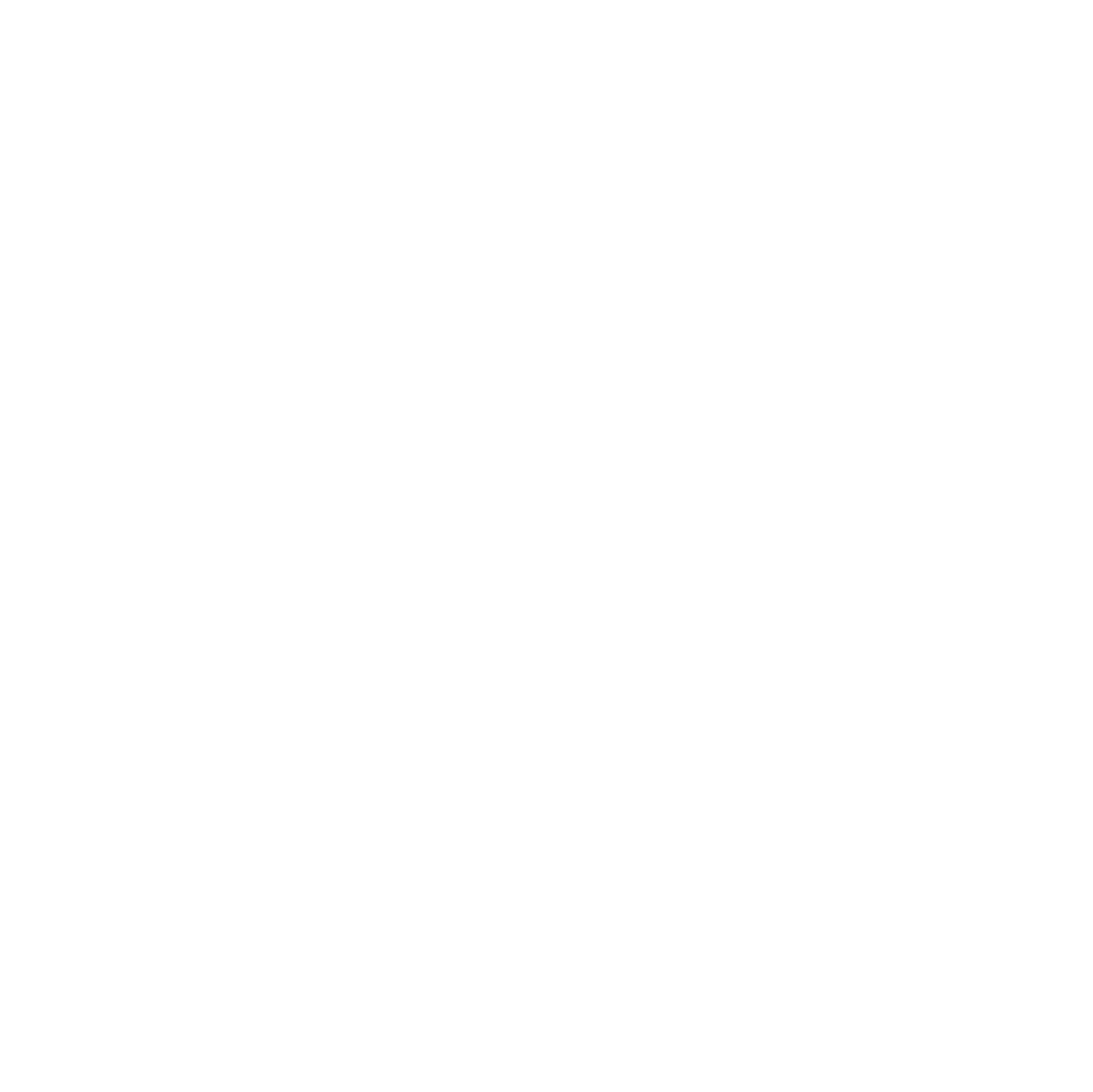
Intracellular diffusion in the cytoplasm increases with cell size in fission yeast
Leeba Ann Chacko, Sameer Thukral

Significantly reduced, but balanced, rates of mitochondrial fission and fusion are sufficient to maintain the integrity of yeast mitochondrial DNA
Leeba Ann Chacko

Also in the pathology category:
Integrin conformation-dependent neutrophil slowing obstructs the capillaries of the pre-metastatic lung in a model of breast cancer
Simon Cleary

LINC complex alterations are a hallmark of sporadic and familial ALS/FTD
Megane Rayer et al.

Hypoxia blunts angiogenic signaling and upregulates the antioxidant system in elephant seal endothelial cells
Sarah Young-Veenstra

Also in the physiology category:
Hyaluronic Acid and Emergent Tissue Mechanics Orchestrate Digit Tip Regeneration
Jonathan Townson

Scaly-Tail Organ Enhances Static Stability during Pel’s Scaly-tailed Flying Squirrels’ Arboreal Locomotion
EMB EMB_Liv et al.

Megakaryocytes assemble a three-dimensional cage of extracellular matrix that controls their maturation and anchoring to the vascular niche
Simon Cleary

Also in the systems biology category:
Modular control of time and space during vertebrate axis segmentation
AND
Natural genetic variation quantitatively regulates heart rate and dimension
Girish Kale, Jennifer Ann Black

Expressive modeling and fast simulation for dynamic compartments
Benjamin Dominik Maier

Clusters of lineage-specific genes are anchored by ZNF274 in repressive perinucleolar compartments
Silvia Carvalho

preLists in the bioinformatics category:
‘In preprints’ from Development 2022-2023
A list of the preprints featured in Development's 'In preprints' articles between 2022-2023
List by | Alex Eve, Katherine Brown |
9th International Symposium on the Biology of Vertebrate Sex Determination
This preList contains preprints discussed during the 9th International Symposium on the Biology of Vertebrate Sex Determination. This conference was held in Kona, Hawaii from April 17th to 21st 2023.
List by | Martin Estermann |
Alumni picks – preLights 5th Birthday
This preList contains preprints that were picked and highlighted by preLights Alumni - an initiative that was set up to mark preLights 5th birthday. More entries will follow throughout February and March 2023.
List by | Sergio Menchero et al. |
Fibroblasts
The advances in fibroblast biology preList explores the recent discoveries and preprints of the fibroblast world. Get ready to immerse yourself with this list created for fibroblasts aficionados and lovers, and beyond. Here, my goal is to include preprints of fibroblast biology, heterogeneity, fate, extracellular matrix, behavior, topography, single-cell atlases, spatial transcriptomics, and their matrix!
List by | Osvaldo Contreras |
Single Cell Biology 2020
A list of preprints mentioned at the Wellcome Genome Campus Single Cell Biology 2020 meeting.
List by | Alex Eve |
Antimicrobials: Discovery, clinical use, and development of resistance
Preprints that describe the discovery of new antimicrobials and any improvements made regarding their clinical use. Includes preprints that detail the factors affecting antimicrobial selection and the development of antimicrobial resistance.
List by | Zhang-He Goh |
Also in the cell biology category:
Biologists @ 100 conference preList
This preList aims to capture all preprints being discussed at the Biologists @100 conference in Liverpool, UK, either as part of the poster sessions or the (flash/short/full-length) talks.
List by | Jonathan Townson, Jonathan Townson |
February in preprints – the CellBio edition
A group of preLighters, with expertise in different areas of cell biology, have worked together to create this preprint reading lists for researchers with an interest in cell biology. This month, categories include: 1) biochemistry and cell metabolism 2) cell organelles and organisation 3) cell signalling, migration and mechanosensing
List by | Barbora Knotkova et al. |
Community-driven preList – Immunology
In this community-driven preList, a group of preLighters, with expertise in different areas of immunology have worked together to create this preprint reading list.
List by | Felipe Del Valle Batalla et al. |
January in preprints – the CellBio edition
A group of preLighters, with expertise in different areas of cell biology, have worked together to create this preprint reading lists for researchers with an interest in cell biology. This month, categories include: 1) biochemistry/metabolism 2) cell migration 3) cell organelles and organisation 4) cell signalling and mechanosensing 5) genetics/gene expression
List by | Barbora Knotkova et al. |
December in preprints – the CellBio edition
A group of preLighters, with expertise in different areas of cell biology, have worked together to create this preprint reading lists for researchers with an interest in cell biology. This month, categories include: 1) cell cycle and division 2) cell migration and cytoskeleton 3) cell organelles and organisation 4) cell signalling and mechanosensing 5) genetics/gene expression
List by | Matthew Davies et al. |
November in preprints – the CellBio edition
This is the first community-driven preList! A group of preLighters, with expertise in different areas of cell biology, have worked together to create this preprint reading lists for researchers with an interest in cell biology. Categories include: 1) cancer cell biology 2) cell cycle and division 3) cell migration and cytoskeleton 4) cell organelles and organisation 5) cell signalling and mechanosensing 6) genetics/gene expression
List by | Felipe Del Valle Batalla et al. |
BSCB-Biochemical Society 2024 Cell Migration meeting
This preList features preprints that were discussed and presented during the BSCB-Biochemical Society 2024 Cell Migration meeting in Birmingham, UK in April 2024. Kindly put together by Sara Morais da Silva, Reviews Editor at Journal of Cell Science.
List by | Reinier Prosee |
‘In preprints’ from Development 2022-2023
A list of the preprints featured in Development's 'In preprints' articles between 2022-2023
List by | Alex Eve, Katherine Brown |
preLights peer support – preprints of interest
This is a preprint repository to organise the preprints and preLights covered through the 'preLights peer support' initiative.
List by | preLights peer support |
The Society for Developmental Biology 82nd Annual Meeting
This preList is made up of the preprints discussed during the Society for Developmental Biology 82nd Annual Meeting that took place in Chicago in July 2023.
List by | Joyce Yu, Katherine Brown |
CSHL 87th Symposium: Stem Cells
Preprints mentioned by speakers at the #CSHLsymp23
List by | Alex Eve |
Journal of Cell Science meeting ‘Imaging Cell Dynamics’
This preList highlights the preprints discussed at the JCS meeting 'Imaging Cell Dynamics'. The meeting was held from 14 - 17 May 2023 in Lisbon, Portugal and was organised by Erika Holzbaur, Jennifer Lippincott-Schwartz, Rob Parton and Michael Way.
List by | Helen Zenner |
9th International Symposium on the Biology of Vertebrate Sex Determination
This preList contains preprints discussed during the 9th International Symposium on the Biology of Vertebrate Sex Determination. This conference was held in Kona, Hawaii from April 17th to 21st 2023.
List by | Martin Estermann |
Alumni picks – preLights 5th Birthday
This preList contains preprints that were picked and highlighted by preLights Alumni - an initiative that was set up to mark preLights 5th birthday. More entries will follow throughout February and March 2023.
List by | Sergio Menchero et al. |
CellBio 2022 – An ASCB/EMBO Meeting
This preLists features preprints that were discussed and presented during the CellBio 2022 meeting in Washington, DC in December 2022.
List by | Nadja Hümpfer et al. |
Fibroblasts
The advances in fibroblast biology preList explores the recent discoveries and preprints of the fibroblast world. Get ready to immerse yourself with this list created for fibroblasts aficionados and lovers, and beyond. Here, my goal is to include preprints of fibroblast biology, heterogeneity, fate, extracellular matrix, behavior, topography, single-cell atlases, spatial transcriptomics, and their matrix!
List by | Osvaldo Contreras |
EMBL Synthetic Morphogenesis: From Gene Circuits to Tissue Architecture (2021)
A list of preprints mentioned at the #EESmorphoG virtual meeting in 2021.
List by | Alex Eve |
FENS 2020
A collection of preprints presented during the virtual meeting of the Federation of European Neuroscience Societies (FENS) in 2020
List by | Ana Dorrego-Rivas |
Planar Cell Polarity – PCP
This preList contains preprints about the latest findings on Planar Cell Polarity (PCP) in various model organisms at the molecular, cellular and tissue levels.
List by | Ana Dorrego-Rivas |
BioMalPar XVI: Biology and Pathology of the Malaria Parasite
[under construction] Preprints presented at the (fully virtual) EMBL BioMalPar XVI, 17-18 May 2020 #emblmalaria
List by | Dey Lab, Samantha Seah |
1
Cell Polarity
Recent research from the field of cell polarity is summarized in this list of preprints. It comprises of studies focusing on various forms of cell polarity ranging from epithelial polarity, planar cell polarity to front-to-rear polarity.
List by | Yamini Ravichandran |
TAGC 2020
Preprints recently presented at the virtual Allied Genetics Conference, April 22-26, 2020. #TAGC20
List by | Maiko Kitaoka et al. |
3D Gastruloids
A curated list of preprints related to Gastruloids (in vitro models of early development obtained by 3D aggregation of embryonic cells). Updated until July 2021.
List by | Paul Gerald L. Sanchez and Stefano Vianello |
ECFG15 – Fungal biology
Preprints presented at 15th European Conference on Fungal Genetics 17-20 February 2020 Rome
List by | Hiral Shah |
ASCB EMBO Annual Meeting 2019
A collection of preprints presented at the 2019 ASCB EMBO Meeting in Washington, DC (December 7-11)
List by | Madhuja Samaddar et al. |
EMBL Seeing is Believing – Imaging the Molecular Processes of Life
Preprints discussed at the 2019 edition of Seeing is Believing, at EMBL Heidelberg from the 9th-12th October 2019
List by | Dey Lab |
Autophagy
Preprints on autophagy and lysosomal degradation and its role in neurodegeneration and disease. Includes molecular mechanisms, upstream signalling and regulation as well as studies on pharmaceutical interventions to upregulate the process.
List by | Sandra Malmgren Hill |
Lung Disease and Regeneration
This preprint list compiles highlights from the field of lung biology.
List by | Rob Hynds |
Cellular metabolism
A curated list of preprints related to cellular metabolism at Biorxiv by Pablo Ranea Robles from the Prelights community. Special interest on lipid metabolism, peroxisomes and mitochondria.
List by | Pablo Ranea Robles |
BSCB/BSDB Annual Meeting 2019
Preprints presented at the BSCB/BSDB Annual Meeting 2019
List by | Dey Lab |
MitoList
This list of preprints is focused on work expanding our knowledge on mitochondria in any organism, tissue or cell type, from the normal biology to the pathology.
List by | Sandra Franco Iborra |
Biophysical Society Annual Meeting 2019
Few of the preprints that were discussed in the recent BPS annual meeting at Baltimore, USA
List by | Joseph Jose Thottacherry |
ASCB/EMBO Annual Meeting 2018
This list relates to preprints that were discussed at the recent ASCB conference.
List by | Dey Lab, Amanda Haage |
Also in the microbiology category:
BioMalPar XVI: Biology and Pathology of the Malaria Parasite
[under construction] Preprints presented at the (fully virtual) EMBL BioMalPar XVI, 17-18 May 2020 #emblmalaria
List by | Dey Lab, Samantha Seah |
1
ECFG15 – Fungal biology
Preprints presented at 15th European Conference on Fungal Genetics 17-20 February 2020 Rome
List by | Hiral Shah |
EMBL Seeing is Believing – Imaging the Molecular Processes of Life
Preprints discussed at the 2019 edition of Seeing is Believing, at EMBL Heidelberg from the 9th-12th October 2019
List by | Dey Lab |
Antimicrobials: Discovery, clinical use, and development of resistance
Preprints that describe the discovery of new antimicrobials and any improvements made regarding their clinical use. Includes preprints that detail the factors affecting antimicrobial selection and the development of antimicrobial resistance.
List by | Zhang-He Goh |
Also in the pathology category:
Fibroblasts
The advances in fibroblast biology preList explores the recent discoveries and preprints of the fibroblast world. Get ready to immerse yourself with this list created for fibroblasts aficionados and lovers, and beyond. Here, my goal is to include preprints of fibroblast biology, heterogeneity, fate, extracellular matrix, behavior, topography, single-cell atlases, spatial transcriptomics, and their matrix!
List by | Osvaldo Contreras |
ECFG15 – Fungal biology
Preprints presented at 15th European Conference on Fungal Genetics 17-20 February 2020 Rome
List by | Hiral Shah |
COVID-19 / SARS-CoV-2 preprints
List of important preprints dealing with the ongoing coronavirus outbreak. See http://covidpreprints.com for additional resources and timeline, and https://connect.biorxiv.org/relate/content/181 for full list of bioRxiv and medRxiv preprints on this topic
List by | Dey Lab, Zhang-He Goh |
1
Cellular metabolism
A curated list of preprints related to cellular metabolism at Biorxiv by Pablo Ranea Robles from the Prelights community. Special interest on lipid metabolism, peroxisomes and mitochondria.
List by | Pablo Ranea Robles |
Also in the physiology category:
Biologists @ 100 conference preList
This preList aims to capture all preprints being discussed at the Biologists @100 conference in Liverpool, UK, either as part of the poster sessions or the (flash/short/full-length) talks.
List by | Jonathan Townson, Jonathan Townson |
Fibroblasts
The advances in fibroblast biology preList explores the recent discoveries and preprints of the fibroblast world. Get ready to immerse yourself with this list created for fibroblasts aficionados and lovers, and beyond. Here, my goal is to include preprints of fibroblast biology, heterogeneity, fate, extracellular matrix, behavior, topography, single-cell atlases, spatial transcriptomics, and their matrix!
List by | Osvaldo Contreras |
FENS 2020
A collection of preprints presented during the virtual meeting of the Federation of European Neuroscience Societies (FENS) in 2020
List by | Ana Dorrego-Rivas |
TAGC 2020
Preprints recently presented at the virtual Allied Genetics Conference, April 22-26, 2020. #TAGC20
List by | Maiko Kitaoka et al. |
Autophagy
Preprints on autophagy and lysosomal degradation and its role in neurodegeneration and disease. Includes molecular mechanisms, upstream signalling and regulation as well as studies on pharmaceutical interventions to upregulate the process.
List by | Sandra Malmgren Hill |
Also in the systems biology category:
2024 Hypothalamus GRC
This 2024 Hypothalamus GRC (Gordon Research Conference) preList offers an overview of cutting-edge research focused on the hypothalamus, a critical brain region involved in regulating homeostasis, behavior, and neuroendocrine functions. The studies included cover a range of topics, including neural circuits, molecular mechanisms, and the role of the hypothalamus in health and disease. This collection highlights some of the latest advances in understanding hypothalamic function, with potential implications for treating disorders such as obesity, stress, and metabolic diseases.
List by | Nathalie Krauth |
‘In preprints’ from Development 2022-2023
A list of the preprints featured in Development's 'In preprints' articles between 2022-2023
List by | Alex Eve, Katherine Brown |
EMBL Synthetic Morphogenesis: From Gene Circuits to Tissue Architecture (2021)
A list of preprints mentioned at the #EESmorphoG virtual meeting in 2021.
List by | Alex Eve |
Single Cell Biology 2020
A list of preprints mentioned at the Wellcome Genome Campus Single Cell Biology 2020 meeting.
List by | Alex Eve |
ASCB EMBO Annual Meeting 2019
A collection of preprints presented at the 2019 ASCB EMBO Meeting in Washington, DC (December 7-11)
List by | Madhuja Samaddar et al. |
EMBL Seeing is Believing – Imaging the Molecular Processes of Life
Preprints discussed at the 2019 edition of Seeing is Believing, at EMBL Heidelberg from the 9th-12th October 2019
List by | Dey Lab |
Pattern formation during development
The aim of this preList is to integrate results about the mechanisms that govern patterning during development, from genes implicated in the processes to theoritical models of pattern formation in nature.
List by | Alexa Sadier |