Applications, Promises, and Pitfalls of Deep Learning for Fluorescence Image Reconstruction
Posted on: 9 January 2019
Preprint posted on 11 December 2018
Belthangady & Royer present a comprehensive and accessible perspective on the current and future impacts of Artificial Intelligence on microscopy. Their vision for the field is inspiring and sets the ground for an AI revolution.
Selected by Romain F. LaineCategories: biochemistry, bioinformatics, biophysics, cancer biology, cell biology, developmental biology, microbiology
Background of the preprint
The development of artificial intelligence (AI) is driving fundamental changes in the way we travel (self-driving cars), we work (automation) and we interact with the world. It is also being exploited to improve diagnosis and detection of cancer. But there is no reason to stop here, fundamental research can also benefit from these advances and this has triggered the start of a revolution in the field of microscopy. The past couple of years have seen a growing literature on application of artificial intelligence to all kind of imaging techniques for biomedical research. Here, Chinmay Belthangady & Loic Royer from the Chan Zuckerberg Biohub, San Francisco, USA review the recent and promising developments in the field. This review aims at clearly presenting the wide range of applications of AI and its pitfalls and gives perspective to Loic Royer’s seminal work on fluorescence microscopy image restoration using AI [1].
Key points of the preprint
The paper first introduces a number of fundamental definitions about AI in the context of fluorescence microscopy. AI can generate an accurate mathematical transform that is able to take a corrupted microscopy image (e.g. with a lot of noise, or a low resolution) and correct for this image corruption. The way it does this is by learning how the data corruption occurs through looking at a lot of examples of data where the same type of corruption is present (the so-called ‘training dataset’). The authors describe how the typical neural networks used to carry this out can be thought of, e.g. a sequence of mathematical functions that progressively extract the important features in the image. The notion that AI acts as a black box is commonly founded on the fact that these features can be very abstract and therefore cannot be easily understood by the researchers.
The recent applications of AI to microscopy have showed that it can significantly improve noise or out-of-focus light removal and even provide super-resolution in unprecedented ways. The authors highlight that one major advantage of this is to be able to significantly reduce illumination on the sample because the images can be corrected post-acquisition, therefore enabling non-invasive long-term live sample imaging.
The authors then present a number of exciting directions where AI could be useful and have a major impact, e.g. massive multi-color imaging, image registration and alignment, intelligent and AI-guided image acquisition, or even providing a higher level of data mining: “In future, we expect that Deep Learning models trained end-to-end will blur the frontier between image reconstruction and image analysis.”
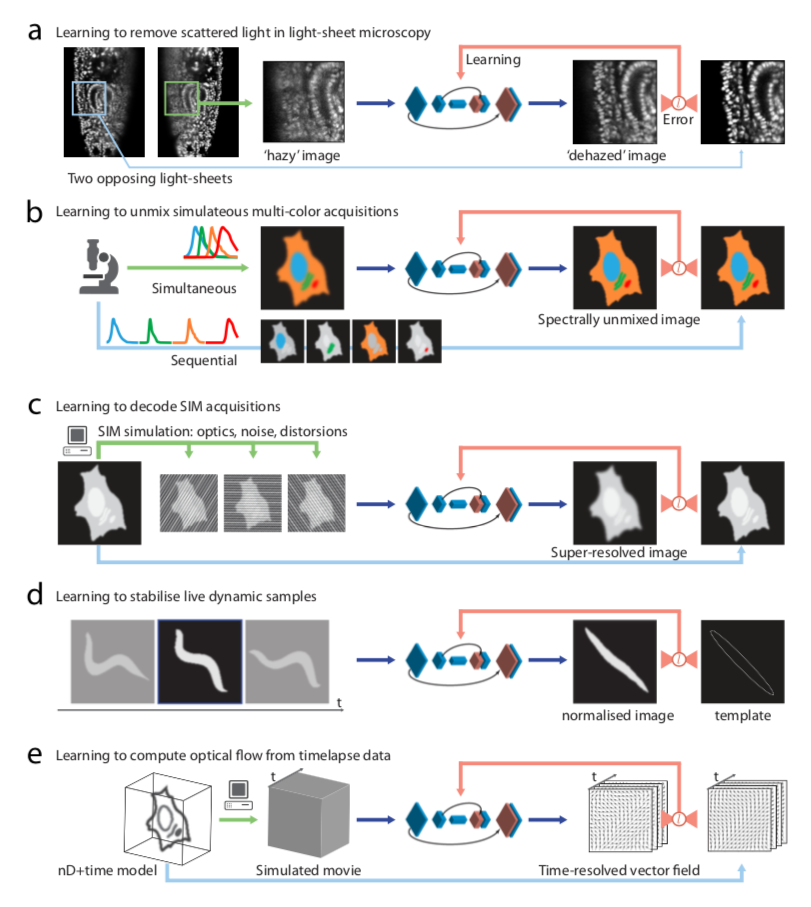
This manuscript also elegantly explains what the limitations of the approach are. When used with the wrong type of training dataset or when too few examples are available for it to learn, it can create hallucinations and therefore make up very realistic features that were not actually present in the underlying sample. The authors make a convincing case about looking beyond the scepticism sometimes found around applying AI to microscopy and focussing on where it is appropriate to use it and its performance.
What I like about this preprint
This preprint provides the accessible overview about AI applied to microscopy, that I have been waiting for, for a couple of years now. It is very well written and presents complex concepts with well-chosen examples. The authors make a balanced case of the pros and cons of AI, but highlight the areas where its potential remains untapped.
Future directions
It is clear to me that AI will change fundamentally the way we acquire and analyse our microscopy data. But in what ways? While this still remains to be established, this preprint sets the ground for an exciting path full of potential. For AI to become mainstream in microscopy, the bottleneck in my opinion still is usability and accessibility of these complex mathematical tools. Will every research department or institute soon need their own in-house AI team? Will these be run like some bioinformatics facilities are today?
doi: https://doi.org/10.1242/prelights.7148
Read preprintSign up to customise the site to your preferences and to receive alerts
Register hereAlso in the biochemistry category:
Notch3 is a genetic modifier of NODAL signalling for patterning asymmetry during mouse heart looping
Bhaval Parmar

14-3-3 binding regulates Tau assembly and microtubule association
Barbora Knotkova et al.

Structural basis of respiratory complexes adaptation to cold temperatures
Pamela Ornelas

Also in the bioinformatics category:
Enhancer-driven cell type comparison reveals similarities between the mammalian and bird pallium
Rodrigo Senovilla-Ganzo

Expressive modeling and fast simulation for dynamic compartments
Benjamin Dominik Maier

Transcriptional profiling of human brain cortex identifies novel lncRNA-mediated networks dysregulated in amyotrophic lateral sclerosis
Julio Molina Pineda
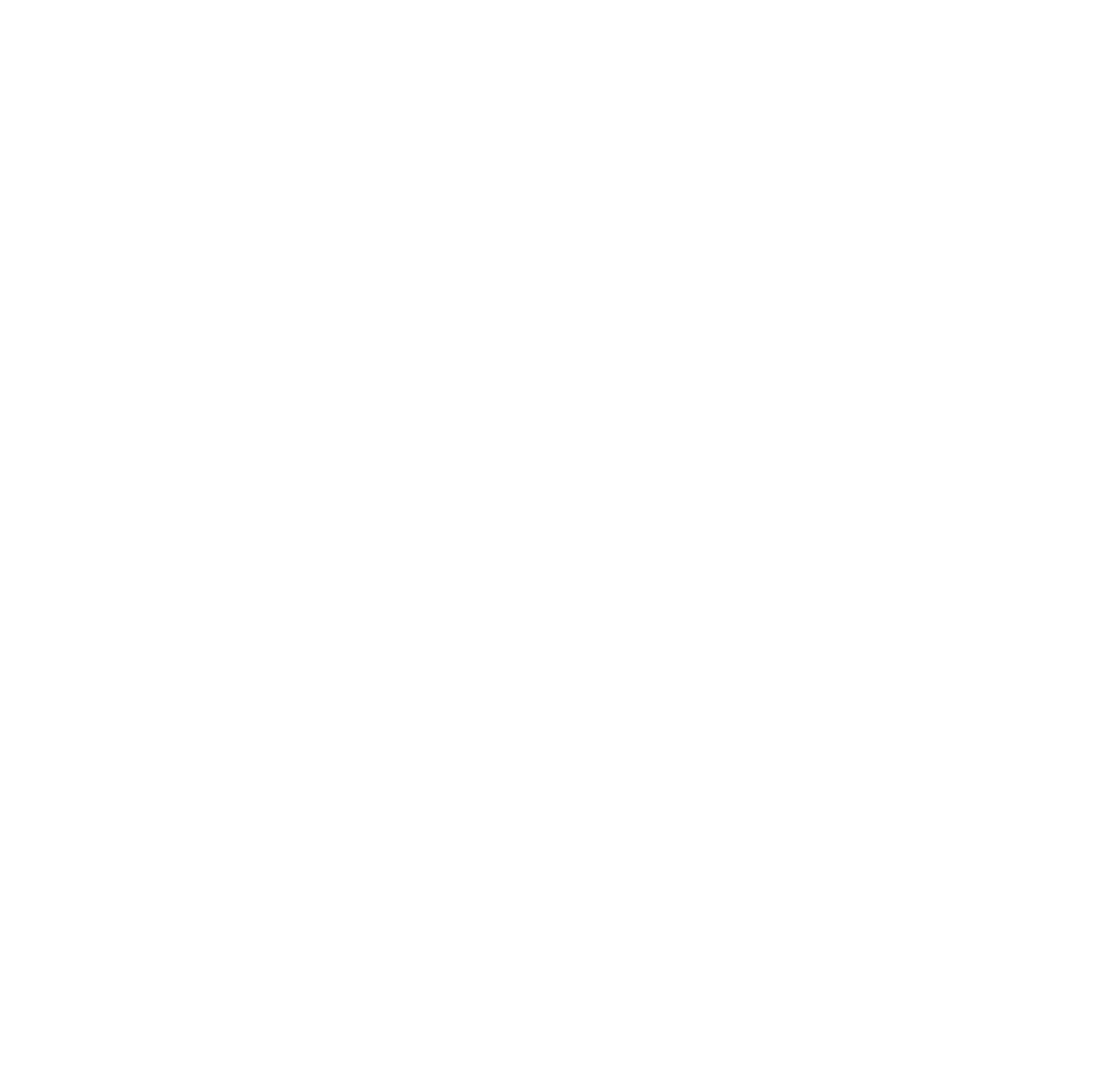
Also in the biophysics category:
Topology changes of the regenerating Hydra define actin nematic defects as mechanical organizers of morphogenesis
Rachel Mckeown

Structural basis of respiratory complexes adaptation to cold temperatures
Pamela Ornelas

Actin polymerization drives lumen formation in a human epiblast model
Megane Rayer, Rivka Shapiro

Also in the cancer biology category:
Mitochondria-derived nuclear ATP surge protects against confinement-induced proliferation defects
Teodora Piskova

Spatial transcriptomics elucidates medulla niche supporting germinal center response in myasthenia gravis thymoma
Jessica Chevallier

Invasion of glioma cells through confined space requires membrane tension regulation and mechano-electrical coupling via Plexin-B2
Jade Chan

Also in the cell biology category:
Cell cycle-dependent mRNA localization in P-bodies
Mohammed JALLOH

Control of Inflammatory Response by Tissue Microenvironment
Roberto Amadio

Notch3 is a genetic modifier of NODAL signalling for patterning asymmetry during mouse heart looping
Bhaval Parmar

Also in the developmental biology category:
Gestational exposure to high heat-humidity conditions impairs mouse embryonic development
Girish Kale, preLights peer support

Modular control of time and space during vertebrate axis segmentation
AND
Natural genetic variation quantitatively regulates heart rate and dimension
Girish Kale, Jennifer Ann Black

Lipid-Based Transfection of Zebrafish Embryos: A Robust Protocol for Nucleic Acid Delivery
Roberto Rodríguez-Morales

Also in the microbiology category:
Characterization of natural product inhibitors of quorum sensing in Pseudomonas aeruginosa reveals competitive inhibition of RhlR by ortho-vanillin
UofA IMB565 et al.
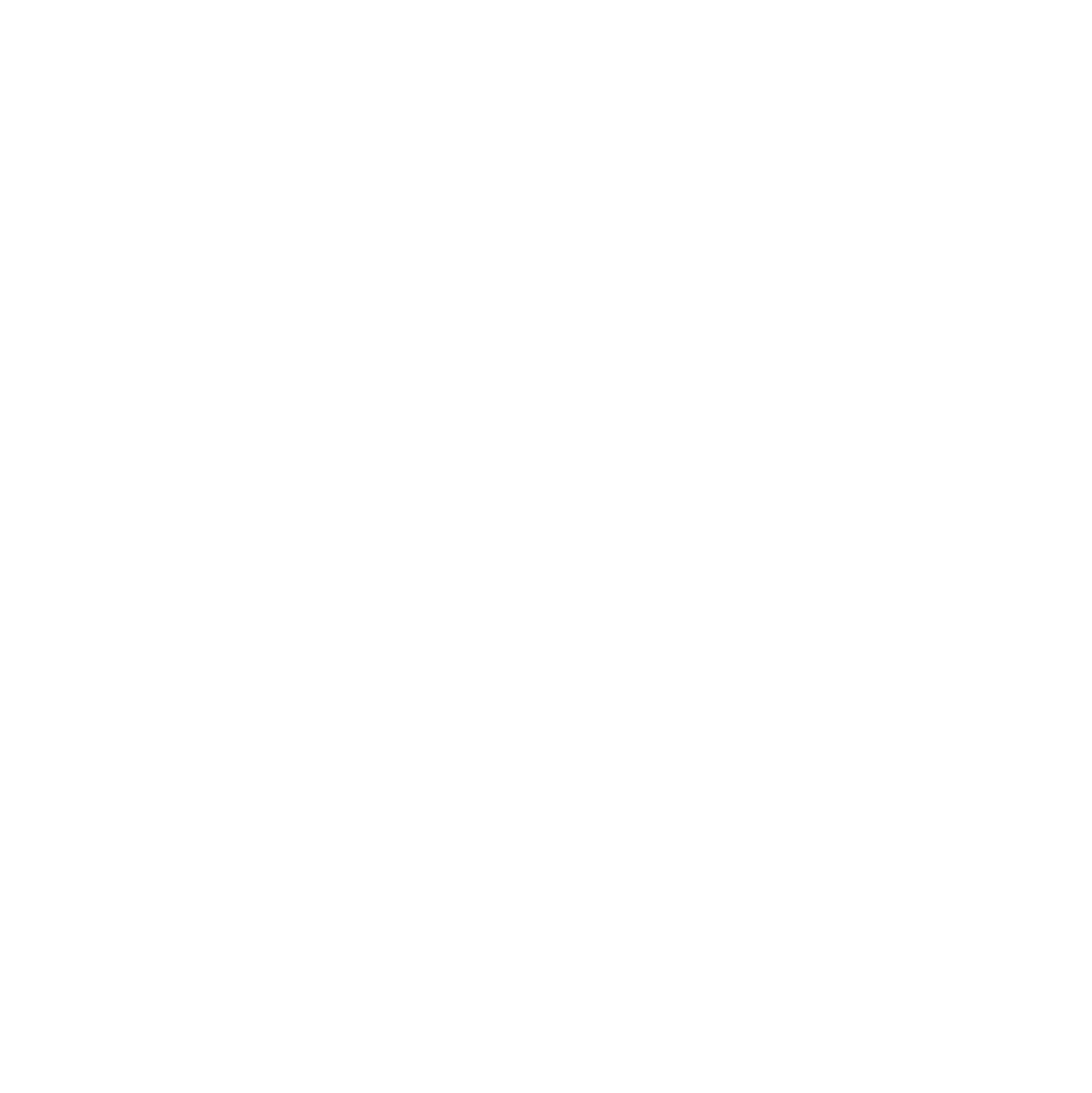
Feedback loop regulation between viperin and viral hemorrhagic septicemia virus through competing protein degradation pathways
UofA IMB565 et al.

Lytic bacteriophages interact with respiratory epithelial cells and induce the secretion of antiviral and proinflammatory cytokines
UofA IMB565 et al.
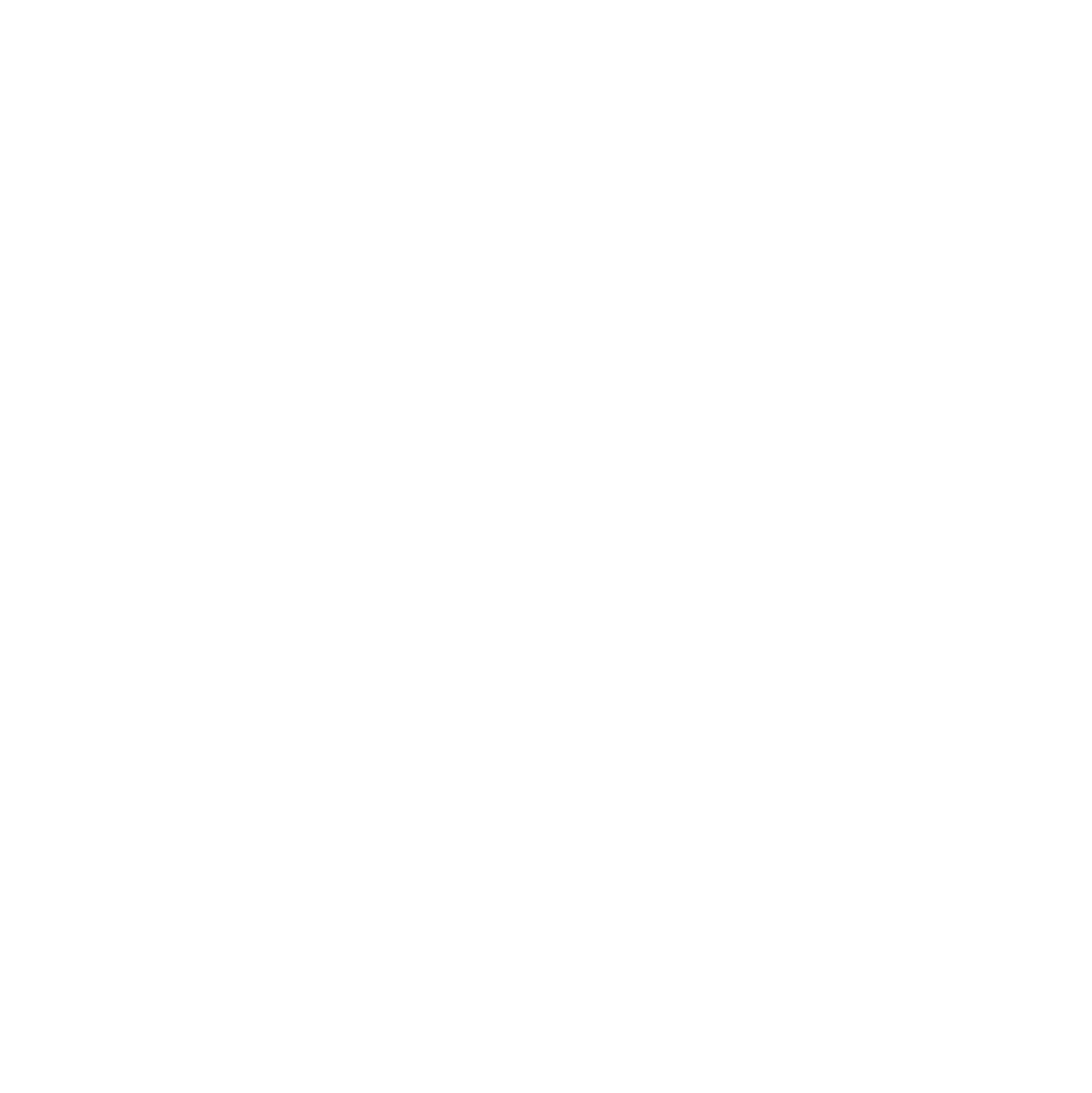
preListsbiochemistry category:
in theBSCB-Biochemical Society 2024 Cell Migration meeting
This preList features preprints that were discussed and presented during the BSCB-Biochemical Society 2024 Cell Migration meeting in Birmingham, UK in April 2024. Kindly put together by Sara Morais da Silva, Reviews Editor at Journal of Cell Science.
List by | Reinier Prosee |
Peer Review in Biomedical Sciences
Communication of scientific knowledge has changed dramatically in recent decades and the public perception of scientific discoveries depends on the peer review process of articles published in scientific journals. Preprints are key vehicles for the dissemination of scientific discoveries, but they are still not properly recognized by the scientific community since peer review is very limited. On the other hand, peer review is very heterogeneous and a fundamental aspect to improve it is to train young scientists on how to think critically and how to evaluate scientific knowledge in a professional way. Thus, this course aims to: i) train students on how to perform peer review of scientific manuscripts in a professional manner; ii) develop students' critical thinking; iii) contribute to the appreciation of preprints as important vehicles for the dissemination of scientific knowledge without restrictions; iv) contribute to the development of students' curricula, as their opinions will be published and indexed on the preLights platform. The evaluations will be based on qualitative analyses of the oral presentations of preprints in the field of biomedical sciences deposited in the bioRxiv server, of the critical reports written by the students, as well as of the participation of the students during the preprints discussions.
List by | Marcus Oliveira et al. |
CellBio 2022 – An ASCB/EMBO Meeting
This preLists features preprints that were discussed and presented during the CellBio 2022 meeting in Washington, DC in December 2022.
List by | Nadja Hümpfer et al. |
20th “Genetics Workshops in Hungary”, Szeged (25th, September)
In this annual conference, Hungarian geneticists, biochemists and biotechnologists presented their works. Link: http://group.szbk.u-szeged.hu/minikonf/archive/prg2021.pdf
List by | Nándor Lipták |
Fibroblasts
The advances in fibroblast biology preList explores the recent discoveries and preprints of the fibroblast world. Get ready to immerse yourself with this list created for fibroblasts aficionados and lovers, and beyond. Here, my goal is to include preprints of fibroblast biology, heterogeneity, fate, extracellular matrix, behavior, topography, single-cell atlases, spatial transcriptomics, and their matrix!
List by | Osvaldo Contreras |
ASCB EMBO Annual Meeting 2019
A collection of preprints presented at the 2019 ASCB EMBO Meeting in Washington, DC (December 7-11)
List by | Madhuja Samaddar et al. |
EMBL Seeing is Believing – Imaging the Molecular Processes of Life
Preprints discussed at the 2019 edition of Seeing is Believing, at EMBL Heidelberg from the 9th-12th October 2019
List by | Dey Lab |
Cellular metabolism
A curated list of preprints related to cellular metabolism at Biorxiv by Pablo Ranea Robles from the Prelights community. Special interest on lipid metabolism, peroxisomes and mitochondria.
List by | Pablo Ranea Robles |
MitoList
This list of preprints is focused on work expanding our knowledge on mitochondria in any organism, tissue or cell type, from the normal biology to the pathology.
List by | Sandra Franco Iborra |
Also in the bioinformatics category:
‘In preprints’ from Development 2022-2023
A list of the preprints featured in Development's 'In preprints' articles between 2022-2023
List by | Alex Eve, Katherine Brown |
9th International Symposium on the Biology of Vertebrate Sex Determination
This preList contains preprints discussed during the 9th International Symposium on the Biology of Vertebrate Sex Determination. This conference was held in Kona, Hawaii from April 17th to 21st 2023.
List by | Martin Estermann |
Alumni picks – preLights 5th Birthday
This preList contains preprints that were picked and highlighted by preLights Alumni - an initiative that was set up to mark preLights 5th birthday. More entries will follow throughout February and March 2023.
List by | Sergio Menchero et al. |
Fibroblasts
The advances in fibroblast biology preList explores the recent discoveries and preprints of the fibroblast world. Get ready to immerse yourself with this list created for fibroblasts aficionados and lovers, and beyond. Here, my goal is to include preprints of fibroblast biology, heterogeneity, fate, extracellular matrix, behavior, topography, single-cell atlases, spatial transcriptomics, and their matrix!
List by | Osvaldo Contreras |
Single Cell Biology 2020
A list of preprints mentioned at the Wellcome Genome Campus Single Cell Biology 2020 meeting.
List by | Alex Eve |
Antimicrobials: Discovery, clinical use, and development of resistance
Preprints that describe the discovery of new antimicrobials and any improvements made regarding their clinical use. Includes preprints that detail the factors affecting antimicrobial selection and the development of antimicrobial resistance.
List by | Zhang-He Goh |
Also in the biophysics category:
preLights peer support – preprints of interest
This is a preprint repository to organise the preprints and preLights covered through the 'preLights peer support' initiative.
List by | preLights peer support |
66th Biophysical Society Annual Meeting, 2022
Preprints presented at the 66th BPS Annual Meeting, Feb 19 - 23, 2022 (The below list is not exhaustive and the preprints are listed in no particular order.)
List by | Soni Mohapatra |
EMBL Synthetic Morphogenesis: From Gene Circuits to Tissue Architecture (2021)
A list of preprints mentioned at the #EESmorphoG virtual meeting in 2021.
List by | Alex Eve |
Biophysical Society Meeting 2020
Some preprints presented at the Biophysical Society Meeting 2020 in San Diego, USA.
List by | Tessa Sinnige |
ASCB EMBO Annual Meeting 2019
A collection of preprints presented at the 2019 ASCB EMBO Meeting in Washington, DC (December 7-11)
List by | Madhuja Samaddar et al. |
EMBL Seeing is Believing – Imaging the Molecular Processes of Life
Preprints discussed at the 2019 edition of Seeing is Believing, at EMBL Heidelberg from the 9th-12th October 2019
List by | Dey Lab |
Biomolecular NMR
Preprints related to the application and development of biomolecular NMR spectroscopy
List by | Reid Alderson |
Biophysical Society Annual Meeting 2019
Few of the preprints that were discussed in the recent BPS annual meeting at Baltimore, USA
List by | Joseph Jose Thottacherry |
Also in the cancer biology category:
BSCB-Biochemical Society 2024 Cell Migration meeting
This preList features preprints that were discussed and presented during the BSCB-Biochemical Society 2024 Cell Migration meeting in Birmingham, UK in April 2024. Kindly put together by Sara Morais da Silva, Reviews Editor at Journal of Cell Science.
List by | Reinier Prosee |
CSHL 87th Symposium: Stem Cells
Preprints mentioned by speakers at the #CSHLsymp23
List by | Alex Eve |
Journal of Cell Science meeting ‘Imaging Cell Dynamics’
This preList highlights the preprints discussed at the JCS meeting 'Imaging Cell Dynamics'. The meeting was held from 14 - 17 May 2023 in Lisbon, Portugal and was organised by Erika Holzbaur, Jennifer Lippincott-Schwartz, Rob Parton and Michael Way.
List by | Helen Zenner |
CellBio 2022 – An ASCB/EMBO Meeting
This preLists features preprints that were discussed and presented during the CellBio 2022 meeting in Washington, DC in December 2022.
List by | Nadja Hümpfer et al. |
Fibroblasts
The advances in fibroblast biology preList explores the recent discoveries and preprints of the fibroblast world. Get ready to immerse yourself with this list created for fibroblasts aficionados and lovers, and beyond. Here, my goal is to include preprints of fibroblast biology, heterogeneity, fate, extracellular matrix, behavior, topography, single-cell atlases, spatial transcriptomics, and their matrix!
List by | Osvaldo Contreras |
Single Cell Biology 2020
A list of preprints mentioned at the Wellcome Genome Campus Single Cell Biology 2020 meeting.
List by | Alex Eve |
ASCB EMBO Annual Meeting 2019
A collection of preprints presented at the 2019 ASCB EMBO Meeting in Washington, DC (December 7-11)
List by | Madhuja Samaddar et al. |
Lung Disease and Regeneration
This preprint list compiles highlights from the field of lung biology.
List by | Rob Hynds |
Anticancer agents: Discovery and clinical use
Preprints that describe the discovery of anticancer agents and their clinical use. Includes both small molecules and macromolecules like biologics.
List by | Zhang-He Goh |
Also in the cell biology category:
BSCB-Biochemical Society 2024 Cell Migration meeting
This preList features preprints that were discussed and presented during the BSCB-Biochemical Society 2024 Cell Migration meeting in Birmingham, UK in April 2024. Kindly put together by Sara Morais da Silva, Reviews Editor at Journal of Cell Science.
List by | Reinier Prosee |
‘In preprints’ from Development 2022-2023
A list of the preprints featured in Development's 'In preprints' articles between 2022-2023
List by | Alex Eve, Katherine Brown |
preLights peer support – preprints of interest
This is a preprint repository to organise the preprints and preLights covered through the 'preLights peer support' initiative.
List by | preLights peer support |
The Society for Developmental Biology 82nd Annual Meeting
This preList is made up of the preprints discussed during the Society for Developmental Biology 82nd Annual Meeting that took place in Chicago in July 2023.
List by | Joyce Yu, Katherine Brown |
CSHL 87th Symposium: Stem Cells
Preprints mentioned by speakers at the #CSHLsymp23
List by | Alex Eve |
Journal of Cell Science meeting ‘Imaging Cell Dynamics’
This preList highlights the preprints discussed at the JCS meeting 'Imaging Cell Dynamics'. The meeting was held from 14 - 17 May 2023 in Lisbon, Portugal and was organised by Erika Holzbaur, Jennifer Lippincott-Schwartz, Rob Parton and Michael Way.
List by | Helen Zenner |
9th International Symposium on the Biology of Vertebrate Sex Determination
This preList contains preprints discussed during the 9th International Symposium on the Biology of Vertebrate Sex Determination. This conference was held in Kona, Hawaii from April 17th to 21st 2023.
List by | Martin Estermann |
Alumni picks – preLights 5th Birthday
This preList contains preprints that were picked and highlighted by preLights Alumni - an initiative that was set up to mark preLights 5th birthday. More entries will follow throughout February and March 2023.
List by | Sergio Menchero et al. |
CellBio 2022 – An ASCB/EMBO Meeting
This preLists features preprints that were discussed and presented during the CellBio 2022 meeting in Washington, DC in December 2022.
List by | Nadja Hümpfer et al. |
Fibroblasts
The advances in fibroblast biology preList explores the recent discoveries and preprints of the fibroblast world. Get ready to immerse yourself with this list created for fibroblasts aficionados and lovers, and beyond. Here, my goal is to include preprints of fibroblast biology, heterogeneity, fate, extracellular matrix, behavior, topography, single-cell atlases, spatial transcriptomics, and their matrix!
List by | Osvaldo Contreras |
EMBL Synthetic Morphogenesis: From Gene Circuits to Tissue Architecture (2021)
A list of preprints mentioned at the #EESmorphoG virtual meeting in 2021.
List by | Alex Eve |
FENS 2020
A collection of preprints presented during the virtual meeting of the Federation of European Neuroscience Societies (FENS) in 2020
List by | Ana Dorrego-Rivas |
Planar Cell Polarity – PCP
This preList contains preprints about the latest findings on Planar Cell Polarity (PCP) in various model organisms at the molecular, cellular and tissue levels.
List by | Ana Dorrego-Rivas |
BioMalPar XVI: Biology and Pathology of the Malaria Parasite
[under construction] Preprints presented at the (fully virtual) EMBL BioMalPar XVI, 17-18 May 2020 #emblmalaria
List by | Dey Lab, Samantha Seah |
1
Cell Polarity
Recent research from the field of cell polarity is summarized in this list of preprints. It comprises of studies focusing on various forms of cell polarity ranging from epithelial polarity, planar cell polarity to front-to-rear polarity.
List by | Yamini Ravichandran |
TAGC 2020
Preprints recently presented at the virtual Allied Genetics Conference, April 22-26, 2020. #TAGC20
List by | Maiko Kitaoka et al. |
3D Gastruloids
A curated list of preprints related to Gastruloids (in vitro models of early development obtained by 3D aggregation of embryonic cells). Updated until July 2021.
List by | Paul Gerald L. Sanchez and Stefano Vianello |
ECFG15 – Fungal biology
Preprints presented at 15th European Conference on Fungal Genetics 17-20 February 2020 Rome
List by | Hiral Shah |
ASCB EMBO Annual Meeting 2019
A collection of preprints presented at the 2019 ASCB EMBO Meeting in Washington, DC (December 7-11)
List by | Madhuja Samaddar et al. |
EMBL Seeing is Believing – Imaging the Molecular Processes of Life
Preprints discussed at the 2019 edition of Seeing is Believing, at EMBL Heidelberg from the 9th-12th October 2019
List by | Dey Lab |
Autophagy
Preprints on autophagy and lysosomal degradation and its role in neurodegeneration and disease. Includes molecular mechanisms, upstream signalling and regulation as well as studies on pharmaceutical interventions to upregulate the process.
List by | Sandra Malmgren Hill |
Lung Disease and Regeneration
This preprint list compiles highlights from the field of lung biology.
List by | Rob Hynds |
Cellular metabolism
A curated list of preprints related to cellular metabolism at Biorxiv by Pablo Ranea Robles from the Prelights community. Special interest on lipid metabolism, peroxisomes and mitochondria.
List by | Pablo Ranea Robles |
BSCB/BSDB Annual Meeting 2019
Preprints presented at the BSCB/BSDB Annual Meeting 2019
List by | Dey Lab |
MitoList
This list of preprints is focused on work expanding our knowledge on mitochondria in any organism, tissue or cell type, from the normal biology to the pathology.
List by | Sandra Franco Iborra |
Biophysical Society Annual Meeting 2019
Few of the preprints that were discussed in the recent BPS annual meeting at Baltimore, USA
List by | Joseph Jose Thottacherry |
ASCB/EMBO Annual Meeting 2018
This list relates to preprints that were discussed at the recent ASCB conference.
List by | Dey Lab, Amanda Haage |
Also in the developmental biology category:
BSDB/GenSoc Spring Meeting 2024
A list of preprints highlighted at the British Society for Developmental Biology and Genetics Society joint Spring meeting 2024 at Warwick, UK.
List by | Joyce Yu, Katherine Brown |
GfE/ DSDB meeting 2024
This preList highlights the preprints discussed at the 2024 joint German and Dutch developmental biology societies meeting that took place in March 2024 in Osnabrück, Germany.
List by | Joyce Yu |
‘In preprints’ from Development 2022-2023
A list of the preprints featured in Development's 'In preprints' articles between 2022-2023
List by | Alex Eve, Katherine Brown |
preLights peer support – preprints of interest
This is a preprint repository to organise the preprints and preLights covered through the 'preLights peer support' initiative.
List by | preLights peer support |
The Society for Developmental Biology 82nd Annual Meeting
This preList is made up of the preprints discussed during the Society for Developmental Biology 82nd Annual Meeting that took place in Chicago in July 2023.
List by | Joyce Yu, Katherine Brown |
CSHL 87th Symposium: Stem Cells
Preprints mentioned by speakers at the #CSHLsymp23
List by | Alex Eve |
Journal of Cell Science meeting ‘Imaging Cell Dynamics’
This preList highlights the preprints discussed at the JCS meeting 'Imaging Cell Dynamics'. The meeting was held from 14 - 17 May 2023 in Lisbon, Portugal and was organised by Erika Holzbaur, Jennifer Lippincott-Schwartz, Rob Parton and Michael Way.
List by | Helen Zenner |
9th International Symposium on the Biology of Vertebrate Sex Determination
This preList contains preprints discussed during the 9th International Symposium on the Biology of Vertebrate Sex Determination. This conference was held in Kona, Hawaii from April 17th to 21st 2023.
List by | Martin Estermann |
Alumni picks – preLights 5th Birthday
This preList contains preprints that were picked and highlighted by preLights Alumni - an initiative that was set up to mark preLights 5th birthday. More entries will follow throughout February and March 2023.
List by | Sergio Menchero et al. |
CellBio 2022 – An ASCB/EMBO Meeting
This preLists features preprints that were discussed and presented during the CellBio 2022 meeting in Washington, DC in December 2022.
List by | Nadja Hümpfer et al. |
2nd Conference of the Visegrád Group Society for Developmental Biology
Preprints from the 2nd Conference of the Visegrád Group Society for Developmental Biology (2-5 September, 2021, Szeged, Hungary)
List by | Nándor Lipták |
Fibroblasts
The advances in fibroblast biology preList explores the recent discoveries and preprints of the fibroblast world. Get ready to immerse yourself with this list created for fibroblasts aficionados and lovers, and beyond. Here, my goal is to include preprints of fibroblast biology, heterogeneity, fate, extracellular matrix, behavior, topography, single-cell atlases, spatial transcriptomics, and their matrix!
List by | Osvaldo Contreras |
EMBL Synthetic Morphogenesis: From Gene Circuits to Tissue Architecture (2021)
A list of preprints mentioned at the #EESmorphoG virtual meeting in 2021.
List by | Alex Eve |
EMBL Conference: From functional genomics to systems biology
Preprints presented at the virtual EMBL conference "from functional genomics and systems biology", 16-19 November 2020
List by | Jesus Victorino |
Single Cell Biology 2020
A list of preprints mentioned at the Wellcome Genome Campus Single Cell Biology 2020 meeting.
List by | Alex Eve |
Society for Developmental Biology 79th Annual Meeting
Preprints at SDB 2020
List by | Irepan Salvador-Martinez, Martin Estermann |
FENS 2020
A collection of preprints presented during the virtual meeting of the Federation of European Neuroscience Societies (FENS) in 2020
List by | Ana Dorrego-Rivas |
Planar Cell Polarity – PCP
This preList contains preprints about the latest findings on Planar Cell Polarity (PCP) in various model organisms at the molecular, cellular and tissue levels.
List by | Ana Dorrego-Rivas |
Cell Polarity
Recent research from the field of cell polarity is summarized in this list of preprints. It comprises of studies focusing on various forms of cell polarity ranging from epithelial polarity, planar cell polarity to front-to-rear polarity.
List by | Yamini Ravichandran |
TAGC 2020
Preprints recently presented at the virtual Allied Genetics Conference, April 22-26, 2020. #TAGC20
List by | Maiko Kitaoka et al. |
3D Gastruloids
A curated list of preprints related to Gastruloids (in vitro models of early development obtained by 3D aggregation of embryonic cells). Updated until July 2021.
List by | Paul Gerald L. Sanchez and Stefano Vianello |
ASCB EMBO Annual Meeting 2019
A collection of preprints presented at the 2019 ASCB EMBO Meeting in Washington, DC (December 7-11)
List by | Madhuja Samaddar et al. |
EDBC Alicante 2019
Preprints presented at the European Developmental Biology Congress (EDBC) in Alicante, October 23-26 2019.
List by | Sergio Menchero et al. |
EMBL Seeing is Believing – Imaging the Molecular Processes of Life
Preprints discussed at the 2019 edition of Seeing is Believing, at EMBL Heidelberg from the 9th-12th October 2019
List by | Dey Lab |
SDB 78th Annual Meeting 2019
A curation of the preprints presented at the SDB meeting in Boston, July 26-30 2019. The preList will be updated throughout the duration of the meeting.
List by | Alex Eve |
Lung Disease and Regeneration
This preprint list compiles highlights from the field of lung biology.
List by | Rob Hynds |
Young Embryologist Network Conference 2019
Preprints presented at the Young Embryologist Network 2019 conference, 13 May, The Francis Crick Institute, London
List by | Alex Eve |
Pattern formation during development
The aim of this preList is to integrate results about the mechanisms that govern patterning during development, from genes implicated in the processes to theoritical models of pattern formation in nature.
List by | Alexa Sadier |
BSCB/BSDB Annual Meeting 2019
Preprints presented at the BSCB/BSDB Annual Meeting 2019
List by | Dey Lab |
Zebrafish immunology
A compilation of cutting-edge research that uses the zebrafish as a model system to elucidate novel immunological mechanisms in health and disease.
List by | Shikha Nayar |
Also in the microbiology category:
BioMalPar XVI: Biology and Pathology of the Malaria Parasite
[under construction] Preprints presented at the (fully virtual) EMBL BioMalPar XVI, 17-18 May 2020 #emblmalaria
List by | Dey Lab, Samantha Seah |
1
ECFG15 – Fungal biology
Preprints presented at 15th European Conference on Fungal Genetics 17-20 February 2020 Rome
List by | Hiral Shah |
EMBL Seeing is Believing – Imaging the Molecular Processes of Life
Preprints discussed at the 2019 edition of Seeing is Believing, at EMBL Heidelberg from the 9th-12th October 2019
List by | Dey Lab |
Antimicrobials: Discovery, clinical use, and development of resistance
Preprints that describe the discovery of new antimicrobials and any improvements made regarding their clinical use. Includes preprints that detail the factors affecting antimicrobial selection and the development of antimicrobial resistance.
List by | Zhang-He Goh |