Memory sequencing reveals heritable single cell gene expression programs associated with distinct cellular behaviors
Posted on: 11 September 2018
Preprint posted on 27 July 2018
Article now published in Cell at http://dx.doi.org/10.1016/j.cell.2020.07.003
Short-term memory: persistence of transient but heritable fluctuations in mRNA levels confer phenotypic variability to genetically identical cells
Selected by Leighton DaighCategories: cancer biology, cell biology, molecular biology, systems biology
Background
Cellular memory enables mother cells to pass on phenotypic information from mother cells from daughter cells in lifeforms from bacteria to multicellular organisms. Genomic DNA sequences represent the most stable form of cellular memory, allowing high fidelity transmission of memory over many generations. Epigenetic memory (e.g., inheritance of chromatin state) allows more plasticity while retaining some degree of stability. For example, epigenetic inheritance is important in development as cells differentiate and need to maintain memory of germ layer, tissue type, etc.
However, the authors note that more transient forms of cellular memory are less well studied and the subject of debate. While cell-to-cell variations in mRNA transcription have been observed, the importance and physiological relevance of these fluctuations is still unclear. Shaffer et al. therefore set out to determine if fluctuations in mRNA expression could be inherited over several rounds of cell division, and if they do, whether this would have phenotypic consequences.
Key findings
To identify transcripts that may exhibit fluctuations persisting over multiple generations of cell divisions, the authors combined Luria and Delbrück’s fluctuation analysis with bulk-cell mRNA sequencing, a technique they termed “MemorySeq.” An isogenic melanoma cell line was sorted into single-cell clones, and each clone was expanded to ~100,000 cells. Each clonal population was subjected to bulk-cell RNA sequencing. The authors looked for transcripts that exhibited a high degree of variance amongst the clonal populations. The authors describe the rational as follows: if a transcript has rapid fluctuations that don’t persist over generations, then during the expansion of each clone many cells will transition in and out of a “high” expression state (Figure 1). Thus, sequencing counts of a given transcript will be fairly similar across clonal populations. However, if a transcript exhibits slower, more persistent fluctuations, then “high” expression of this slowly fluctuating transcript will be inherited across cell divisions. If a cell happened to be in the “high” state early on following single-cell sorting, then clonal expansion will result in a disproportionally high level of that transcript in the population. A slowly-fluctuating transcript (i.e., one with short-term memory) is therefore one with high variability in transcript level across the different clonal populations. The authors were particularly interested in slowly fluctuating transcripts that might be stable across multiple cell divisions, allowing phenotypic inheritance.
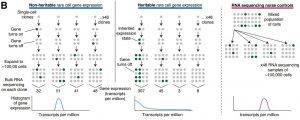
Using this technique, the authors identify 227 slowly fluctuating transcripts (e.g., NGFR). The authors validate their findings through several orthogonal methods. Sparse plating followed by a growth period and mRNA FISH revealed colonies of high-expressing cells amongst a plate of otherwise low-expressing cells. The close spatial proximity of these cells suggests they were all derived from a single highly-expressing cell, providing additional evidence for persistence of high expression levels across multiple generations. The authors also performed endogenous tagging of the NGFR locus with a fluorescent protein. Timelapse fluorescence microscopy of the tagged NGFR protein revealed fluctuations in protein level, suggesting that fluctuations in transcript level translate to fluctuations in protein level.
The authors then provided a nice demonstration of phenotypic consequences of slow fluctuations in transcript level. Cells were sorted for those expressing high levels of the NGFR protein. NGFR-high cells or the original mixed population were treated with a MEK inhibitor, a drug used clinically for the treatment of melanoma. In the mixed population, few resistant colonies were observed. However, in the NGFR-high sorted cells, there was a much greater outgrowth of resistant colonies. Thus, slow fluctuations in transcripts like NGFR may be clinically important in the resistance of cancer cells to targeted therapies.
To demonstrate the flexibility of MemorySeq, the authors also use the technique on a triple-negative breast cancer cell line. Once again, they identify transcripts with slow fluctuations and show that these fluctuations may underlie chemotherapy resistance. This is a nice validation of the technique and suggests that it may be suitable for more widespread use in identifying potential resistance genes in a variety of cancer types and treatment modalities.
Why I picked this preprint
I particularly like this preprint for its readability. The language is clear and concise, with nice explanations of the concepts and interpretations of the data. Diagrams in the first panel of almost every figure clearly depict the experimental setup and hypotheses for potential outcomes of the experiment. Figures are simple to understand and can stand on their own. Overall, it makes for a fun reading experience where you get to follow along with an interesting scientific story.
A better understanding of more flexible forms of cellular memory will contribute to our understanding of how cancer cells may avoid therapy, as the authors demonstrate. Transient memory may also prove important in aspects of normal cellular functioning as well. For example, transient fluctuations in mRNA expression levels may be important during development or in response to environmental stressors such as viral infection. Therefore, I expect future studies to build on this interesting concept and extend it to other areas of cell and organismal biology.
I also enjoy the use of classical techniques combined with new methods to answer interesting questions in biology. In this paper (and in a past paper), the authors employed fluctuation analysis as the fundamental technique of the paper (Shaffer et al., 2017). This technique was most famously used in Luria and Delbrück’s seminal paper studying the heritable nature of bacterial resistance to bacteria-destroying viruses (Luria and Delbrück, 1943). The combination of modern and classical techniques makes for a fun paper that inspired a sense of nostalgia for me.
To those interested in reading more about classical biology, I would recommend The Eighth of Creation by Horace Judson and Phage and the Origins of Molecular Biology from Cold Spring Harbor Lab Press. Both books provide insight into the context behind many of the famous biology experiments of the 20th century.
Additional Questions
- I think the most intriguing question from this work is: from where do the slow fluctuations arise? There are many steps in the generation of an RNA transcript, and I could imagine that any one (or more) of them could give rise to fluctuations in transcript level.
- To what degree do fluctuations in transcript level correlate with fluctuations in protein level? Fluctuations in NGFR protein levels are demonstrated, but I’m curious to what degree this is more universally true.
- Are these long-term changes in mRNA levels reversible? At what frequency do cells revert to/from the high-expression state?
Citations and Further reading
- Luria, S. E., Delbrück, M. (1943). Mutations of Bacteria from Virus Sensitivity to Virus Resistance. Genetics 28, 491–511.
- Shaffer, S.M., Dunagin, M.C., Torborg, S.R., Torre, E.A., Emert, B., Krepler, C., Beqiri, M., Sproesser, K., Brafford, P.A., Xiao, M., et al. (2017). Rare cell variability and drug-induced reprogramming as a mode of cancer drug resistance. Nature 546, 431-435.
- Symmons, O., and Raj, A. (2016). What’s Luck Got to Do with It: Single Cells, Multiple Fates, and Biological Nondeterminism. Mol Cell 62, 788-802.
doi: https://doi.org/10.1242/prelights.4711
Read preprintArjun Raj
Thanks so much for preLighting our paper!! And so sorry for the crazy late reply…
Regarding where the slow fluctuations arise: absolutely no idea! We don’t think that it has to do with the details of the transcriptional process per se, but rather something about the regulatory milieu. We (Lea Schuh and Yogesh Goyal) have done some simulations showing that pretty standard models of transcriptional bursting coupled with regulatory interactions CAN in principle give these sorts of fluctuations, but of course, could be some other mechanism as well.
Fluctuations at the protein level: well, we looked at NGFR by live cell tagging, and have also demonstrated correlation by a few other antibodies (CA9, EGFR, AXL). Probably holds more generally, but we are doing some more tagging with timelapse to figure it out.
Regarding reversibility: yes, the changes appear reversible. It can take a while, and we still don’t have a good sense of what the time scale is—probably something on the order of 3-10 cell divisions. Probably also depends on the particular gene, as some will be faster/slower than others.
Anyway, thanks again so much for highlighting our paper!
Arjun
Have your say
Sign up to customise the site to your preferences and to receive alerts
Register hereAlso in the cancer biology category:
Target cell tension regulates macrophage trogocytosis
Felipe Del Valle Batalla

Restoring mechanophenotype reverts malignant properties of ECM-enriched vocal fold cancer
Teodora Piskova

Integrin conformation-dependent neutrophil slowing obstructs the capillaries of the pre-metastatic lung in a model of breast cancer
Simon Cleary

Also in the cell biology category:
Deletion of PIEZO1 in adult cardiomyocytes accelerates cardiac aging and causes premature death
Theodora Stougiannou

Megakaryocytes assemble a three-dimensional cage of extracellular matrix that controls their maturation and anchoring to the vascular niche
Simon Cleary

Fis1 is required for the development of the dendritic mitochondrial network in pyramidal cortical neurons
Felipe Del Valle Batalla

Also in the molecular biology category:
Conservation and divergence of regulatory architecture in nitrate-responsive plant gene circuits
Jeny Jose
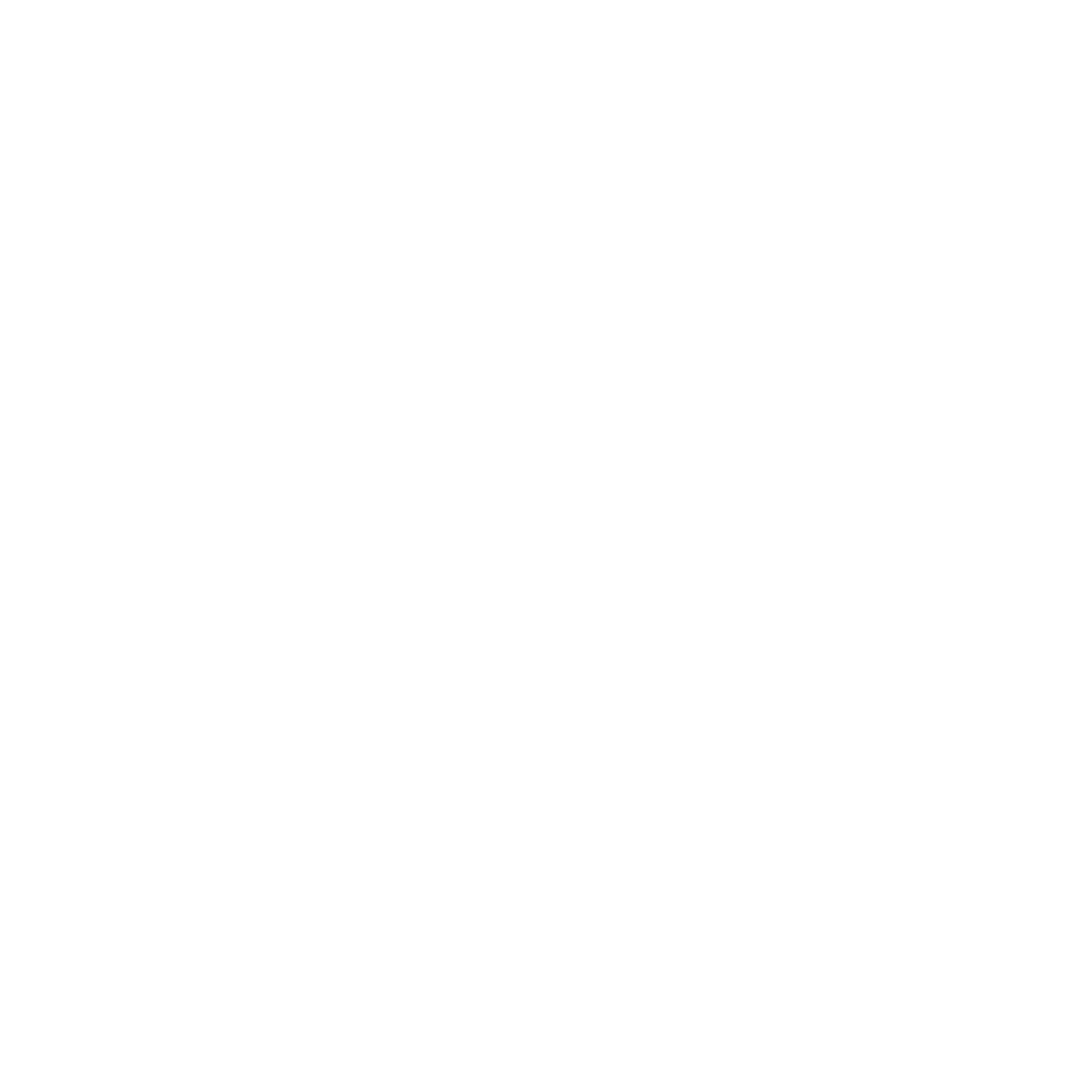
Levetiracetam prevents Aβ42 production through SV2a-dependent modulation of App processing in Alzheimer’s disease models
Jawdat Sandakly
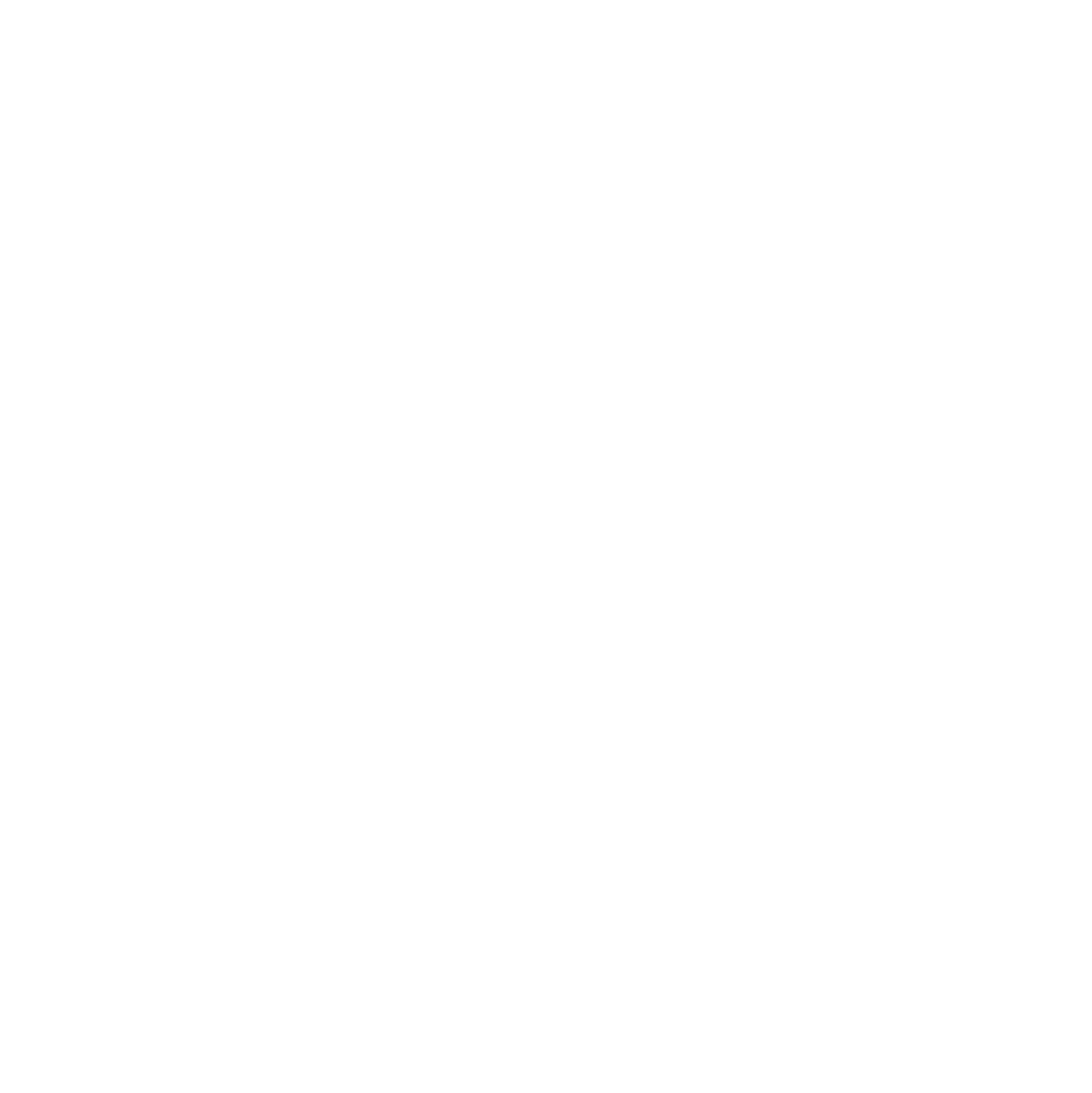
Chromosomal instability in human trophoblast stem cells and placentas
Carly Guiltinan

Also in the systems biology category:
Modular control of time and space during vertebrate axis segmentation
AND
Natural genetic variation quantitatively regulates heart rate and dimension
Girish Kale, Jennifer Ann Black

Expressive modeling and fast simulation for dynamic compartments
Benjamin Dominik Maier

Clusters of lineage-specific genes are anchored by ZNF274 in repressive perinucleolar compartments
Silvia Carvalho

preLists in the cancer biology category:
Biologists @ 100 conference preList
This preList aims to capture all preprints being discussed at the Biologists @100 conference in Liverpool, UK, either as part of the poster sessions or the (flash/short/full-length) talks.
List by | Jonathan Townson, Jonathan Townson |
February in preprints – the CellBio edition
A group of preLighters, with expertise in different areas of cell biology, have worked together to create this preprint reading lists for researchers with an interest in cell biology. This month, categories include: 1) biochemistry and cell metabolism 2) cell organelles and organisation 3) cell signalling, migration and mechanosensing
List by | Barbora Knotkova et al. |
BSCB-Biochemical Society 2024 Cell Migration meeting
This preList features preprints that were discussed and presented during the BSCB-Biochemical Society 2024 Cell Migration meeting in Birmingham, UK in April 2024. Kindly put together by Sara Morais da Silva, Reviews Editor at Journal of Cell Science.
List by | Reinier Prosee |
CSHL 87th Symposium: Stem Cells
Preprints mentioned by speakers at the #CSHLsymp23
List by | Alex Eve |
Journal of Cell Science meeting ‘Imaging Cell Dynamics’
This preList highlights the preprints discussed at the JCS meeting 'Imaging Cell Dynamics'. The meeting was held from 14 - 17 May 2023 in Lisbon, Portugal and was organised by Erika Holzbaur, Jennifer Lippincott-Schwartz, Rob Parton and Michael Way.
List by | Helen Zenner |
CellBio 2022 – An ASCB/EMBO Meeting
This preLists features preprints that were discussed and presented during the CellBio 2022 meeting in Washington, DC in December 2022.
List by | Nadja Hümpfer et al. |
Fibroblasts
The advances in fibroblast biology preList explores the recent discoveries and preprints of the fibroblast world. Get ready to immerse yourself with this list created for fibroblasts aficionados and lovers, and beyond. Here, my goal is to include preprints of fibroblast biology, heterogeneity, fate, extracellular matrix, behavior, topography, single-cell atlases, spatial transcriptomics, and their matrix!
List by | Osvaldo Contreras |
Single Cell Biology 2020
A list of preprints mentioned at the Wellcome Genome Campus Single Cell Biology 2020 meeting.
List by | Alex Eve |
ASCB EMBO Annual Meeting 2019
A collection of preprints presented at the 2019 ASCB EMBO Meeting in Washington, DC (December 7-11)
List by | Madhuja Samaddar et al. |
Lung Disease and Regeneration
This preprint list compiles highlights from the field of lung biology.
List by | Rob Hynds |
Anticancer agents: Discovery and clinical use
Preprints that describe the discovery of anticancer agents and their clinical use. Includes both small molecules and macromolecules like biologics.
List by | Zhang-He Goh |
Biophysical Society Annual Meeting 2019
Few of the preprints that were discussed in the recent BPS annual meeting at Baltimore, USA
List by | Joseph Jose Thottacherry |
Also in the cell biology category:
Biologists @ 100 conference preList
This preList aims to capture all preprints being discussed at the Biologists @100 conference in Liverpool, UK, either as part of the poster sessions or the (flash/short/full-length) talks.
List by | Jonathan Townson, Jonathan Townson |
February in preprints – the CellBio edition
A group of preLighters, with expertise in different areas of cell biology, have worked together to create this preprint reading lists for researchers with an interest in cell biology. This month, categories include: 1) biochemistry and cell metabolism 2) cell organelles and organisation 3) cell signalling, migration and mechanosensing
List by | Barbora Knotkova et al. |
Community-driven preList – Immunology
In this community-driven preList, a group of preLighters, with expertise in different areas of immunology have worked together to create this preprint reading list.
List by | Felipe Del Valle Batalla et al. |
January in preprints – the CellBio edition
A group of preLighters, with expertise in different areas of cell biology, have worked together to create this preprint reading lists for researchers with an interest in cell biology. This month, categories include: 1) biochemistry/metabolism 2) cell migration 3) cell organelles and organisation 4) cell signalling and mechanosensing 5) genetics/gene expression
List by | Barbora Knotkova et al. |
December in preprints – the CellBio edition
A group of preLighters, with expertise in different areas of cell biology, have worked together to create this preprint reading lists for researchers with an interest in cell biology. This month, categories include: 1) cell cycle and division 2) cell migration and cytoskeleton 3) cell organelles and organisation 4) cell signalling and mechanosensing 5) genetics/gene expression
List by | Matthew Davies et al. |
November in preprints – the CellBio edition
This is the first community-driven preList! A group of preLighters, with expertise in different areas of cell biology, have worked together to create this preprint reading lists for researchers with an interest in cell biology. Categories include: 1) cancer cell biology 2) cell cycle and division 3) cell migration and cytoskeleton 4) cell organelles and organisation 5) cell signalling and mechanosensing 6) genetics/gene expression
List by | Felipe Del Valle Batalla et al. |
BSCB-Biochemical Society 2024 Cell Migration meeting
This preList features preprints that were discussed and presented during the BSCB-Biochemical Society 2024 Cell Migration meeting in Birmingham, UK in April 2024. Kindly put together by Sara Morais da Silva, Reviews Editor at Journal of Cell Science.
List by | Reinier Prosee |
‘In preprints’ from Development 2022-2023
A list of the preprints featured in Development's 'In preprints' articles between 2022-2023
List by | Alex Eve, Katherine Brown |
preLights peer support – preprints of interest
This is a preprint repository to organise the preprints and preLights covered through the 'preLights peer support' initiative.
List by | preLights peer support |
The Society for Developmental Biology 82nd Annual Meeting
This preList is made up of the preprints discussed during the Society for Developmental Biology 82nd Annual Meeting that took place in Chicago in July 2023.
List by | Joyce Yu, Katherine Brown |
CSHL 87th Symposium: Stem Cells
Preprints mentioned by speakers at the #CSHLsymp23
List by | Alex Eve |
Journal of Cell Science meeting ‘Imaging Cell Dynamics’
This preList highlights the preprints discussed at the JCS meeting 'Imaging Cell Dynamics'. The meeting was held from 14 - 17 May 2023 in Lisbon, Portugal and was organised by Erika Holzbaur, Jennifer Lippincott-Schwartz, Rob Parton and Michael Way.
List by | Helen Zenner |
9th International Symposium on the Biology of Vertebrate Sex Determination
This preList contains preprints discussed during the 9th International Symposium on the Biology of Vertebrate Sex Determination. This conference was held in Kona, Hawaii from April 17th to 21st 2023.
List by | Martin Estermann |
Alumni picks – preLights 5th Birthday
This preList contains preprints that were picked and highlighted by preLights Alumni - an initiative that was set up to mark preLights 5th birthday. More entries will follow throughout February and March 2023.
List by | Sergio Menchero et al. |
CellBio 2022 – An ASCB/EMBO Meeting
This preLists features preprints that were discussed and presented during the CellBio 2022 meeting in Washington, DC in December 2022.
List by | Nadja Hümpfer et al. |
Fibroblasts
The advances in fibroblast biology preList explores the recent discoveries and preprints of the fibroblast world. Get ready to immerse yourself with this list created for fibroblasts aficionados and lovers, and beyond. Here, my goal is to include preprints of fibroblast biology, heterogeneity, fate, extracellular matrix, behavior, topography, single-cell atlases, spatial transcriptomics, and their matrix!
List by | Osvaldo Contreras |
EMBL Synthetic Morphogenesis: From Gene Circuits to Tissue Architecture (2021)
A list of preprints mentioned at the #EESmorphoG virtual meeting in 2021.
List by | Alex Eve |
FENS 2020
A collection of preprints presented during the virtual meeting of the Federation of European Neuroscience Societies (FENS) in 2020
List by | Ana Dorrego-Rivas |
Planar Cell Polarity – PCP
This preList contains preprints about the latest findings on Planar Cell Polarity (PCP) in various model organisms at the molecular, cellular and tissue levels.
List by | Ana Dorrego-Rivas |
BioMalPar XVI: Biology and Pathology of the Malaria Parasite
[under construction] Preprints presented at the (fully virtual) EMBL BioMalPar XVI, 17-18 May 2020 #emblmalaria
List by | Dey Lab, Samantha Seah |
1
Cell Polarity
Recent research from the field of cell polarity is summarized in this list of preprints. It comprises of studies focusing on various forms of cell polarity ranging from epithelial polarity, planar cell polarity to front-to-rear polarity.
List by | Yamini Ravichandran |
TAGC 2020
Preprints recently presented at the virtual Allied Genetics Conference, April 22-26, 2020. #TAGC20
List by | Maiko Kitaoka et al. |
3D Gastruloids
A curated list of preprints related to Gastruloids (in vitro models of early development obtained by 3D aggregation of embryonic cells). Updated until July 2021.
List by | Paul Gerald L. Sanchez and Stefano Vianello |
ECFG15 – Fungal biology
Preprints presented at 15th European Conference on Fungal Genetics 17-20 February 2020 Rome
List by | Hiral Shah |
ASCB EMBO Annual Meeting 2019
A collection of preprints presented at the 2019 ASCB EMBO Meeting in Washington, DC (December 7-11)
List by | Madhuja Samaddar et al. |
EMBL Seeing is Believing – Imaging the Molecular Processes of Life
Preprints discussed at the 2019 edition of Seeing is Believing, at EMBL Heidelberg from the 9th-12th October 2019
List by | Dey Lab |
Autophagy
Preprints on autophagy and lysosomal degradation and its role in neurodegeneration and disease. Includes molecular mechanisms, upstream signalling and regulation as well as studies on pharmaceutical interventions to upregulate the process.
List by | Sandra Malmgren Hill |
Lung Disease and Regeneration
This preprint list compiles highlights from the field of lung biology.
List by | Rob Hynds |
Cellular metabolism
A curated list of preprints related to cellular metabolism at Biorxiv by Pablo Ranea Robles from the Prelights community. Special interest on lipid metabolism, peroxisomes and mitochondria.
List by | Pablo Ranea Robles |
BSCB/BSDB Annual Meeting 2019
Preprints presented at the BSCB/BSDB Annual Meeting 2019
List by | Dey Lab |
MitoList
This list of preprints is focused on work expanding our knowledge on mitochondria in any organism, tissue or cell type, from the normal biology to the pathology.
List by | Sandra Franco Iborra |
ASCB/EMBO Annual Meeting 2018
This list relates to preprints that were discussed at the recent ASCB conference.
List by | Dey Lab, Amanda Haage |
Also in the molecular biology category:
Biologists @ 100 conference preList
This preList aims to capture all preprints being discussed at the Biologists @100 conference in Liverpool, UK, either as part of the poster sessions or the (flash/short/full-length) talks.
List by | Jonathan Townson, Jonathan Townson |
February in preprints – the CellBio edition
A group of preLighters, with expertise in different areas of cell biology, have worked together to create this preprint reading lists for researchers with an interest in cell biology. This month, categories include: 1) biochemistry and cell metabolism 2) cell organelles and organisation 3) cell signalling, migration and mechanosensing
List by | Barbora Knotkova et al. |
Community-driven preList – Immunology
In this community-driven preList, a group of preLighters, with expertise in different areas of immunology have worked together to create this preprint reading list.
List by | Felipe Del Valle Batalla et al. |
January in preprints – the CellBio edition
A group of preLighters, with expertise in different areas of cell biology, have worked together to create this preprint reading lists for researchers with an interest in cell biology. This month, categories include: 1) biochemistry/metabolism 2) cell migration 3) cell organelles and organisation 4) cell signalling and mechanosensing 5) genetics/gene expression
List by | Barbora Knotkova et al. |
2024 Hypothalamus GRC
This 2024 Hypothalamus GRC (Gordon Research Conference) preList offers an overview of cutting-edge research focused on the hypothalamus, a critical brain region involved in regulating homeostasis, behavior, and neuroendocrine functions. The studies included cover a range of topics, including neural circuits, molecular mechanisms, and the role of the hypothalamus in health and disease. This collection highlights some of the latest advances in understanding hypothalamic function, with potential implications for treating disorders such as obesity, stress, and metabolic diseases.
List by | Nathalie Krauth |
BSCB-Biochemical Society 2024 Cell Migration meeting
This preList features preprints that were discussed and presented during the BSCB-Biochemical Society 2024 Cell Migration meeting in Birmingham, UK in April 2024. Kindly put together by Sara Morais da Silva, Reviews Editor at Journal of Cell Science.
List by | Reinier Prosee |
‘In preprints’ from Development 2022-2023
A list of the preprints featured in Development's 'In preprints' articles between 2022-2023
List by | Alex Eve, Katherine Brown |
CSHL 87th Symposium: Stem Cells
Preprints mentioned by speakers at the #CSHLsymp23
List by | Alex Eve |
9th International Symposium on the Biology of Vertebrate Sex Determination
This preList contains preprints discussed during the 9th International Symposium on the Biology of Vertebrate Sex Determination. This conference was held in Kona, Hawaii from April 17th to 21st 2023.
List by | Martin Estermann |
Alumni picks – preLights 5th Birthday
This preList contains preprints that were picked and highlighted by preLights Alumni - an initiative that was set up to mark preLights 5th birthday. More entries will follow throughout February and March 2023.
List by | Sergio Menchero et al. |
CellBio 2022 – An ASCB/EMBO Meeting
This preLists features preprints that were discussed and presented during the CellBio 2022 meeting in Washington, DC in December 2022.
List by | Nadja Hümpfer et al. |
EMBL Synthetic Morphogenesis: From Gene Circuits to Tissue Architecture (2021)
A list of preprints mentioned at the #EESmorphoG virtual meeting in 2021.
List by | Alex Eve |
FENS 2020
A collection of preprints presented during the virtual meeting of the Federation of European Neuroscience Societies (FENS) in 2020
List by | Ana Dorrego-Rivas |
ECFG15 – Fungal biology
Preprints presented at 15th European Conference on Fungal Genetics 17-20 February 2020 Rome
List by | Hiral Shah |
ASCB EMBO Annual Meeting 2019
A collection of preprints presented at the 2019 ASCB EMBO Meeting in Washington, DC (December 7-11)
List by | Madhuja Samaddar et al. |
Lung Disease and Regeneration
This preprint list compiles highlights from the field of lung biology.
List by | Rob Hynds |
MitoList
This list of preprints is focused on work expanding our knowledge on mitochondria in any organism, tissue or cell type, from the normal biology to the pathology.
List by | Sandra Franco Iborra |
Also in the systems biology category:
2024 Hypothalamus GRC
This 2024 Hypothalamus GRC (Gordon Research Conference) preList offers an overview of cutting-edge research focused on the hypothalamus, a critical brain region involved in regulating homeostasis, behavior, and neuroendocrine functions. The studies included cover a range of topics, including neural circuits, molecular mechanisms, and the role of the hypothalamus in health and disease. This collection highlights some of the latest advances in understanding hypothalamic function, with potential implications for treating disorders such as obesity, stress, and metabolic diseases.
List by | Nathalie Krauth |
‘In preprints’ from Development 2022-2023
A list of the preprints featured in Development's 'In preprints' articles between 2022-2023
List by | Alex Eve, Katherine Brown |
EMBL Synthetic Morphogenesis: From Gene Circuits to Tissue Architecture (2021)
A list of preprints mentioned at the #EESmorphoG virtual meeting in 2021.
List by | Alex Eve |
Single Cell Biology 2020
A list of preprints mentioned at the Wellcome Genome Campus Single Cell Biology 2020 meeting.
List by | Alex Eve |
ASCB EMBO Annual Meeting 2019
A collection of preprints presented at the 2019 ASCB EMBO Meeting in Washington, DC (December 7-11)
List by | Madhuja Samaddar et al. |
EMBL Seeing is Believing – Imaging the Molecular Processes of Life
Preprints discussed at the 2019 edition of Seeing is Believing, at EMBL Heidelberg from the 9th-12th October 2019
List by | Dey Lab |
Pattern formation during development
The aim of this preList is to integrate results about the mechanisms that govern patterning during development, from genes implicated in the processes to theoritical models of pattern formation in nature.
List by | Alexa Sadier |
7 years
Carmen Adriaens
Hey Leighton, awesome preLight!
This study pertains very interestingly to another concept in RNA/cell identity biology: are each of the cells inherently different from each other (do they have their own identity?) or are they the same and do they display differences in “behavior” (does their mood change upon their context?). Is this behavior, potentially driven by its environment, going to be ingrained in the next generation? This is particularly fascinating, thinking about how each cell descents from another one in the population (which, even in normal development, is true, to an extreme extent).
As we are starting to understand how a single cell in a tissue distinguishes itself from its neighbor, and how it knows what to “do” in its exact location within a certain tissue, I think these concepts and questions are becoming increasingly important. Basic (in-plate) experiments may prove extremely useful to start studying, in a potentially slightly less complex model system (the plate, the monolayer), a concept that we will hopefully decipher in the future!